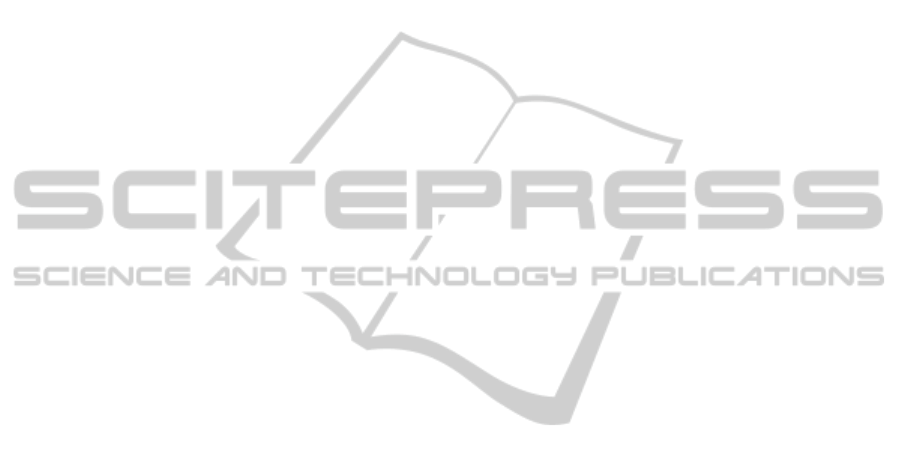
ACKNOWLEDGEMENTS
This work was supported by the European Regional
Development Fund in the IT4Innovations Centre
of Excellence project (CZ.1.05/1.1.00/02.0070)
and EU project Development of Research Ca-
pacities of the Silesian University in Opava
(CZ.1.07/2.3.00/30.0007).
REFERENCES
Atkinson, M. R., Savageau, M. A., Myers, J. T., and Ninfa,
A. J. (2003). Development of genetic circuitry ex-
hibiting toggle switch or oscillatory behavior in Es-
cherichia Coli. Cell, 113(5):597–607.
Balagadd
´
e, F. K., Song, H., Ozaki, J., Collins, C. H., Barnet,
M., Arnold, F. H., Quake, S. R., and You, L. (2008).
A synthetic escherichia coli predator–prey ecosystem.
Molecular systems biology, 4(1).
Barkai, N. and Leibler, S. (2000). Circadian clocks limited
by noise. Nature, 403(6767):267–268.
Becskei, A., Seraphin, B., and Serrano, L. (2001). Positive
feedback in eukaryotic gene networks: cell differen-
tiation by graded to binary response conversion. The
EMBO Journal, 20(10):2528–2535.
Conrad, E., Mayo, A. E., Ninfa, A. J., and Forger, D. B.
(2008). Rate constants rather than biochemical mech-
anism determine behaviour of genetic clocks. Journal
of the Royal Society Interface, 5(supp1):S9–S15.
Danino, T., Mondragon-Palomino, O., Tsimring, L., and
Hasty, J. (2010). A synchronized quorum of genetic
clocks. Nature, 463(7279):326–330.
Elowitz, M. B. and Leibler, S. (2000). A synthetic oscil-
latory network of transcriptional regulators. Nature,
403(6767):335–338.
Ferrell Jr., J. E. (2002). Self-perpetuating states in sig-
nal transduction: positive feedback, double-negative
feedback and bistability. Current Opinion in Cell Bi-
ology, 14(2):140–148.
Franc¸ois, P. (2005). A model for the Neurospora circadian
clock. Biophysical Journal, 88:2369–2383.
Fung, E., Wong, W. W., Suen, J. K., Bulter, T., Lee, S.,
and Liao, J. C. (2005). A synthetic gene-metabolic
oscillator. Nature, 435(7038):118–122.
Gallego, M. and Virshup, D. M. (2007). Post-translational
modifications regulate the ticking of the circadian
clock. Nature Reviews Molecular Cell Biology,
8(2):139–148.
Garcia-Ojalvo, J., Elowitz, M. B., and Strogatz, S. H.
