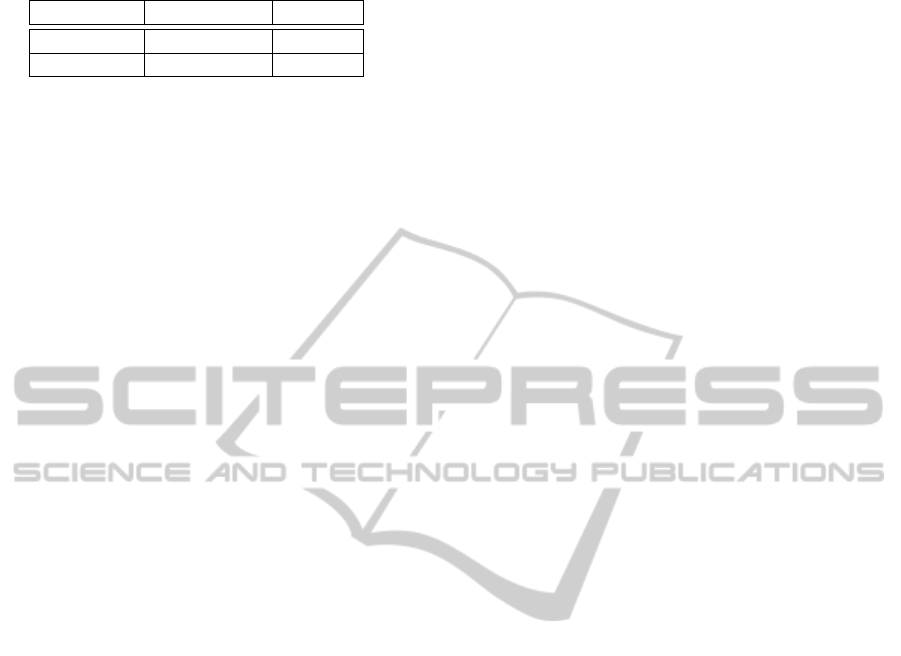
Table 1: Classifying graphs of GREC (C
T
= 500 ms).
Algorithms t AC
A*GED 119491.5 ms 42.23%
DF-GED 60468.7 ms 98.48 %
6 CONCLUSION AND
PERSPECTIVES
In the present paper, we have considered the prob-
lem of GED computation for PR. Graph edit distance
is a powerful and flexible paradigm that has been
used in different applications in PR. The exact algo-
rithm, A*GED, presented in the literature suffers from
high memory consumption and thus is too costly to
match large graphs. In this paper, we propose another
exact GED algorithm, DF-GED, which is based on
depth-first search. This algorithm speeds up the com-
putations of graph edit distance thanks to its upper
and lower bounds pruning strategy and its preprocess-
ing step. Moreover, this algorithm does not exhaust
memory as the number of pending edit paths that are
stored in the set OPEN is relatively small thanks to
the depth-first search where the number of pending
nodes is |V1|.|V 2| in the worst case.
In the experimental section, we have proposed to
evaluate sub-optimally both exact methods: A*GED
and DF-GED under some memory and time con-
straints. Experiments on the GREC database em-
pirically demonstrated that DF-GED outperforms
A*GED in terms of precision, speed and classification
rate. In future work, we aim at proposing a bench-
mark to measure the quality of the solutions found by
approximate methods. Both exact and approximate
graph edit distance computations will be evaluated on
different PR databases.
REFERENCES
Andreas Fischer, Ching Y. Suen, V. F. K. R. H. B. (2013). A
fast matching algorithm for graph-based handwriting
recognition. GbRPR 2013, 7877:194–203.
Andrew D. J. Cross, E. R. H. (1998). Graph matching with
a dual-step em algorithm. IEEE Trans. Pattern Anal.
Mach. Intell., 20(11):1236–1253.
Christmas, W., Kittler, J., and Petrou, M. (1995). Struc-
tural matching in computer vision using probabilistic
relaxation. Pattern Analysis and Machine Intelligence,
IEEE Transactions on, 17(8):749–764.
Cross, A. D., Wilson, R. C., and Hancock, E. R. (1997).
Inexact graph matching using genetic search. Pattern
Recognition, 30(6):953–970.
Dosch, P. and Valveny, E. (2006). Report on the second
symbol recognition contest. 3926:381–397.
Fankhauser, S., Riesen, K., Bunke, H., and Dickinson, P. J.
(2012). Suboptimal graph isomorphism using bipar-
tite matching. IJPRAI, 26(6).
Finch, A., Wilson, R., and Hancock, E. (1998). An energy
function and continuous edit process for graph match-
ing. Neural Computation, 10(7):1873–1894.
Justice, D. and Hero, A. (2006). A binary linear program-
ming formulation of the graph edit distance. IEEE
Transactions on Pattern Analysis and Machine Intel-
ligence, 28(8):1200–1214.
Kuner, P. and Ueberreiter, B. (1988). Pattern recognition
by graph matching: Combinatorial versus continuous
optimization. International Journal in Pattern Recog-
nition and Artificial Intelligence, 2(3):527–542.
M. Neuhaus, K. R. and Bunke., H. (2006). Fast suboptimal
algorithms for the computation of graph edit distance.
Proceedings of 11th International Workshop on Struc-
tural and Syntactic Pattern Recognition., 28:163–172.
Riesen, K. and Bunke, H. (2008). Iam graph database repos-
itory for graph based pattern recognition and machine
learning. pages 287–297.
Riesen, K. and Bunke, H. (2009). Approximate graph
edit distance computation by means of bipartite graph
matching. Image and Vision Computing, 27(7):950–
959.
Riesen, K. and Bunke, H. (2010). Graph Classification and
Clustering Based on Vector Space Embedding.
Riesen, K., Fankhauser, S., and Bunke, H. (2007). Speed-
ing up graph edit distance computation with a bipartite
heuristic. In Mining and Learning with Graphs, MLG
2007, Proceedings.
Sanfeliu, A. and Fu, K. (1983). A distance measure be-
tween attributed relational graphs for pattern recogni-
tion. IEEE Transactions on Systems, Man, and Cyber-
netics, 13(3):353–362.
Tsai, W.-H. and Fu, K.-S. (1979). Error-correcting isomor-
phisms of attributed relational graphs for pattern anal-
ysis. Systems, Man and Cybernetics, IEEE Transac-
tions on, 9(12):757–768.
Tsai, W.-H. and Fu, K.-S. (1983). Subgraph error-correcting
isomorphisms for syntactic pattern recognition. Sys-
tems, Man and Cybernetics, IEEE Transactions on,
SMC-13(1):48–62.
Vento, M. (2015). A long trip in the charming world of
graphs for pattern recognition. Pattern Recognition,
48(2):291–301.
Zeng, Z., Tung, A. K. H., Wang, J., Feng, J., and Zhou,
L. (2009). Comparing stars: On approximating graph
edit distance. Proc. VLDB Endow., 2(1):25–36.
ICPRAM2015-InternationalConferenceonPatternRecognitionApplicationsandMethods
278