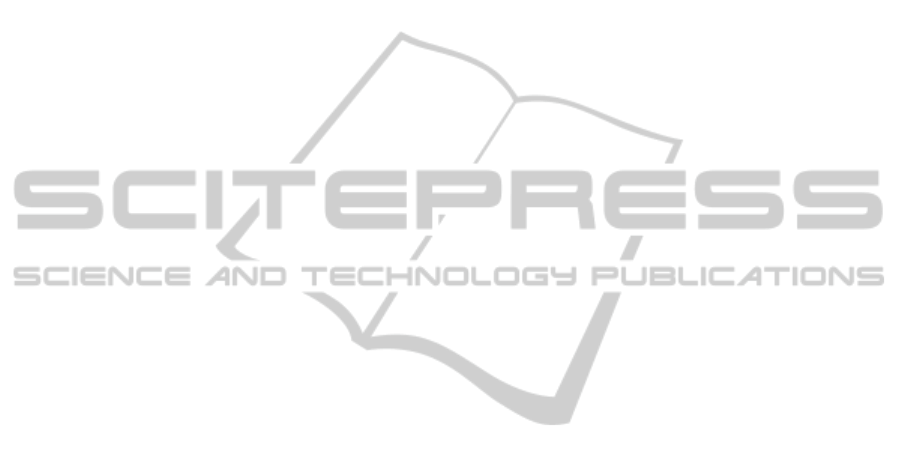
than others. Table1 and Table2 shows the recogni-
tion rate of facial expression using OEPA-PCA and
OEPA-NMF. This result is from the joint dataset of
CK+ and JAFFE. As we achieve good recognition
rate for face recognition using PCA and NMF, we are
not interested to apply OEPA based method for face
recognition.
8 CONCLUSIONS
In this work we propose a multi feature fusion based
algorithm to fuse different combination of facia fea-
ture subspace and to analyze how it improves the
facial expression recognition rate. We name this
method as Optimal Expression Specific Parts Accu-
mulation (OEPA). Here our main work is to imple-
ment OEPA based NMF algorithm and to compare
it with OEPA based PCA. As written before, we
only apply OEPA based approach for facial expres-
sion recognition, not for face recognition. As for
face recognition we achieve a reasonable result using
straight PCA and NMF. Oue result shows OEPA-PCA
and OEPA-NMF outperforms the predominant PCA
and NMF method.
ACKNOWLEDGEMENTS
This work was supported in part by the Chinese Nat-
ural Science Foundation under Grant No. 61070117,
the Beijing Natural Science Foundation under Grant
No. 4122004, and the Australian Research Council
under ARC Thinking Systems Grant No. TS0689874
as well as the Importation and Development of High-
Caliber Talents Project of Beijing Municipal Institu-
tions.
REFERENCES
Ali, H. B. and Powers, D. M. W. (2013). Facial expres-
sion recognition based on weighted all parts accu-
mulation and optimal expression specific parts accu-
mulation. In Digital Image Computing Techniques
and Applications (DICTA),International Conference
on. IEEE,Hobart, Tasmania,Vol.2,No. 1.pp.1-7.
Ali, H. B. and Powers, D. M. W. (2014). Fusion based fas-
tica method: Facial expression recognition. Journal
of Image and Graphics, 2(1):1–7.
C. Hesher, A. S. and Erlebacher, G. (2003). A novel tech-
nique for face recognition using range images. In Sev-
enth Intl Symp. on Signal Processing and Its Applica-
tions.
Charlesworth, W. R. and Kreutzer, M. A. (1973). Facial
expressions of infants and children. In In P. Ekman
(Ed.), Darwin and facial expression: A century of re-
search in review, pages 91–138. New York; Academic
Press.
Cichocki, Andrzej, e. a. (2009). Nonnegative matrix
and tensor factorizations: applications to exploratory
multi-way data analysis and blind source separation.
John Wiley and Sons.
Ekman, P. (1994). Strong evidence for universals in facial
expressions: A reply to russell’s mistaken critique. In
Psychological Bulletin., pages 115(2): 268–287.
Ensari, Tolga, J. C. and Zurada, J. M. (2012). Occluded
face recognition using correntropy-based nonnegative
matrix factorization. In Machine Learning and Appli-
cations (ICMLA),2012 11th International Conference
on IEEE.vol 1.
Frith, U. and Baron-Cohen, S. (1987). Perception in autistic
children. In Advances in Neural Information Process-
ing Systems, pages 85–102. Handbook of autism and
pervasive developmental disorders.New York: John
Wiley.
I. Buciu, N. N. and Pitas, I. (2007). Nonnegative matrix fac-
torization in polynomial feature space. IEEE Transac-
tions on Neural Network, 42:300–311.
J. Buhmann, M. J. L. and Malsburg, C. (1990). Size and
distortion invariant object recognition by hierarchical
graph matching. In Proceedings, International Joint
Conference on Neural Networks, pages 411–416.
L. Zhao, G. Z. and Xu, X. (2008). Facial expression recog-
nition based on pca and nmf. In Proceedings of the 7th
World Congress on Intelligent Control and Automa-
tion, Chongqing, China.
Lee, D. D. and Seung, H. S. (2009). Learning the parts of
objects by non-negative matrix factorization. In Let-
ters to Nature, pages 788–791.
Li, J. and Oussalah, M. (2010). Automatic face emotion
recognition system. In Cybernetic Intelligent Systems
(CIS), 2010 IEEE 9th International Conference.
M. Lades, J. Vorbruggen, J. B. J. L. C. M. R. W. and Konen,
W. (1993). Distortion invariant object recognition in
the dynamic link architecture. IEEE Transaction on
Computing, 42300-311.
Marco, Virgil R., D. M. Y. and Turner., D. W. (1987).
The euclidean distance classifier: an alternative to the
linear discriminant function. In Advances in Neu-
ral Information Processing Systems, pages 485–505.
Communications in Statistics-Simulation and Compu-
tation.
Moghaddam, B. and Pentland, A. (1997). Probabilistic vi-
sual learning for object representation. IEEE Trans-
action on Pattern Analysis and Machine Intelligence,
19:696–710.
Paul, V. and Jones, M. (2001). Rapid object detection using
a boosted cascade of simple features. In Computer
Vision and Pattern Recognition.
Powers, D. M. W. (2003). Recall and precision versus the
bookmaker. In Interna- tional Conference on Cogni-
tive Science (ICSC-2003), page 529534.
Powers, D. M. W. (2011). Evaluation: From precision, re-
call and f-measure to roc., informedness, markedness
FaceandFacialExpressionRecognition-FusionbasedNonNegativeMatrixFactorization
433