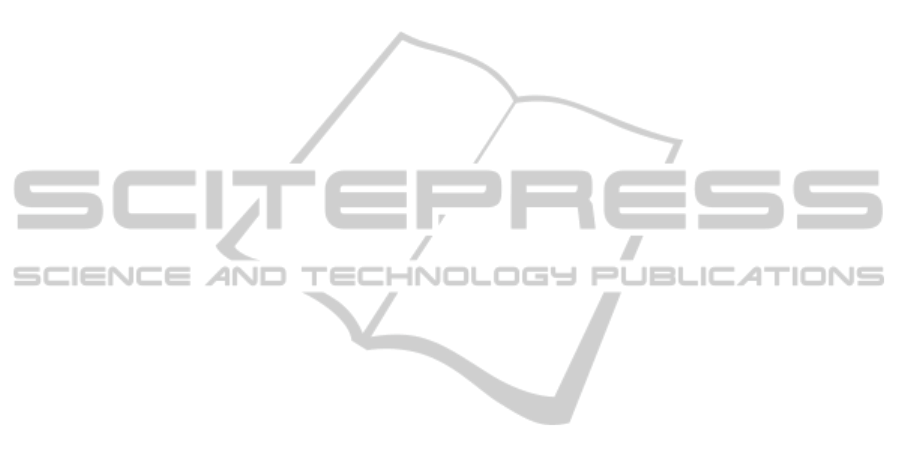
amenability to genetic manipulations (Gratacap and
Wheeler, 2014), the zebrafish is a novel and poten-
tial model organism to study the immunity. Fur-
thermore, the zebrafish and human immune systems
are remarkably similar and more than 75% of hu-
man genes implicated in diseases have counterparts
in zebrafish (Schier, 2013). This provides a strong
connection between the zebrafish and human on the
pathogenic mechanisms as well as immune responses,
which are important for biomedical applications. The
immune system of zebrafish as well as other verte-
brates can further be divided into two subsystems,
i.e., innate (unspecific) and adaptive (specific) im-
munity (Trede et al., 2004). Hence, the first dataset
we used to construct the dynamic host-pathogen PPI
network (HP-PPIN) measured the gene expression
profiles during the first 18 hours after zebrafish is
firstly exposed to a lethal dose of C. albicans (Chen
et al., 2013). This dataset sampled the gene ex-
pression profiles at 9 time points (i.e., 0.5, 1, 2, 4,
8, 12, 16, 18-hour post-injection) with three repli-
cates for C. albicans and zebrafish, respectively (Fig-
ure 1A). The outcomes of the interplay between the
host and pathogen after their first contact are cap-
tured by time course microarray experiments. Im-
mediately after the injection of C. albicans into ze-
brafish, the immune surveillance system of zebrafish
senses the existence of the invaders. The recogni-
tion of the pathogen-associated molecular patterns
(PAMPs) and/or damage-associated molecular pat-
terns (DAMPs) by the pattern recognition receptors
(PRRs) (e.g., toll-like receptors, C-type lectin recep-
tors, etc.) (Trede et al., 2004; Romani, 2011) may be
viewed as a starting point of a series of complex HPIs.
Those PRRs would initiate downstream pathways that
promote the activation of other parts of innate im-
mune system and the clearance of pathogens (e.g.,
production and secretion of cytokines, chemokines,
and chemotactic cues to recruit more immunocytes).
Thus, the morphological transitions (yeast-to-hyphal
form) (Kuo et al., 2013), required ions and small
molecules transportation (Wang et al., 2014), and
structures and components of molecules on the cell
wall changes (Romani, 2011) are the strategies uti-
lized by C. albicans to acquire nutrients and evade the
clearance from the host innate defensive mechanisms.
Those primary responses of the host and pathogen are
recorded in the first dataset which is a result from a
constant innate immune response and a delayed adap-
tive immune response (see Figure 1).
In addition to the activation of innate defensive
mechanisms, the PRRs and antigen presenting cells
would further activate the specific cells to clear the
pathogens much more efficiently (Romani, 2011). To
investigate the host-pathogen interactions in the spe-
cific defensive mechanisms (i.e., the adaptive immu-
nity), we adopted the second dataset. The experimen-
tal design of the second dataset comprises two C. al-
bicans injections into zebrafish. In the first injection,
a nonlethal dose is used to cause the host primary re-
sponses and immunological memory in the host. And
in 14 days after the first injection, a lethal dose is
applied to zebrafish. Then, the gene expression pro-
files are recorded through microarray after the second
injection and comprise 8 time points (2, 6, 12, 18,
24, 30, 36, 42-hour post-reinjection) with two repli-
cates for C. albicans and zebrafish, respectively (Fig-
ure 1A). Due to the previous exposure to C. albicans,
the immunological memory in zebrafish would be ac-
tivated and force the activation of the adaptive im-
munity. Thus, the main portion of the information
recorded in the second dataset reflects the outcomes
of the HPIs in the adaptive immunity. In contrast
to the innate immunity, the adaptive immunity of ze-
brafish and the responses of C. albicans to the adap-
tive immunity are less well-known. Here, in this study
the combination of these two datasets provides an op-
portunity to investigate the HPIs and their roles in the
innate and adaptive immunity.
The infection processes are often described as bat-
tles between the host and pathogen (Leroy and Raoult,
2010). The simultaneous considerations on the roles
of the host and pathogen in the infection processes
and the available genome-wide measurements make
the possibility of the systematic viewpoints on the ef-
fects of the HPIs in the innate and adaptive immu-
nity (Wang et al., 2013). In this study, the usage of
the C. albicans-zebrafish infection model (Chao et al.,
2010) shed light on the infectious diseases of the hu-
man host. As for the pathogen, the C. albicans is
the most virulent member of the CUG clade of yeasts
and a common cause of both superficial and invasive
infections (Lohberger et al., 2014) which may cause
life-threatening infections in immune-comprised host
(Odds, 1979), such as HIV positive patients. Investi-
gating the infection processes of C. albicans in detail
can improve the knowledge of the pathogenic mecha-
nisms and promote the control of infectious diseases.
Hence, the two datasets (GSE32119 and GSE51603)
are used for further analyses on the HPIs. To extract
the interaction information from the time course mi-
croarray data, two dynamic HP-PPINs are built up
for innate and adaptive immunity (Wang et al., 2013).
The HP-PPINs consist of the PPIs between zebrafish
and C. albicans, zebrafish and zebrafish, and C. albi-
cans and C. albicans. Through examining the interac-
tion variation between the innate and adaptive PPINs,
the interactions of the largest difference in the net-
BIOINFORMATICS2015-InternationalConferenceonBioinformaticsModels,MethodsandAlgorithms
18