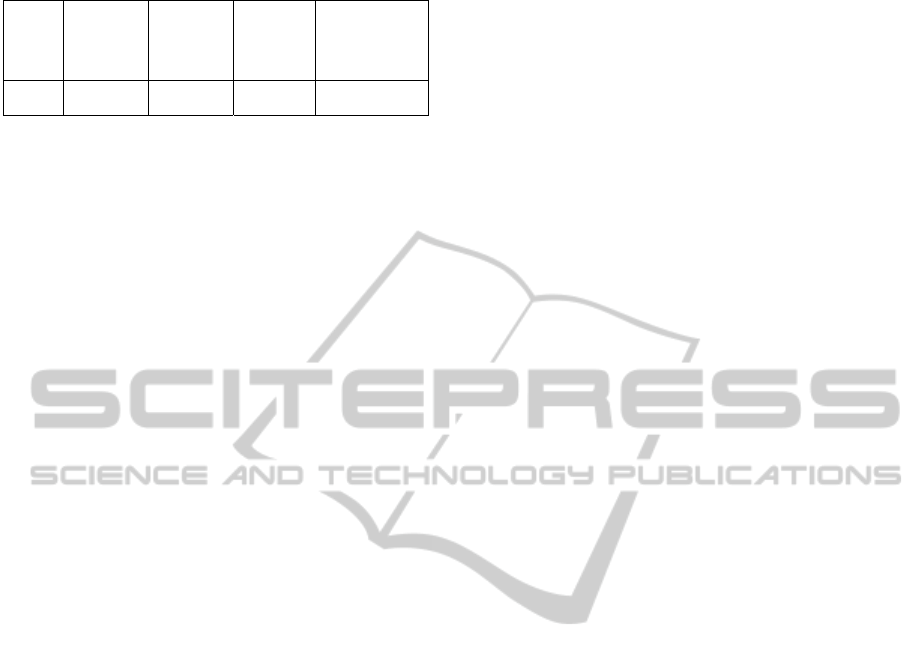
Table 3: HeartSafe dataset results.
ECG
Ch1
ECG
Ch2
PCG ECG Ch1
Filt
3.29E-4 1.67E-7 1.87E-6 2.48E-7
We also compare the fitting capability of ECG and
PCG to AR linear models (Table 3). We make an
assumption that if a signal is more linearly
predictable than another one, it may adjust better to
these AR linear models. The HeartSafe dataset
results showed that filtered ECG is a more linearly
predictable signal than filtered PCG. The first ECG
channel exhibits higher noise levels when compared
to the second one, as a consequence ̅
is greater in
the first channel making it a more unreliable
channel.
7 CONCLUSIONS
Using a null hypothesis test, we concluded with 99%
of confidence that the PCG and ECG data came
from a deterministic system, although potentially
contaminated with a broad type of noises.
The FNN statistic revealed itself to be insufficient to
extract an embedding dimension from both PCG and
ECG signals, simply because it was never observed
a zero fraction of false neighbours. Therefore any
attempt to build a phase space turns to be
insufficient to completely describe the dynamical
system so the embedding dimension does not insure
a deterministic mapping. This can be caused by the
measurement noise (error which is independent of
the system, where all observations are contaminated
by some amount) or dynamical noise (feedback
process where in the system is perturbed by some
amount in each time step (Schreiber, 1996)).
Dynamical noise may sometimes be a higher
dimensional part of the dynamics with small
amplitude. At least one type of the dynamical noise
in a PCG is not static but it is periodic or quasi-
periodic and it depends on the breathing cycle,
making the analysis of PCG a more difficult task.
Finally, in the HeartSafe dataset, ECG revealed to be
a more linearly predictable signal when compared to
the PCG, although a filtering step is needed in
channel 1. Therefore, in order to improve the
predictability of a multi-signal acquisition system ,
we suggest to have more PCG than ECG channels,
since they are more linearly unpredictable signals.
ACKNOWLEDGEMENTS
This work was partially funded by the Fundação
para a Ciência e Tecnologia (FCT, Portuguese
Foundation for Science and Technology) under the
reference Heart Safe PTDC/EEI-PRO/2857/2012;
and Project I-CITY - ICT for Future
Health/Faculdade de Engenharia da Universidade do
Porto, NORTE-07-0124-FEDER-000068, Pest-
OE/EEI/LA0008/2013.
REFERENCES
D. T. Kaplan and L.Glass, Phys. Rev. Lett 68, 427 (1992).
D. T. Kaplan and L.Glass, Phys. Rev. Lett 64, 431 (1993).
T. Schreiber and A.Schmitz, Phys. Rev. Lett. 77. 635
(1996).
M. Kennel, H. Abarbanel, False neighbours and false
strands: A reliable minimum embedding dimension al-
gorithm, Phys. Rev.E, Vol 66, Nub 4, (2002).
A. Guyton, J.E.Hall, Textbook of Medical Physiology.
Elsevier Saunders, 11th ed, Ed Hall, (Jun 2006).
R. Hegger, H.Kantz, Improved false nearest neighbour
method to detect determinism in the time series data,
Phys. Rev. E, Vol 60, Numb 4, (Oct 1999).
T. Schreiber and A.Schmitz, Surrogate time series Physica
D, vol. 142, no 3-4, pp 34-382, (2000).
The TISEAN Software packet of Hegger, H. Kantz and T.
Schreiber can be download for free from :
http://www.mpipks-dresden.mpg.de/~tisean/
M. B. Kennel, R.Brown, and H.D.I Abarbanel, Phys. Rev.
A 45, 3403 (1992).
J. F. Kaiser, System Analysis by Digital Computer, chap. 7.
New York, Wiley (1996).
H. Kantz, T. Schreiber, Nonlinear Time Series Analysis ,
2th ed. Vol .3, Ed. Cambridge University Press, ( Jan
2004).
R. B. Govindan, K. Narayanan, and M. S. Gopinathan On
the evidence of deterministic chaos in ECG: Surrogate
and predictability analysis, Vol .8, Numb 2, Chaos
(June 1998).
CanWeFindDeterministicSignaturesinECGandPCGSignals?
189