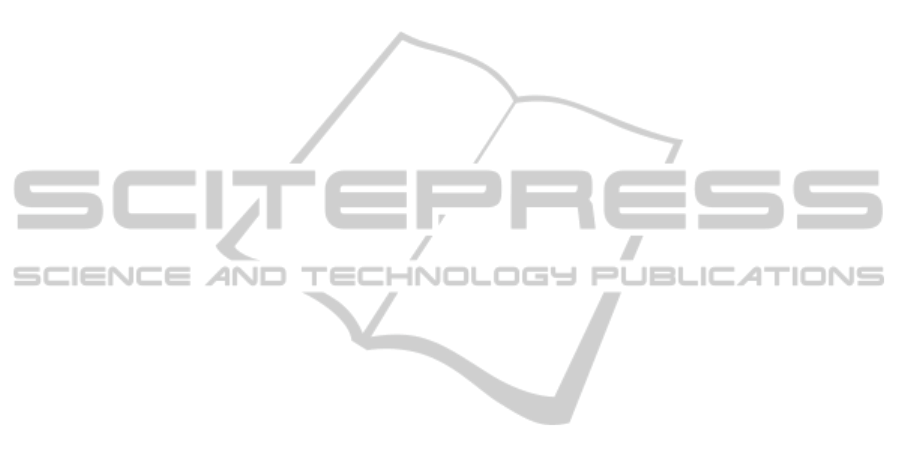
REFERENCES
Babiloni, C., Carducci, F., Cincotti, F., Rossini, P. M., Neu-
per, C., Pfurtscheller, G., and Babiloni, F. (1999). Hu-
man movement-related potentials vs desynchroniza-
tion of EEG alpha rhythm: a high-resolution EEG
study. Neuroimage, 10(6):658–65.
Bai, O., Mari, Z., Vorbach, S., and Hallett, M. (2005).
Asymmetric spatiotemporal patterns of event-related
desynchronization preceding voluntary sequential fin-
ger movements: a high-resolution EEG study. Clin.
Neurophysiol., 116(5):1213–1221.
Bai, O., Rathi, V., Lin, P., and Huang, D. (2011). Prediction
of human voluntary movement before it occurs. Clin.
Neurophysiol., 122(2):364–372.
Blankertz, B., Tomioka, R., Lemm, S., Kawanabe, M., and
M
¨
uller, K.-R. (2008). Optimizing spatial filters for ro-
bust EEG single-trial analysis. IEEE Signal Process-
ing Magazine, pages 41–56.
Cavanagh, P. R. and Komi, P. V. (1979). Electromechanical
delay in human skeletal muscle under concentric and
eccentric contractions. European Journal of Applied
Physiology and Occupational Physiology, 42(3):159–
163.
Chang, C.-C. and Lin, C.-J. (2011). LIBSVM:
A library for support vector machines. ACM
Transactions on Intelligent Systems and Tech-
nology, 2:27:1–27:27. Software available at
http://www.csie.ntu.edu.tw/ cjlin/libsvm.
Deecke, L., Gr
¨
ozinger, B., and Kornhuber, H. H. (1976).
Voluntary finger movement in man: Cerebral poten-
tials and theory. Biol. Cybern., 23(2):99–119.
Folgheraiter, M., Kirchner, E., and Seeland, A. (2011). A
multimodal brain-arm interface for operation of com-
plex robotic systems and upper limb motor recovery.
In BIODEVICES 2011 - International Conference on
Biomedical Electronics and Devices, pages 150–162.
Ibanez, J., Serrano, J. I., del Castillo, M. D., Monge-
Pereira, E., Molina-Rueda, F., Alguacil-Diego, I.,
and Pons, J. L. (2014). Detection of the onset of
upper-limb movements based on the combined anal-
ysis of changes in the sensorimotor rhythms and slow
cortical potentials. Journal of Neural Engineering,
11(5):056009.
Jiang, N., Gizzi, L., Mrachacz-Kersting, N., Dremstrup, K.,
and Farina, D. (2014). A brain-computer interface for
single-trial detection of gait initiation from movement
related cortical potentials. Clin Neurophysiol.
