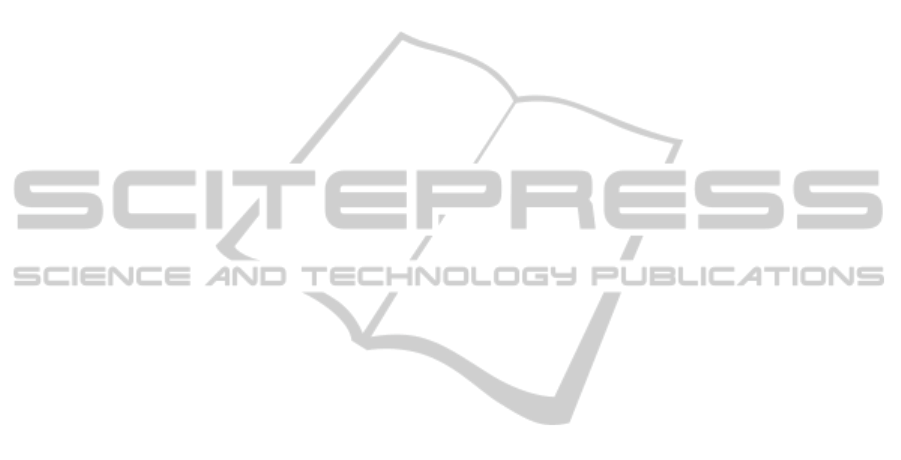
fails its value is really low or even zero.
In spite of these problems, the obtained results are
valuable because they demonstrate that the election
of the dissimilarity measure along with the clustering
algorithm is important for the classification perfor-
mance. This fact should be taken into account in an-
other clustering applications of FTIR data, where only
the Euclidean distance is commonly utilised (Sec. 1).
5 CONCLUSIONS
A methodology for studying the ability of five dissim-
ilarity coefficients to correctly separate hyperspectral
data was carried out. For this purpose three different
clustering algorithms were used to gather eighty five
spectra in their corresponding types of cell. These
spectra belonged to two different groups due to the
two different protocols used in the acquisition step.
As a novelty, a new spectral representation model
has been described. This method extracts the main
features enclosed in the principal peaks of the spec-
trum and translates them into a signal that can be more
robust against scattering and sensor’s artefacts.
As main conclusion of this study, not only the op-
timal dissimilarity measure is data dependent, but also
the optimal clustering algorithm. It is necessary to ex-
tend this study to new spectral data to be able to gener-
alise the results. Nevertheless, the Spectral Informa-
tion Divergence has obtained the best overall results
in the classification task when is applied over the pro-
posed Gaussian model in both treatment protocols.
The future steps will be the comparison of other
dissimilarity coefficients and more complex cluster-
ing algorithms in new FTIR datasets containing more
samples. As inputs of the algorithms, new ways to
represent the main information of spectra (PCA and
Sparse Representation) will be studied and compared
with the proposed Gaussian model, which will be im-
proved to contain other significant signal properties.
ACKNOWLEDGEMENTS
This research has been supported by the European
Commission through the Framework Seven project
MINERVA (317803; www.minerva-project.eu).
REFERENCES
Baker, M., Gazi, E., Brown, M., Shanks, J., Gardner, P., and
Clarke, N. (2008). FTIR-based spectroscopic analysis
in the identification of clinically aggressive prostate
cancer. Br J Cancer, 99(11):1859–66.
Bandyopadhyay, S. and Saha, S. (2013). Unsuper-
vised Classification: Similarity Measures, Classi-
cal and Metaheuristic Approaches, and Applications.
Springer.
Berkhin, P. (2006). A survey of clustering data mining tech-
niques. In Grouping multidimensional data, pages 25–
71. Springer.
Carter, H. B., Albertsen, P., Barry, M., Etzioni, R., Freed-
land, S., Greene, K., Holmberg, L., Kantoff, P.,
Konety, B., Murad, M., Penson, D., and Zietman,
A. (2013). Early detection of prostate cancer: AUA
guideline. The Journal of Urology, 190(2):419 – 26.
Chang, C.-I. (2003). Hyperspectral imaging: techniques
for spectral detection and classification, volume 1.
Springer.
Jain, A. K. (2010). Data clustering: 50 years beyond k-
means. Pattern Recognition Letters, 31(8):651–666.
Kendall, C., Isabelle, M., Bazant-Hegemark, F., Hutchings,
J., Orr, L., Babrah, J., Baker, R., and Stone, N. (2009).
Vibrational spectroscopy: a clinical tool for cancer di-
agnostics. Analyst, 134(6):1029–1045.
Krafft, C., Codrich, D., Pelizzo, G., and Sergo, V. (2008).
Raman and ftir microscopic imaging of colon tissue: a
comparative study. Journal of biophotonics, 1(2):154–
169.
Lasch, P. (2012). Spectral pre-processing for biomedical vi-
brational spectroscopy and microspectroscopic imag-
ing. Chemometrics and Intelligent Laboratory Sys-
tems, 117:100–114.
Lasch, P., Haensch, W., Naumann, D., and Diem, M.
(2004). Imaging of colorectal adenocarcinoma using
ft-ir microspectroscopy and cluster analysis. Biochim-
ica et Biophysica Acta (BBA)-Molecular Basis of Dis-
ease, 1688(2):176–186.
Nallala, J., Piot, O., Diebold, M.-D., Gobinet, C., Bouch´e,
O., Manfait, M., and Sockalingum, G. D. (2014).
Infrared and raman imaging for characterizing com-
plex biological materials: A comparative morpho-
spectroscopic study of colon tissue. Applied spec-
troscopy, 68(1):57–68.
Petibois, C. and Deleris, G. (2006). Chemical mapping
of tumor progression by FT-IR imaging: towards
molecular histopathology. Trends in Biotechnology,
24(10):455 – 62.
Rinnan, A., Berg, F. v. d., and Engelsen, S. B. (2009). Re-
view of the most common pre-processing techniques
for near-infrared spectra. TrAC Trends in Analytical
Chemistry, 28(10):1201–1222.
Soille, P. (2002). Morphological Image Analysis, volume
Second edition. Springer.
NewSpectralRepresentationandDissimilarityMeasuresAssessmentforFTIR-spectrausingUnsupervisedClassification
177