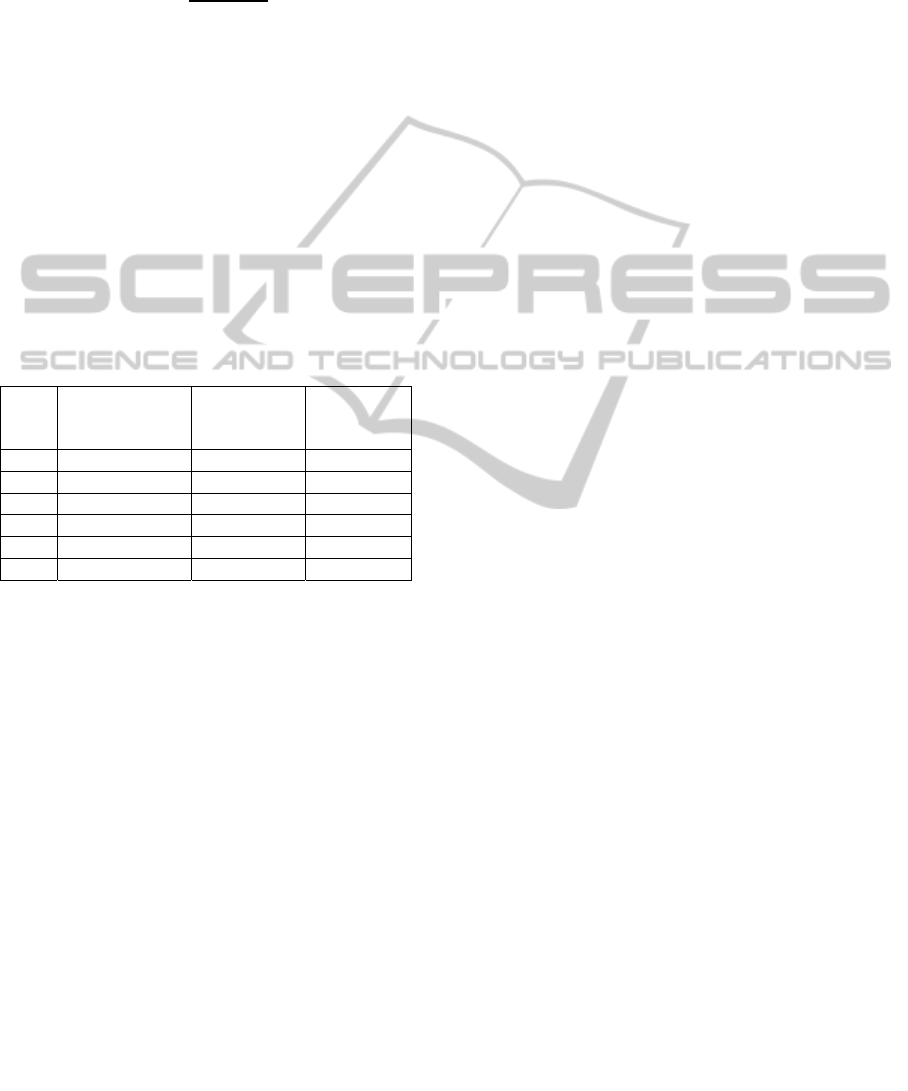
data, represents the wavelet level, and
represents the length of the wavelet coefficients or
raw data. After calculating at each wavelet
level, the ratio of at each level to that of raw
data is calculated as Equation 3.
(3)
The ratios of at wavelet levels 1 - 4,
which correspond to the frequency ranges of the
bolus flowing sound, and those at wavelet levels 5 -
6, which correspond to the frequency ranges of the
throat movement sound, are summed up separately
to describe the characteristics of high frequencies
and low frequencies, as shown in Table 3. The ratios
of in high frequencies to those in low
frequencies on six days are also calculated as the last
column in Table 3. As for the ratio of in high
frequencies to that in low frequencies, a large
increase appears between the second and third days.
Table 3: Ratio of in high frequencies and low
frequencies.
Day
SWLC in high
frequencies
(125 - 2000 Hz)
SWLC in low
frequencies
(32 - 125 Hz)
Ratio of high
to low
1st 17.50 1.09 16.0
2nd 18.47 1.12 16.5
3rd 16.20 0.77 21.1
4th 13.76 0.61 22.6
5th 13.93 0.70 19.9
6th 14.27 0.60 24.0
The ratio of the sum of the absolute values of
wavelet coefficients in the high frequency ranges
(levels 1 - 4) to that in the low frequency ranges
(levels 5 - 6) can be considered as the change in
swallowing sound as a possible reason for the time-
dependent change in low frequency ranges.
4.4 Novel Proposal for Compensation
for Time-dependent Change
On the basis of the acoustic characteristics of
swallowing sounds, it is possible that throat
movement sounds at low frequencies are affected
easily by changes in a person’s physical and mental
conditions such as the state of throat movement. In
comparison, the bolus flowing sound occurs by
bolus flowing through the throat with little influence
from a person’s physical and mental conditions.
Therefore, the bolus flowing sound at high
frequencies is robust to time-dependent change,
while some changes possibly occur in the throat
movement sound over a long period of time.
Use of the bolus flowing sound is proposed to
reduce time-dependent change. Therefore, the
optimal feature selection from wavelet based
features at high frequency levels (levels 1 - 4) can
be regarded as a compensation method for time-
dependent change.
5 CONCLUSIONS
In this research, an analysis method for detecting
drug administration behavior by using swallowing
sound was proposed, and a compensation method for
time-dependent change based on the frequency
characteristics of the sound was also proposed for
long-term use.
In the classification of swallowing activities, a
high classification accuracy of 85.4% was achieved
by using the optimal feature vector from six levels of
statistical features of wavelet coefficients and six
levels of AR-model parameter of wavelet
coefficients as features and an artificial neural
network as a classifier. Due to the large individual
differences, a subject-dependent database is adopted
in the classification.
Generally, time-dependent change can be
ignored in the classification of swallowing activities.
However, for a long-term use, as a compensation
method for time-dependent change, the use of
wavelet based features at high frequency levels is
proposed.
REFERENCES
Sabaté, E. (Ed.), 2003. Adherence to long-term therapies:
evidence for action. World Health Organization.
Y. Tanabe, H. Takahashi, T. Tomii, Y. Iiduka and K.
Yamasue, 2012. Design and an Experimental
Evaluation of Training Data Management Method for
Object-based ADL Recognition, DEIM Forum 2012
C6-1.
Au-Yeung, K. Y., Robertson, T., Hafezi, H., Moon, G.,
DiCarlo, L., Zdeblick, M., and Savage, G, 2010. A
networked system for self-management of drug
therapy and wellness. Wireless Health 2010. ACM,
2010, pages 1-9.
Amft, O., Tröster, G., 2009. On-body sensing solutions for
automatic dietary monitoring. IEEE pervasive
computing. 8(2), pages 62-70.
Klahn, M. S., Perlman, A. L., 1999. Temporal and
durational patterns associating respiration and
swallowing. Dysphagia, 14(3), pages 131-138.
Sazonov, E. S., Makeyev, O., Schuckers, S., Lopez-
Meyer, P., Melanson, E. L., and Neuman, M. R., 2010.
HEALTHINF2015-InternationalConferenceonHealthInformatics
372