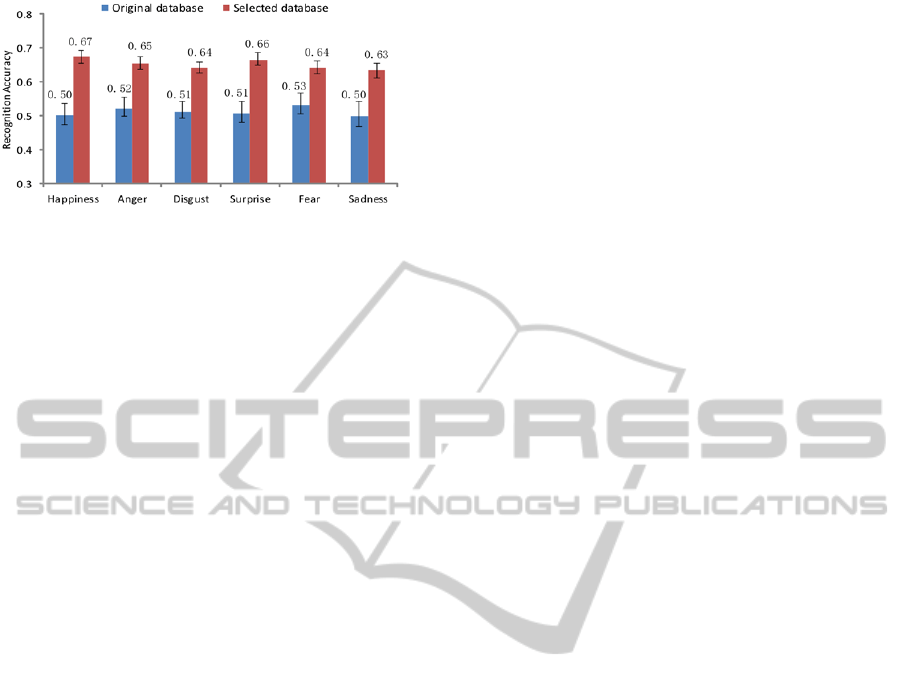
Figure 10: Recognition accuracies using original and
selected databases.
The recognition accuracy can be regarded as
reflecting the quality of the corresponding training
database. As shown in Figure 10, a great difference
in two-level arousal recognition performance
appears among these two databases. The quality of
the selected database is obviously higher than that of
the original database. Such a result also proves that
the EEG-based data selection method is an effective
way to improve the quality of an emotion database.
5 CONCLUSION AND FUTURE
PERSPECTIVES
In this research, we carried out arousal-related
studies on EEG signals because they are an
important area of affective computing. Firstly, by
applying a cross-level feature selection method and
the optimal channel groups, the recognition accuracy
for two-level arousal recognition has been improved
to 95.4%, which is better than the results from the
full channel test. Adoption of the optimal channel
groups shows that the individual difference in EEG
signals is very large.
Secondly, the discussion on common channels is
fulfilled based on two rankings of SCP and ANOVA.
Common channels also show a good performance of
91.1%. The 5-channel group provides some ideas for
further study on arousal-related sensitive channels.
Finally, in database validation, an EEG-based
data selection method is useful to select the typical
emotional data from the original database. And this
method has been proved to be effective by
evaluating the quality of the selected database.
However, there are still some points that need to
be studied:
1. Other arousal-related features. In this research,
four kinds of statistical features for detecting
arousal status were discussed. However, there
are still other features that we did not mention.
2. For common channels, 5 channels are simply
selected out of 16 channels. However, the other
channel combinations were not studied.
REFERENCES
Aftanas, L. I., Reva, N. V., Varlamov, A. A., Pavlov, S. V.,
& Makhnev, V. P. 2004. Analysis of Evoked EEG
Synchronization and Desynchronization in Conditions
of Emotional Activation in Humans: Temporal and
Topographic Characteristics. Neuroscience and
Behavioral Physiology. 34(8). pp.859-867.
Ekman, P., Friesen. W. V., & Ellsworth. P. 1972. Emotion
in the human face: Guidelines for research and an
integration of findings. New York: Pergamon Press.
Hosseini, S. A., & Naghibi-Sistani. M. B. 2011. Emotion
recognition method using entropy analysis of EEG
signals. I. J. Image, Graphics and Signal Processing.
3(5). pp.30-36.
Lane, R. D., & Jennings, J. R. 1995. Hemispheric
asymmetry, autonomic asymmetry, and the problem of
sudden cardiac death. In R.J. Davidson, & K. Hugdahl
(Eds), Brain asymmetry. pp. 271-304. Cambridge,
MA: MIT Press.
Lang, Peter J., Bardley, Margaret M., Fitzismmons,
Jeffrey R., Cuthbert, Bruce N., Scott, James D.,
Moulder, B., & Nangia, V. 1998. Emotional arousal
and activation of the visual cortex: An fMRI analysis:
Psychophysiology. 35. pp. 199-210.
Liu, Y., & Sourina, O. 2012. EEG-based Valence Level
Recognition for Real-Time Applications. International
conference on Cyberworlds. pp.53-60.
Murugappan, M., Rizon, M., RNagarajan, Yaacob, S.,
Zunaidi, I., &Hazry, D. 2007. EEG Feature Extraction
for Classifying Emotions using FCM and FKM.
International Journal of Computers and
Communications. 2(1). pp. 21-25.
Musha, T., Terasaki, Y., Haque, Hasnine A., & Ivanitsky,
George A. 1997. Feature Extraction from EEGs
Associated with Emotions. Artif Life Robotics. 1(1).
pp.15-19.
Russell, James A. 1980. A Circumplex Model of Affect.
Journal of Personality and Social Psychology. 39(6).
pp.1161-1178.
Sander, D., Grandjean, D., & Scherer, K. R. 2005. A
systems approach to appraisal mechanisms in emotion.
Neural Networks. 18. pp. 317-352.
Soleymani, M., Lichtenauer, J., Pun, T., & Pantic, M.
2012. A Multimodal Database for Affect Recognition
ArousalRecognitionMethodusingElectroencephalographySignalstoConstructEmotionalDatabase
365