Kirchner, E. A., Albiez, J., Seeland, A., Jordan, M., and
Kirchner, F. (2013a). Towards assistive robotics for
home rehabilitation. In Chimeno, M. F., Sol
´
e-Casals,
J., Fred, A., and Gamboa, H., editors, Proceedings of
the 6th International Conference on Biomedical Elec-
tronics and Devices (BIODEVICES-13), pages 168–
177, Barcelona. ScitePress.
Kirchner, E. A., Kim, S. K., Straube, S., Seeland, A.,
W
¨
ohrle, H., Krell, M. M., Tabie, M., and Fahle, M.
(2013b). On the applicability of brain reading for pre-
dictive human-machine interfaces in robotics. PLoS
ONE, 8(12):e81732.
Krell, M. M., Straube, S., Seeland, A., W
¨
ohrle, H., Teiwes,
J., Metzen, J. H., Kirchner, E. A., and Kirchner, F.
(2013). pySPACE - a signal processing and classifica-
tion environment in Python. Frontiers in Neuroinfor-
matics, 7(40). https://github.com/pyspace.
Leocani, L., Toro, C., Zhuang, P., Gerloff, C., and Hallett,
M. (2001). Event-related desynchronization in reac-
tion time paradigms: a comparison with event-related
potentials and corticospinal excitability. Clin. Neuro-
physiol., 112(5):923–30.
Lew, E., Chavarriaga, R., Silvoni, S., and Mill
´
an, J. D. R.
(2012). Detection of self-paced reaching movement
intention from EEG signals. Front. Neuroeng., 5:13.
Li, Y., Gao, X., Liu, H., and Gao, S. (2004). Classifica-
tion of single-trial electroencephalogram during finger
movement. IEEE Trans. Biomed. Eng., 51(6):1019–
1025.
Liao, X., Yao, D., Wu, D., and Li, C. (2007). Combining
spatial filters for the classification of single-trial EEG
in a finger movement task. IEEE Trans. on Bio-med.
Eng., 54(5):821–31.
Morash, V., Bai, O., Furlani, S., Lin, P., and Hallett, M.
(2008). Classifying EEG signals preceding right hand,
left hand, tongue, and right foot movements and motor
imageries. Clin. Neurophysiol., 119(11):2570–2578.
M
¨
uller-Gerking, J., Pfurtscheller, G., and Flyvbjerg, H.
(1999). Designing optimal spatial filters for single-
trial EEG classification in a movement task. Clin.
Neurophysiol., 110(5):787–798.
Niazi, I. K., Jiang, N., Tiberghien, O., Nielsen, J. r. F. k.,
Dremstrup, K., and Farina, D. (2011). Detection
of movement intention from single-trial movement-
related cortical potentials. J Neural Eng, 8(6).
Nikolic, M. and Krarup, C. (2011). EMGTools, an adaptive
and versatile tool for detailed EMG analysis. Biomed-
ical Engineering, IEEE Transactions on, 58(10):2707
–2718.
Paradiso, G., Cunic, D., Saint-Cyr, J. a., Hoque, T., Lozano,
A. M., Lang, A. E., and Chen, R. (2004). Involve-
ment of human thalamus in the preparation of self-
paced movement. Brain, 127(Pt 12):2717–2731.
Pfurtscheller, G. (1981). Central beta rythm during senso-
rimotor activities in man. Electroencephalogr. Clin.
Neurophysiol., 51(3535):253–264.
Pfurtscheller, G. and Berghold, a. (1989). Patterns of corti-
cal activation during planning of voluntary movement.
Electroencephalogr. Clin. Neurophysiol., 72(3):250–
258.
Pfurtscheller, G. and Lopes da Silva, F. H. (1999). Event-
related EEG/MEG synchronization and desynchro-
nization: basic principles. Clin. Neurophysiol.,
110(11):1842–1857.
Pfurtscheller, G. and Neuper, C. (1992). Simultaneous EEG
10Hz desynchronization and 40 Hz synchronization
during finger movements. Neuroreport, 3:1057–1060.
Pfurtscheller, G., Neuper, C., and Kalcher, J. (1993). 40-Hz
oscillations during motor behavior in man. Neurosci.
Lett., 164(1-2):179–182.
Rivet, B., Souloumiac, A., Attina, V., and Gibert, G. (2009).
xDAWN algorithm to enhance evoked potentials: ap-
Spatio-temporalComparisonbetweenERD/ERSandMRCP-basedMovementPrediction
225