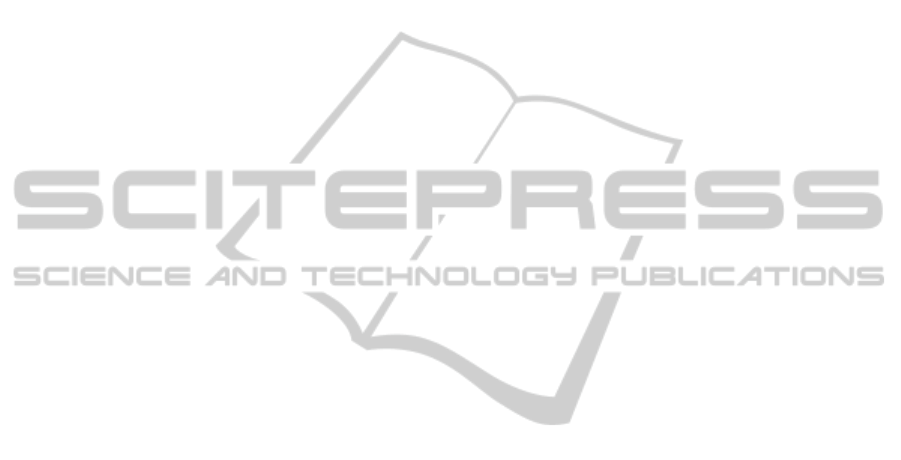
partial or occluded data is available for recognition.
Scenarios, like the one described in the last section,
where faces are purposely occluded may be an inter-
esting area to explore.
Besides from the conceptual advantages of the
proposed algorithm, a few technical details may be
improved in further works. Exploring further color
channels besides the RGB space could bring benefits
to the proposed algorithm. Regarding fusion, explor-
ing individual specific parameters instead of a global
parametrization, would enable the algorithm to be
trained to counter the Doddington zoo effect. As not
all people are as easy to identify, fitting the properties
of the designed classification block to adapt to dif-
ferent classes of individuals seems like an interesting
idea.
Finally, and regarding the training setup, some
questions might be worthy of a more thorough re-
search. In the case of voice recognition it is com-
mon to train two separate UBMs for male and fe-
male speakers. Extrapolating this idea to image-based
traits, multiple UBMs trained on homogeneous sets
of equally or similarly zoomed images might improve
the results when more realistic and dynamic condi-
tions are presented to the acquisition system. In a re-
lated topic it is also not consensual whether the left
and right eyes, due to the intrinsic symmetry of the
face, should be considered in a single model or as
separate entities. All the aforementioned questions
demonstrate how much the present results can be im-
proved, leaving some promising prospects for future
works.
REFERENCES
Bakshi, S., Kumari, S., Raman, R., and Sa, P. K. (2012).
Evaluation of periocular over face biometric: A case
study. Procedia Engineering, 38:1628–1633.
Bharadwaj, S., Bhatt, H. S., Vatsa, M., and Singh, R.
(2010). Periocular biometrics: When iris recognition
fails. In 4th IEEE International Conference on Bio-
metrics: Theory Applications and Systems, pages 1–6.
Boddeti, V. N., Smereka, J. M., and Kumar, B. V. (2011). A
comparative evaluation of iris and ocular recognition
methods on challenging ocular images. In 2011 Inter-
national Joint Conference on Biometrics, pages 1–8.
IEEE.
Joshi, A., Gangwar, A. K., and Saquib, Z. (2012). Per-
son recognition based on fusion of iris and periocu-
lar biometrics. In Hybrid Intelligent Systems (HIS),
2012 12th International Conference on, pages 57–62.
IEEE.
Ke, Y. and Sukthankar, R. (2004). Pca-sift: A more
distinctive representation for local image descriptors.
In Computer Vision and Pattern Recognition, 2004.
CVPR 2004. Proceedings of the 2004 IEEE Computer
Society Conference on, volume 2, pages II–506. IEEE.
Kinnunen, T., Saastamoinen, J., Hautamaki, V., Vinni, M.,
and Franti, P. (2009). Comparing maximum a poste-
riori vector quantization and gaussian mixture models
in speaker verification. In IEEE International Con-
ference on Acoustics, Speech and Signal Processing,
pages 4229–4232. IEEE.
Kittler, J., Hatef, M., Duin, R. P., and Matas, J. (1998). On
combining classifiers. Pattern Analysis and Machine
Intelligence, IEEE Transactions on, 20(3):226–239.
Kumar, A. and Chan, T.-S. (2012). Iris recognition using
quaternionic sparse orientation code (qsoc). In 2012
IEEE Computer Society Conference on Computer Vi-
sion and Pattern Recognition Workshops, pages 59–
64.
Lowe, D. G. (2004). Distinctive image features from scale-
invariant keypoints. International journal of computer
vision, 60(2):91–110.
Miller, P. E., Lyle, J. R., Pundlik, S. J., and Woodard, D. L.
(2010a). Performance evaluation of local appearance
based periocular recognition. In IEEE International
Conference on Biometrics: Theory, Applications, and
Systems, pages 1–6.
Miller, P. E., Rawls, A. W., Pundlik, S. J., and Woodard,
D. L. (2010b). Personal identification using periocular
skin texture. In 2010 ACM Symposium on Applied
Computing, pages 1496–1500. ACM.
Moreno, J. C., Prasath, V., and Proenc¸a, H. (2013a). Ro-
bust periocular recognition by fusing local to holis-
tic sparse representations. In Proceedings of the 6th
International Conference on Security of Information
and Networks, pages 160–164. ACM.
Moreno, J. C., Prasath, V. B. S., Santos, G. M. M., and
Proenc¸a, H. (2013b). Robust periocular recognition by
fusing sparse representations of color and geometry
information. CoRR, abs/1309.2752.
Nabney, I. T. (2004). NETLAB: algorithms for pattern
recognition. Springer.
Padole, C. N. and Proenca, H. (2012). Periocular recog-
nition: Analysis of performance degradation factors.
In 5th IAPR International Conference on Biometrics,
pages 439–445.
Park, U., Jillela, R. R., Ross, A., and Jain, A. K. (2011).
Periocular biometrics in the visible spectrum. IEEE
Transactions on Information Forensics and Security,
6(1):96–106.
Park, U., Ross, A., and Jain, A. K. (2009). Periocular bio-
metrics in the visible spectrum: A feasibility study.
In IEEE 3rd International Conference on Biometrics:
Theory, Applications, and Systems, pages 1–6.
Povey, D., Chu, S. M., and Varadarajan, B. (2008). Univer-
sal background model based speech recognition. In
IEEE International Conference on Acoustics, Speech
and Signal Processing, pages 4561–4564.
Proenc¸a, H. (2009). NICE:II: Noisy iris challenge evalua-
tion - part ii. http://http://nice2.di.ubi.pt/.
Proenc¸a, H. (2011). Non-cooperative iris recognition: Is-
sues and trends. In 19th European Signal Processing
Conference, pages 1–5.
PeriocularRecognitionunderUnconstrainedSettingswithUniversalBackgroundModels
47