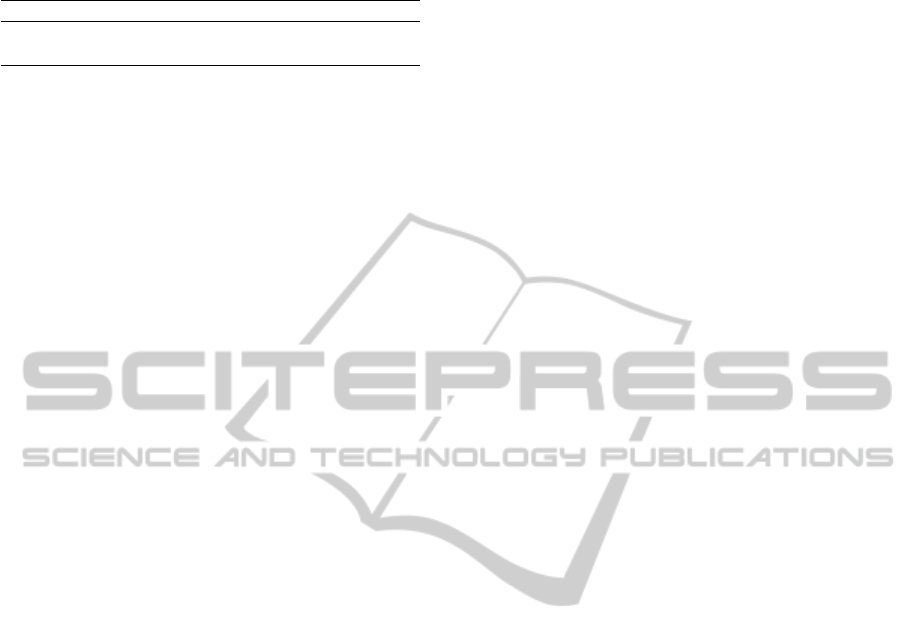
Table 1: Pollution Emission Level Comparison between IGCC
and Conventional PCT.
SO
X
NO
X
Particulate
IGCC
1
1.0 ppm 3.4 ppm < 0.1 mg/m
3
N
PCT
2
< 17.7 ppm < 49.4 ppm < 20 mg/m
3
N
1
Joban Joint Power: http://www.joban-power.co.jp/igccdata/en/
2
Shenhua Guohua Beijing Cogeneration: http://www.shghrd.
com/HistoryData.aspx
to define the allocation rule either by a proportional
game or a queue game exogenously.
To illustrate our model, consider the following
case. According to a statement released after the State
Council’s executive meeting presided by Premier Li
Keqiang, Chinese central government has laid out a
number of detailed measures that identify large cities
and regions with the most frequent smog and haze as
key areas in the battle against air pollution. A spe-
cial fund of 10 billion yuan ($1.65 billion) is set up
in 2014 to reward efforts to curb air pollution in the
key areas. Suppose the central government is provid-
ing a fixed amount of clean air fund (benefit) and calls
for proposals from both domestic and overseas orga-
nizations. Successful applicants (players) have ad-
vantages in different criteria, namely, the sustainabil-
ity indicators. For example, applicant A (i.e., Joban
Joint Power, Japan) owns IGCC (integrated gasifica-
tion combined cycle) technology, which is a type of
gasification technology that turns coal into gas, and
can potentially improve the efficiency of coal-fired
power compared to conventional pulverized coal tech-
nology (PCT) as well as the environmental perfor-
mance (Table 1). Applicant B (i.e., Shenhua Guohua
Beijing Cogeneration, China) has advantage in good
source of clean coal with low sulfur content and low
installation cost of new coal-fired power plant. The
central government has to decide a reasonable alloca-
tion of the fund to these successful applicants, which
should reach the consensus among them. In DEA
game, as assumed by (Nakabayashi and Tone, 2006),
the successful applicants are egoistic and each of them
sticks to his superiority regarding the sustainability
indicators, i.e., efficiency, cleaner resource, pollution
emission, installation cost, maintenance cost and etc.
The process above is similar to a two-stage tender-
ing with technical and financial proposals submitted
separately. In the first stage, organizations A, B, C ...
submit their technical proposals without fund claim,
in accordance with the specifications by the central
government and their respective performance on dif-
ferent criteria. The technical proposals are evaluated
and successful applicants are announced. The second
stage is to invite the successful applicants to vote for
the importance of all the criteria. The fund allocation
is endogenously determined by DEA game with ref-
erence to this voting result. Our paper focuses on the
second stage.
In the literature, DEA is generally introduced as a
mathematical programming approach for measuring
relative efficiencies of decision-making units (DMUs,
in DEA game we use “player” instead), where multi-
ple inputs and multiple outputs are present. The in-
terest of this paper can be best justified by the latest
technical note by (Cook et al., 2014), they emphasized
that
“Ultimately DEA is a method for performance evaluation
and benchmarking against best-practice. The inputs are
usually the ‘less-the-better’ type of performance measures
and the outputs are usually the ‘more-the-better’ type of per-
formance measures. This case is particularly relevant to the
situations where DEA is employed as a MCDM (multiple
criteria decision making) tool. DEA then can be viewed
as a multiple-criteria evaluation methodology where DMUs
are alternatives, and DEA inputs and outputs are two sets
of performance criteria where one set (inputs) is to be min-
imized and the other (outputs) is to be maximized.”
In the case above, the central government has
taken the trouble to apply multiple criteria to deter-
mine the allocation of the fund, as a single criterion is
hardly to become an acceptable basis of a fair judg-
ment. Different interest group, either a single appli-
cant, or a coalition, has different opinion on the im-
portance of each criterion for estimating a reasonable
allocation. Hence DEA is employed as a MCDM tool,
where the DMUs are the successful applicants, and
output-to-input ratio is the relative contribution of one
DMU compared to the total contribution of all DMUs.
Here “contribution” is equivalent to “performance”,
which is quantitatively measured by the score given
to each DMU by different criteria. This representa-
tion, presented in the next section, is not a typical effi-
ciency measure within the context of maximizing the
ratio of weighted outputs to weighted inputs subject
to constraints reflecting the performance of the other
DMUs; whilst it is the ratio of the fund each DMU
may receive by integrating the performance of other
DMUs in the objective function. (Nakabayashi et al.,
2009) justified this representation by addressing the
fundamental concept of DEA–variable weights. The
weights are derived directly from the existing data
and they are chosen in a manner that assigns a best
set of weights to each DMU. The term “best” means
that the resulting output-to-input ratio for each DMU
is maximized relative to all other DMUs when these
weights are assigned to these outputs and inputs for
every DMU.
(Nakabayashi and Tone, 2006) first proposed
DEA game to solve this kind of multi-criteria benefit
allocation problem. Their DEA max game was, how-
ICORES2015-InternationalConferenceonOperationsResearchandEnterpriseSystems
108