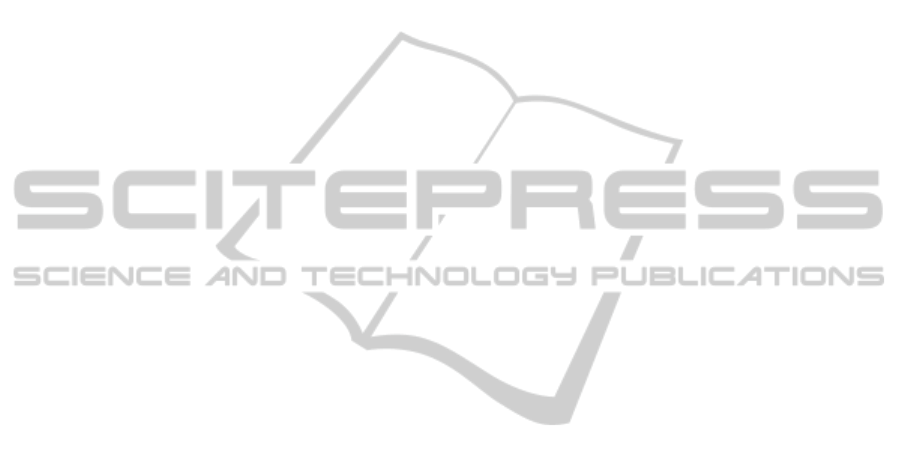
tions. This system will cover various critical aspects
of personal health, including sleep quality, weight,
blood pressure, blood glucose, mood, etc. We will
collect feedback from the users based on which exten-
sive evidence-based study will be conducted to verify
the effectiveness of this framework.
REFERENCES
Almalki, M., Lopez-Gampos, G., Martin-Sanchez, F., and
Gray, K. (2014). Classification of data and activities
in self-quantification systems. Proceedings of HISA
BIG DATA 2014 conference, pages 18–19.
Berengueres, J. (2012). The Toyota production system re-
contextualized. lulu.com.
Buysse, D., Reynolds, C., Monk, T., Berman, S., and
Kupfer, D. (1989). The pittsburge sleep quality index:
a new instrument for psychiatric practice and research.
Psychiatry Res, 28:193–213.
Casson, R. J. (2011). The pesty p value. Clinical & Exper-
imental Ophthalmology, 39(9):849–850.
Clifton, L., Clifton, D. A., Pimentel, M. A. F., Watkin-
son, P. J., and Tarassenko, L. (2013). Gaussian pro-
cesses for personalized ehealth monitoring with wear-
able sensors. Biomedical Engineering, IEEE Transac-
tions on, 60(1):193–197.
CNET (2014). Best storage devices of 2014. Available on-
line at http://www.cnet.com/topics/storage/best-hard-
drives-and-storage/ (Last accessed on July 28, 2014).
Code42 (2014). Crashplan. http://www.code42.com/
crashplan/features/compare/ (Last accessed on July
28, 2014).
Doi, Y., Minowa, M., and Tango, T. (2003). Impact and cor-
relates of poor sleep quality in japanese white-collar
employees. Sleep, 26(4):467–471.
Fox, S. and Duggan, M. (2013). Tracking for health. In Pew
Research Center’s Internet and American Life Project.
Group, D. P. P. R. (2012). The 10-year cost-effectiveness of
lifestyle intervention or metformin for diabetes pre-
vention: an intent-to-treat analysis of the dpp/dppos.
Diabetes Care, 35(4):723–730.
Harman, H. H. (1976). Modern Factor Analysis. University
of Chicago Press.
HealthVault (2014). https://www.healthvault.com/ (Last ac-
cessed on July 28, 2014).
HL7 (viewed on July 28, 2014). Introduction to hl7 stan-
dard. http://www.hl7.org/implement/standards/.
Juniper (2013). Mobile health & fitness: monitoring, app-
enabled devices & cost savings 2013-2018.
JustCloud (2014). http://www.justcloud.com/ (Last ac-
cessed on July 28, 2014).
Kampmeier, C. (viewed on July 31, 2014). Home net-
work data storage and backup. Available online at
http://kampmeier.com/chris/blog/?p=8.
Lewis, D. (2014). Letting go of things we cant control:
remembering that sleep matters. https://scottleibrand.
wordpress.com/2014/07/25/letting-go-of-things-
we-cant-control-remembering-that-sleep-matters-
diyps/.
Mathers, C. D. and Loncar, D. (2006). Projections of global
mortality and burden of disease from 2002 to 2030.
PLoS Med, 3(11):e442.
Mehl, M. R. and Conner, T. S. (2012). Handbook of Re-
search Methods for Studying Daily Life. The Guilford
Press.
MyPCBackup (2014). http://www.mypcbackup.com/ (Last
accessed on July 28, 2014).
Nuzzo, R. (2014). Scientific method: Statistical errors. Na-
ture, 506:150–152.
Pearson, K. (1895). Notes on regression and inheritance
in the case of two parents. Proceedings of the Royal
Society of London, 58:240–242.
Philip, B. A. and Danner, F. J. (1995). Cigarette smoling and
sleep disturbance. Arch Intern Med, 155:734–737.
Poelstra, P. A. M. (1984). Relationship between phys-
ical, psychological, social, and environmental vari-
ables and subjective sleep quality. Sleep, 7(3):255–
260.
Pyzdek, T. and Keller, P. (2014). The Six Sigma Handbook.
McGraw-Hill Education, 4 edition.
ReVelle, J. B. (2004). Quality Essentials: A Reference
Guide from A to Z. ASQ Quality Press.
Shuttleworth, M. (viewed on 31 July, 2014). De-
sign of experiment. Available online at
https://explorable.com/design-of-experiment.
Stepnowsky, C. J., J., M. P., and Dimsdale, J. E. (2003). Ef-
fect of ethnicity on sleep: complexities for epidemio-
logic research. Sleep, 26(3):329–332.
Stieber, J. (2005). preventive health care in australia: The
shape of the elephant, reliance on evidence, and com-
parisons to u.s. medicare.
Swan, M. (2013). The quantified self: fundamental disrup-
tion in big data science and biological discovery. Big
Data, 1(2):85–99.
Thomas, R. J. (2012). Seeking useful biomarkers for the
quality and effectiveness of sleep. Sleep, 35(2):173–
174.
Utts, J. M. (2005). Seeing Through Statistics. Thomson
Brooks, 3 edition.
Vitiello, M. V., Larsen, L. H., and Moe, K. E. (2004). Age-
related sleep change: Gender and estrogen effects on
the subjectiveobjective sleep quality relationships of
healthy, noncomplaining older men and women. Jour-
nal of Psychosomatic Research, 56(5):503–510.
Whittaker, R., Merry, S., Dorey, E., and Maddison, R.
(2012). A development and evaluation process for
mhealth interventions: examples from new zealand.
J Health Commun, 17(Suppl 1):11–21.
WHO (2011). The top 10 causes of death.
FramingSelf-quantificationforIndividual-levelPreventiveHealthCare
343