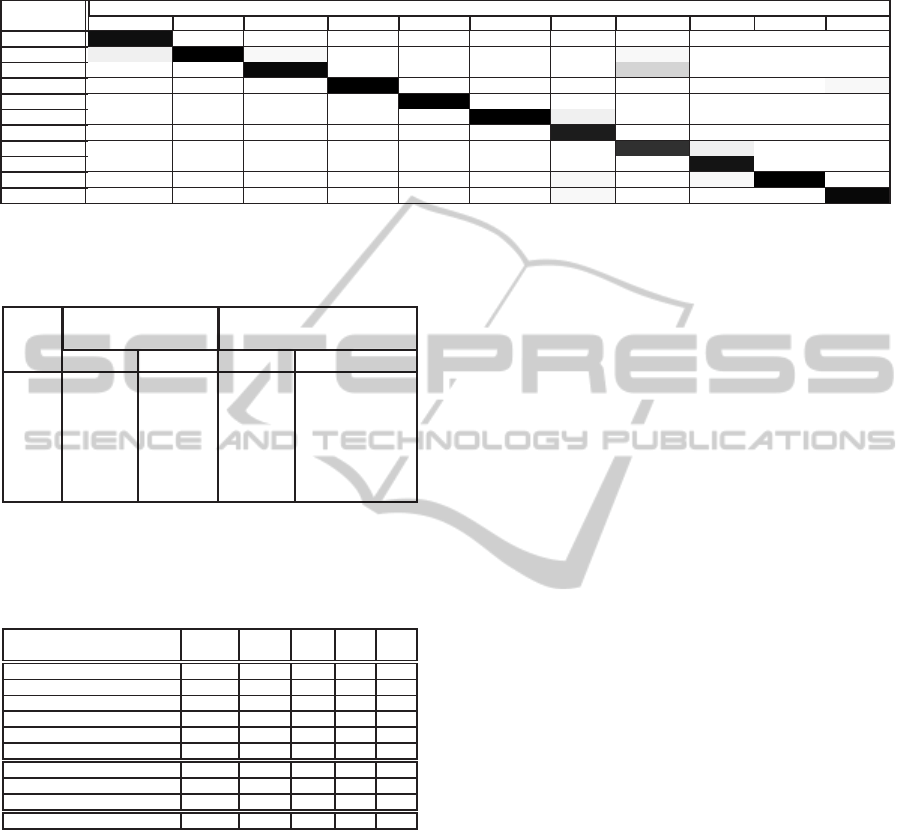
Table 4: Confusion matrix for IXMAS dataset: mask size L
x,y,z
= 4; average recognition rate = 95.5%; (·) represents the
number of trials recognized as each action class in 36 samples (12 actors × 3 trials).
Recognized Performed actions
actions Check watch Cross arm Scratch head Sit down Get up Turn around Walk Wave hand Punch Kick Pick up
Check watch 94.4(34) 0.0(0) 0.0(0) 0.0(0) 0.0(0) 0.0(0) 0.0(0) 0.0(0) 0.0(0) 0.0(0) 0.0(0)
Cross arm 5.6(2) 100.0(36) 2.8(1) 0.0(0) 0.0(0) 0.0(0) 0.0(0) 2.8(1) 0.0(0) 0.0(0) 0.0(0)
Scratch head 0.0(0) 0.0(0) 97.2(35) 0.0(0) 0.0(0) 0.0(0) 0.0(0) 16.7(6) 0.0(0) 0.0(0) 0.0(0)
Sit down 0.0(0) 0.0(0) 0.0(0) 100.0(36) 0.0(0) 0.0(0) 0.0(0) 0.0(0) 0.0(0) 0.0(0) 2.8(1)
Get up 0.0(0) 0.0(0) 0.0(0) 0.0(0) 100.0(36) 0.0(0) 0.0(0) 0.0(0) 0.0(0) 0.0(0) 0.0(0)
Turn around 0.0(0) 0.0(0) 0.0(0) 0.0(0) 0.0(0) 100.0(36) 5.6(2) 0.0(0) 0.0(0) 0.0(0) 0.0(0)
Walk 0.0(0) 0.0(0) 0.0(0) 0.0(0) 0.0(0) 0.0(0) 88.9(32) 0.0(0) 0.0(0) 0.0(0) 0.0(0)
Wave 0.0(0) 0.0(0) 0.0(0) 0.0(0) 0.0(0) 0.0(0) 0.0(0) 80.6(29) 5.6(2) 0.0(0) 0.0(0)
Punch 0.0(0) 0.0(0) 0.0(0) 0.0(0) 0.0(0) 0.0(0) 0.0(0) 0.0(0) 91.7(33) 0.0(0) 0.0(0)
Kick 0.0(0) 0.0(0) 0.0(0) 0.0(0) 0.0(0) 0.0(0) 2.8(1) 0.0(0) 2.8(1) 100.0(36) 0.0(0)
Pick up 0.0(0) 0.0(0) 0.0(0) 0.0(0) 0.0(0) 0.0(0) 2.8(1) 0.0(0) 0.0(0) 0.0(0) 97.2(35)
Table 5: LOAO recognition rate [%] for IXMAS dataset
with different feature extraction conditions; L
x,y,z
is voxel
size in the IXMAS format.
