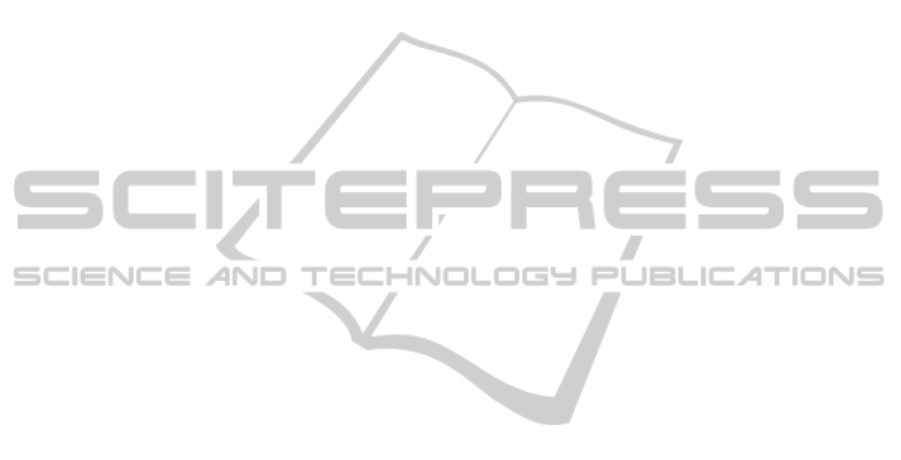
required equipment. The video images used here are
standard resolution images captured by a normal
camera. The processing only requires a computer.
This combination is less expensive than some of the
other devices used to monitor the respiration rate
from a distance. This simple equipment is also easy
to use and could be used in a home environment as
well. Home monitoring is more comfortable for the
patient and its parents, but it is also less expensive
and allows the hospital to take care of another
patient instead of the one being monitored at home.
In conclusion, this setup is a first step improving the
neonatal assessment regarding the vital sign of
respiration.
ACKNOWLEDGEMENTS
Research supported by:
Research Council KUL: GOA/10/09 MaNet, CoE
PFV/10/002 (OPTEC); PhD/Postdoc grants;
Flemish Government: IWT: projects: TBM 110697-
NeoGuard; PhD/Postdoc grants;
Belgian Federal Science Policy Office: IUAP P7/19/
(DYSCO)
EU: ERC Advanced Grant: BIOTENSORS (n°
339804).
REFERENCES
Aarts, L. A., Jeanne, V., Cleary, J. P., Lieber, C., Nelson,
J.S., Bambang Oetomo, S., Verkruysse, W., 2013.
Non-contact heart rate monitoring utilizing camera
photoplethysmography in the neonatal intensive care
unit - a pilot study. In Early Human Development,
89(12): p. 943-948.
Abbas, A. and Heiman, K., 2009. Non-contact respiratory.
monitoring based on real-time IR-thermography. In
IFMBE Proceedings, 25(4): p. 1306–1309.
Al-Khalidi, F.Q., Saatchi, R., Burke, D., Elphick, H., and.
Tan, S., 2011. Respiration rate monitoring methods: a
review. In Pediatric pulmonology, 46(6): p. 523–529.
Choi, J., Jeon,W. J., and Lee, S-C., 2008. Spatio-temporal
pyramid matching for sports videos. In Proceeding of
the first ACM international conference on Multimedia
information retrieval. New York, USA: p. 291–297.
Fleet, D. and Weiss, Y., 2006. Optical flow estimation. In
Handbook of Mathematical Models in Computer
Vision: p. 239–258.
Folke, M., Cernerud, L., Ekström, M., and Hök, B., 2003.
Critical review of non-invasive respiratory monitoring
in medical care. In Medical & biological engineering
& computing, 41(4): p. 377–383.
Horn, B. K. and Schunck, B. G., 1981. Determining
optical flow. In Artificial Intelligence, 17(1-3): p. 185–
203.
Kwon, S., Kim, H., and Park, K. S., 2012. Validation of
heart rate extraction using video imaging on a builtin
camera system of a smartphone. In Proceedings of the
Annual International Conference of the IEEE
Engineering in Medicine and Biology Society, EMBS.
San Diego, USA: p. 2174–2177.
Li, C., Lubecke, V., Boric-Lubecke, O., and Lin, J., 2013.
A review on recent advances in Doppler radar sensors
for noncontact healthcare monitoring. In IEEE
Transactions on microwave theory and techniques,
61(5): p. 2046–2060.
Miller, H. and Behrle, F., 1953. Changing patterns of
respiration in newborn infants. In Pediatrics, 12(2): p.
141–150.
Nakjima, K., 2001. Development of real-time image
sequence analysis for evaluating posture change and
respiratory rate of a subject in bed. In Physiological
Measurement, 22(3): p. 21–28.
O’Donovan, P., 2005. Optical Flow: Techniques and
Applications. In International Journal of Computer
Vision, p. 1–26.
Penne, J., Schaller, C., Hornegger, J., and Kuwert, T.,
2008. Robust real-time 3D respiratory motion
detection using time-of-flight cameras. In
International Journal of Computer Assisted Radiology
and Surgery, 3(5): p. 427–431.
Sun, D., Roth, S., Lewis, J., and Black, M., 2008.
Learning optical flow. In Computer Vision ECCV,
Lecture Notes in Computer Science, 5304: p. 83-97.
Tan, K., Saatchi, R., Elphick, H., and Burke, D., 2010.
Real-time vision based respiration monitoring system.
In Proceeding of the seventh IEEE IET International
Symposium on Communication Systems, Networks and
Digital Signal Processing. Newcastle, UK: p. 770–
774.
Wu, H.-y., Rubinstein, M., Shih, E., and Freeman, W.,
2012. Eulerian Video Magnification for Revealing
Subtle Changes in theWorld. In Proceedings of ACM
Transactions on Graphics, 31: p. 1–8.
Automated Respiration Detection from Neonatal Video Data
169