(2004). Modeling a synthetic multicellular clock: re-
pressilators coupled by quorum sensing. Proceedings
of the National Academy of Sciences, 101(30):10955–
10960.
Gardner, T. S., Cantor, C. R., and Collins, J. J. (2000).
Construction of a genetic toggle switch in Escherichia
coli. Nature, 403(6767):339–342.
Gillespie, D. T. (1977). Exact stochastic simulation of
coupled chemical reactions. The Journal of Physical
Chemistry, 81(25):2340–2361.
Guantes, R. and Poyatos, J. F. (2006). Dynamical principles
of two-component genetic oscillators. PLoS Compu-
tational Biology, 2(3):e30.
Hasty, J., Isaacs, F., Dolnik, M., McMillen, D., and Collins,
J. J. (2001). Designer gene networks: Towards funda-
mental cellular control. Chaos, 11(1):207–220.
Hong, C. I., Jolma, I. W., Loros, J. J., Dunlap, J. C., and
Ruoff, P. (2008). Simulating dark expressions and in-
teractions of frq and wc-1 in the Neurospora circadian
clock. Biophysical Journal, 94(4):1221–1232.
Keller, A. D. (1995). Model genetic circuits encoding au-
toregulatory transcription factors. Journal of Theoret-
ical Biology, 172(2):169–185.
Kim, J. and Winfree, E. (2011). Synthetic in vitro transcrip-
tional oscillators. Molecular Systems Biology, 7.
Krishna, S., Semsey, S., and Jensen, M. H. (2009). Frus-
trated bistability as a means to engineer oscillations in
biological systems. Physical Biology, 6(3):036009.
Lenz, P. and Søgaard-Andersen, L. (2011). Temporal and
spatial oscillations in bacteria. Nature Reviews Micro-
biology, 9(8):565–577.
McMillen, D., Kopell, N., Hasty, J., and Collins, J. (2002).
Synchronizing genetic relaxation oscillators by inter-
cell signaling. Proceedings of the National Academy
of Sciences, 99(2):679–684.
Mir
´
o-Bueno, J. M. and Rodr
´
ıguez-Pat
´
on, A. (2011). A sim-
ple negative interaction in the positive transcriptional
feedback of a single gene is sufficient to produce reli-
able oscillations. PLoS ONE, 6(11):e27414.
Mitrophanov, A. Y. and Groisman, E. A. (2008). Posi-
tive feedback in cellular control systems. BioEssays,
30(6):542–555.
Mohawk, J. A., Green, C. B., and Takahashi, J. S. (2012).
Central and peripheral circadian clocks in mammals.
Annual review of neuroscience, 35:445.
Mondrag
´
on-Palomino, O., Danino, T., Selimkhanov, J.,
Tsimring, L., and Hasty, J. (2011). Entrainment of
a population of synthetic genetic oscillators. Science,
333(6047):1315–1319.
Montagne, K., Plasson, R., Sakai, Y., Fujii, T., and Ron-
delez, Y. (2011). Programming an in vitro DNA oscil-
lator using a molecular networking strategy. Molecu-
lar Systems Biology, 7.
Munteanu, A., Constante, M., Isalan, M., and Sole, R.
(2010). Avoiding transcription factor competition at
promoter level increases the chances of obtaining os-
cillation. BMC Systems Biology, 4(1):66.
Nandi, A., Vaz, C., Bhattacharya, A., and Ramaswamy, R.
(2009). miRNA-regulated dynamics in circadian os-
cillator models. BMC Systems Biology, 3(1):45.
Ng, W.-L. and Bassler, B. L. (2009). Bacterial quorum-
sensing network architectures. Annual review of ge-
netics, 43:197–222.
Nov
´
ak, B. and Tyson, J. J. (2008). Design principles of bio-
chemical oscillators. Nature Reviews Molecular Cell
Biology, 9(12):981–991.
BIOINFORMATICS2015-InternationalConferenceonBioinformaticsModels,MethodsandAlgorithms
126