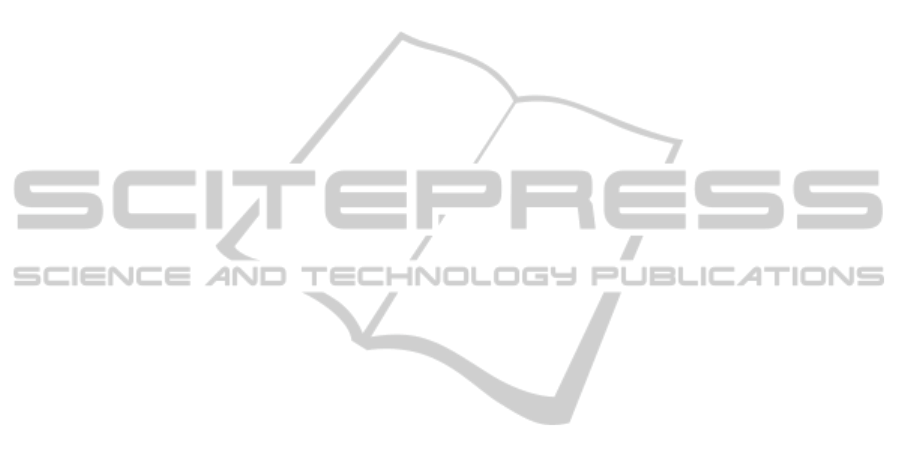
REFERENCES
Altschul, S. F., Madden, T. L., Schaffer, A. A., Zhang,
J., Zhang, Z., Miller, W., and Lipman, D. J. (1997).
Gapped BLAST and PSI-BLAST: A new generation
of protein database search programs. 25:3389–3402.
Aydin, Z., Singh, A., Bilmes, J., and Noble, W. S. (2011).
Learning sparse models for a dynamic Bayesian net-
work classifier of protein secondary structure. BMC
Bioinformatics, 12:154.
Aydin, Z., Thompson, J., Bilmes, J., Baker, D., and Noble,
W. S. (2012). Protein torsion angle class prediction
by a hybrid architecture of bayesian and neural net-
works. In 13th International Conference on Bioinfor-
matics and Computational Biology.
Berjanskii, M. V., Neal, S., and Wishart, D. S. (2006).
PREDITOR: a web server for predicting protein tor-
sion angle restraints. Nucleic Acids Research, 34(Web
Server Issue):W:63–69.
Blum, B., Jordan, M., Kim, D., Das, R., Bradley, P., and
Baker, D. (2008). Feature selection methods for im-
proving protein structure prediction with Rosetta. In
Platt, J., Koller, D., Singer, Y., and Roweis, S., editors,
Advances in Neural Information Processing Systems
20, pages 137–144. MIT Press, Cambridge, MA.
Cheng, J., Tegge, A. N., and Baldi, P. (2008). Ma-
chine learning methods for protein structure predic-
tion. IEEE Reviews in Biomedical Engineering, 1:41–
49.
Cong, P., Li, D., Wang, Z., Tang, S., and Li, T. (2013).
Spssm8: An accurate approach for predicting eight-
state secondary structures of proteins. Biochimie,
95(12):2460–2464.
Faraggi, E., Zhang, T., Yang, Y., Kurgan, L., and Zhou, Y.
(2012). SPINE X: improving protein secondary struc-
ture prediction by multistep learning coupled with
prediction of solvent accessible surface area and back-
bone torsion angles. PLoS One, 7(2):e30361.
Henikoff, S. and Henikoff, J. G. (1994). Position-based se-
quence weights. 243:574–578.
Hobohm, U. and Sander, C. (1994). Enlarged representative
set of protein structures. Protein Science, 3:522–524.
Jones, D. T. (1999). Protein secondary structure prediction
based on position-specific scoring matrices. 292:195–
202.
Li, D., Li, T., Cong, P., Xong, W., and Sun, J. (2012). A
novel structural position-specific scoring matrix for
the prediction of protein secondary structures. Bioin-
formatics, 28(1):32–39.
Mooney, C. and Pollastri, G. (2009). Beyond the twilight
zone: Automated prediction of structural properties of
proteins by recursive neural networks and remote ho-
mology information. Proteins: Structure, Function,
and Bioinformatics, 77:181–190.
Pollastri, G., Martin, A. J. M., Mooney, C., and Vullo,
A. (2007). Accurate prediction of protein secondary
structure and solvent accessibility by consensus com-
biners of sequence and structure information. BMC
Bioinformatics, 8(201).
Remmert, M., Biegert, A., Hauser, A., and Soding, J.
(2011). Hhblits: lightning-fast iterative protein se-
quence searching by hmm-hmm alignment. Nature
Methods, 9(2):173–175.
Shen, Y., Delaglio, F., Cornilescu, G., and Bax, A. (2009).
TALOS+: a hybrid method for predicting protein
backbone torsion angles from nmr chemical shifts.
Journal of Biomolecular NMR, 44(4):213–223.
Singh, H., Singh, S., and Raghava, G. P. S. (2014). Eval-
uation of protein dihedral angle prediction methods.
PLoS One, 9(8):e105667.
Soding, J. (2005). Protein homology detection by HMM-
HMM comparison. Bioinformatics, 21:951–960.
Soding, J. (2006). Quick guide to HHsearch.
ftp://toolkit.genzentrum.lmu.de/pub/HHsearch/old/
HHsearch/HHsearch1.5.1/HHsearch-guide.pdf.
Soding, J., Remmert, M., and Hauser, A. (2012). HH-suite
for sensitive sequence searching based on hmm-hmm
alignment. ftp://toolkit.genzentrum.lmu.de/pub/HH-
suite/hhsuite-userguide.pdf.
Song, J., Tan, H., Wang, M., Webb, G. I., and Akutsu, T.
(2012). TANGLE: two-level support vector regression
approach for protein backbone torsion angle predic-
tion from primary sequences. PLoS One, 7(2):e30361.
Sun, J., Tang, S., Xiong, W., Cong, P., and Li, T. (2012).
Dsp: a protein shape string and its profile predic-
tion server. Nucleic Acids Research, 40(W1):W298–
W302.
Walsh, I., Bau, D., Martin, A. J. M., Mooney, C., Vullo,
A., and Pollastri, G. (2009). Ab initio and template-
based prediction of multi-class distance maps by two-
dimensional recursive neural networks. BMC Struc-
tural Biology, 9(5).
Wang, G. and Dunbrack, Jr., R. L. (2003). PISCES:
a protein sequence culling server. Bioin-
formatics, 19:1589–1591. Web server at
http://dunbrack.fccc.edu/PISCES.php.
Wang, G. and Dunbrack, Jr., R. L. (2005). PISCES: re-
cent improvements to a pdb sequence culling server.
Nucleic Acids Res., 33:W94–W98. Web server at
http://dunbrack.fccc.edu/PISCES.php.
Wu, S. and Zhang, Y. (2008a). ANGLOR: A composite
machine-learning algorithm for protein backbone tor-
sion angle prediction. PLoS One, 3(10):e3400.
Wu, S. and Zhang, Y. (2008b). MUSTER: Improving pro-
tein sequence profile-profile alignments by using mul-
tiple sources of structure information. Proteins: Struc-
ture, Function, and Bioinformatics, 72(2):547–556.
Zemla, A., Venclovas, C., Fidelis, K., and Rost, B. (1999).
A modified definition of Sov, a segment-based mea-
sure for protein secondary structure prediction assess-
ment. Proteins, 34:220–223.
ConstructingStructuralProfilesforProteinTorsionAnglePrediction
35