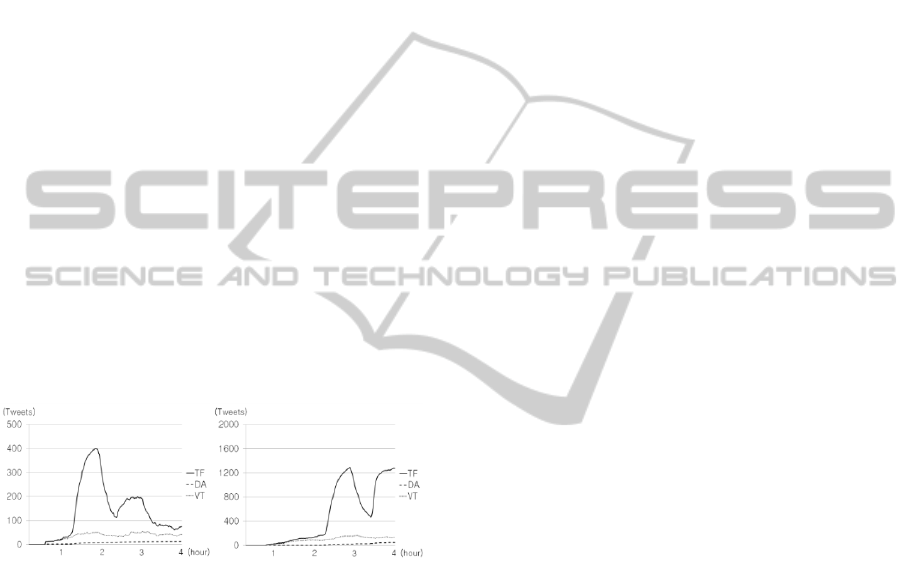
EventDecision(k) must be bigger than 0 to be
determined as an event location.
(1)
(2)
4 RESULT
We collected the social data on Twitter from March
2013 to April 2014, more than a year. The control
event set was from the list of Breaking News from
Korean Broadcasting System, and the experimental
event set was extracted from the proposed system.
At the time when we were conducting the evaluation,
the system could collect about 60,000 Korean
Tweets in an hour. It took 22 minutes in average to
initialize the system. The initializing time includes
the time to scan all the data with two days history
and to create a set of clusters. Once the system is on
track, it takes only 0.277 seconds in average to scan
all the locations. This result shows that our system
can analyze the social data in real time within a
second.
Figure 4: Changes of TF, DA, and VT values.
Figure 4-left shows the changes of TF, DA, and VT
values at 3:50pm on March 9, 2013 in Pohang,
Korea. At the time, there was a fire at a mountain.
Our system detected the event 26 minutes after the
fire occurs. It only took less than half an hour
because there were not many Tweets in this city and
many people could observe the incident.
Figure 4-right is the graph that shows another
incident at 9:16pm on February 17, 2014 in
Gyeongju, Korea. When the roof of a resort was
collapsed, more than a thousand university students
were staying at the resort. The interesting point on
this graph is the aspect of information propagation.
Right after the incident, the graph reached the first
peak by the people who were at the scene. VT value
explains this aspect. The number of Tweets had been
reduced for a while, and the graph hit the second
peak later. During the graph hits the second peak,
the Tweets of the first peak started to be retweeted
by their followers and the Tweets from the news media
pushed up the graph as well.
5 CONCLUSIONS
This paper introduces the Real-time Event-location
Analyzer and shows its performance. The proposed
system detects an event in real-time if the event
occurs in a town or at a resort, but it still need to be
improved in its precision of the result and need to
reduce the false event. We plan to explore the up-to-
date methods in natural language processing that can
be applied to our system.
ACKNOWLEDGEMENTS
This research was supported by Basic Science
Research Program through the National Research
Foundation of Korea (NRF) funded by the Ministry
of Education, Science and Technology (No. 2011-
0009407).
REFERENCES
Barbosa, L., Feng. J., 2010. Robust Sentiment Detection
on Twitter from Biased and Noisy Data. In Proc. of
23
rd
Int'l Conf. on Computational Linguistics. pp. 36-44.
Lee, B., 2012. A Temporal Analysis of Posting Behavior
in Social Media Streams. In Proc. of ICWSM'12
Workshop on Social Media Visualization.
Hong, C., Kim, H., 2011. Effective Feature Extraction for
Tweets Classification. In Proc. of Korea Computer
Congress. pp. 229-232.
Lee, B., Hwang, B. Y., 2012. A Study of the Correlation
between the Spatial Attributes on Twitter. In Proc. of
ICDE'12 Workshop on Spatio-Temporal Data
Integration and Retrieval.
Lee, B., Yoon, J., Kim, S., and Hwang, B. Y., 2012.
Detecting Social Signals of Flu Symptoms. In Proc. of
COLLABORATECOM'12. pp. 544-545.
Lee, J., Bengio, S., Kim, S., Lebanon, G., and Singer, Y.,
2014. Local Collaborative Ranking. In Proc of 23rd
Int'l Conf. on WWW Conference Committee. pp 85-95.
Sakaki, T., Okzaki, M., and Matsuo, Y., 2010. Earthquake
Shakes Twitter Users: Real-time Event Detection by
Social Sensors. In Proc. of the 19th International
Conference on WWW. pp. 851-860.
Li, R., Lei, K. H., Khadiwala, R., and Chang, K. C. C.,
2012. TEDAS: a Twitter based Event Detection and
Analysis System. In Proc of the 28th International
Conference on Data Engineering. pp. 1273-1276.
ICAART2015-InternationalConferenceonAgentsandArtificialIntelligence
352