L
x,y,z
Whole body Separated into
upper and lower bodies
Filled Surface Filled Surface
1 84.3 % 81.1 % 87.4 % 89.1 %
2 91.2 % 88.1 % 94.4 % 90.7 %
3 90.9 % 91.9 % 94.7 % 92.7 %
4 92.7 % 91.7 % 93.7 % 95.5 %
5 93.4 % 91.4 % 91.9 % 93.2 %
6 92.2 % 92.7 % 90.7 % 90.7 %
Table 6: Comparison with 3D human action recognition ap-
proaches. The results for the LOAO cross-validation were
obtained using the IXMAS dataset. ‘Dim.’ denotes data
dimension used in the IXMAS dataset.
Approach Actions Actors Dim. Rate time
[%] [ms]
(Wu et al., 2011) 12 12 2D 89.4 N/A
(Pehlivan and Duygulu, 2010) 11 10 3D 90.9 N/A
(Weinland et al., 2006) 11 10 3D 93.3 N/A
(Cilla et al., 2013) 11 10 2D 94.0 N/A
(Turaga et al., 2008) 11 10 3D 98.8 N/A
(Holte et al., 2012) 13 12 3D 100 N/A
(Cherla et al., 2008) 13 N/A 2D 80.1 50
(Weinland et al., 2010) 11 10 2D 83.5 2∼
(Chaaraoui et al., 2014) 11 12 2D 91.4 5
4D HLAC approach 11 12 3D 95.5 *
* Computational costs of some implementation are shown in Table 3
6 CONCLUSION
In this article, we have proposed 4D HLAC for 3D
motion recognition. Our experimental results rein-
force the simplicity and low computational cost of
the proposed method, as well as its general versatil-
ity and performance. We conclude that 4D HLAC is a
highly capable and computationally efficient 3D mo-
tion recognition technique.
The next steps for the research are to extend multi
resolution analysis of 4D pattern from the split anal-
ysis in IXMAS experiment, to improve the classifica-
tion algorithm appropriate for 4D HLAC and apply it
to practical applications.
ACKNOWLEDGEMENTS
The work reported in this paper has been supported
by Grant-in-Aid Nos. 24680024, 24119001, and
24000012 from the Ministry of Education, Culture,
Sports, Science and Technology, Japan.
REFERENCES
Canny, J. (1986). A computational approach to edge de-
tection. IEEE Transactions on pattern analysis and
achine intelligence, pages 679–714.
Chaaraoui, A. A., Padilla-Lopez, J. R., Ferrandez-Postor,
F. J., Nieto-Hidalgo, M., and Florenz-Revuelta, F.
(2014). A vision-based system for intelligent monitor-
ing: human behaviour analysis and privacy by context.
Sensors, 14:8895–8925.
Cherla, S., Kulkarni, K., Kale, A., and Ramasubramanian,
V. (2008). Towards fast, view-invariant human action
recognition. In Proc. of the IEEE Conf. on Computer
Vision and Pattern Recognition Workshops.
Cilla, R., Patricio, M. A., Berlanga, A., and Molina, J. M.
(2013). Human action recognition with sparse classi-
fication and multiple-view learning. Expert Systems.
Holte, M., Chakraborty, B., Gonzalez, J., and Moeslund, T.
(2012). A local 3-d motion descriptor for multi-view
human action recognition from 4-d spatio-temporal
interest points. IEEE Journal of Sellected Topics in
Signal Process, 6:553–565.
Kanezaki, A., Harada, T., and Kuniyoshi, Y. (2010). Partial
matching of real textured 3d objects using color cu-
bic higher-order local auto-correlation features. The
Visual Computer, 26(10):1269–1281.
Kobayashi, T. and Otsu, N. (2004). Action and simultane-
ous multiple-person identification using cubic higher-
order local auto-correlation. In Proc. of 17th ICPR,
pages 741–744.
ICPRAM2015-InternationalConferenceonPatternRecognitionApplicationsandMethods
230