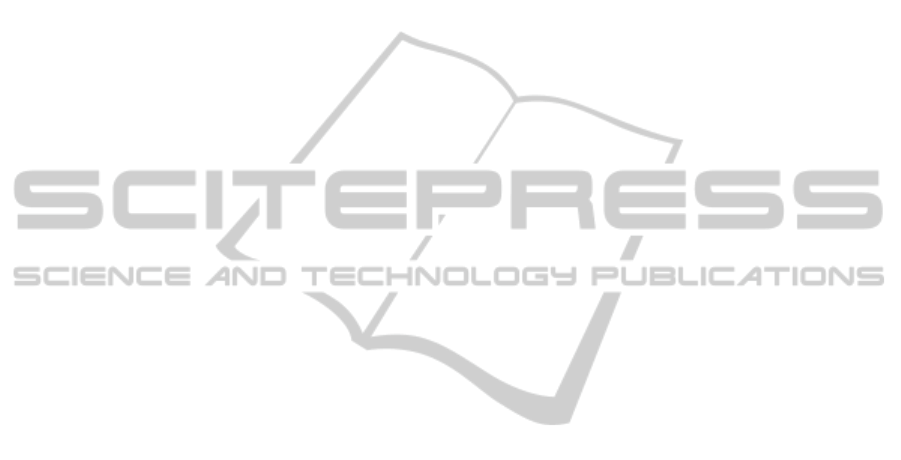
generally lower than 50%, which translate to a high
count of missed positives for the respective
threshold values.
The results generally show improved or
comparable diagnostic performance achieved using
the proposed, automatic method in comparison to
the human-based evaluation. It is foreseeable that
better and more intelligent classification methods
making use of all signal properties (and not just the
extracted segmentation metric S) could lead to
definitively better performance than human reading.
Such improved detection can not only lead to better
detection but can also improve the therapy
monitoring utility of optical imaging by reducing
operator dependency. Such improved classification
is being currently researched. Furthermore, semi-
quantitative scoring of synovitis using optical
images as well as further development of the
proposed method in conjunction with larger cohorts
are subjects of ongoing work.
REFERENCES
Backhaus, M., Kamradt, T., Sandrock, D., Loreck, D.,
Fritz, J., Wolf, K. J., Raber, H., Hamm, B., Burmester,
G. R. & Bollow, M. 1999. Arthritis Of The Finger
Joints: A Comprehensive Approach Comparing
Conventional Radiography, Scintigraphy, Ultrasound,
And Contrast-Enhanced Magnetic Resonance
Imaging. Arthritis Rheum, 42, 1232-45.
Chen, W. T., Mahmood, U., Weissleder, R. & Tung, C. H.
2005. Arthritis Imaging Using A Near-Infrared
Fluorescence Folate-Targeted Probe. Arthritis
Research & Therapy, 7, R310-R317.
Delle Sedie, A., Riente, L. & Bombardieri, S. 2008. Limits
And Perspectives Of Ultrasound In The Diagnosis
And Management Of Rheumatic Diseases. Modern
Rheumatology, 18, 125-131.
Emery, P. & Quinn, M. A. 2003. Window Of Opportunity
In Early Rheumatoid Arthritis: Possibility Of Altering
The Disease Process With Early Intervention. Clinical
And Experimental Rheumatology, 21, S154-S157.
Emery, P., Wakefield, R. J., O'connor, P. J., Conaghan, P.
G., Mcgonagle, D., Hensor, E. M. A., Gibbon, W. W.
& Brown, C. 2007. Finger Tendon Disease In
Untreated Early Rheumatoid Arthritis: A Comparison
Of Ultrasound And Magnetic Resonance Imaging.
Arthritis & Rheumatism-Arthritis Care & Research,
57, 1158-1164.
Fawcett, T. 2006. An Introduction To Roc Analysis.
Pattern Recognition Letters, 27, 861–874.
Fischer, T., Ebert, B., Voigt, J., Macdonald, R., Schneider,
U., Thomas, A., Hamm, B. & Hermann, K. G. 2010.
Detection Of Rheumatoid Arthritis Using Non-
Specific Contrast Enhanced Fluorescence Imaging.
Acad Radiol, 17, 375-81.
Gompels, L. L., Lim, N. H., Vincent, T. & Paleolog, E. M.
2010. In Vivo Optical Imaging In Arthritis--An
Enlightening Future? Rheumatology (Oxford), 49,
1436-46.
Hielscher, A. H., Kim, H. K., Montejo, L. D., Blaschke,
S., Netz, U. J., Zwaka, P. A., Illing, G., Muller, G. A.
& Beuthan, J. 2011. Frequency-Domain Optical
Tomographic Imaging Of Arthritic Finger Joints. Ieee
Trans Med Imaging, 30, 1725-36.
Hielscher, A. H., Klose, A. D., Scheel, A. K., Moa-
Anderson, B., Backhaus, M., Netz, U. & Beuthan, J.
2004. Sagittal Laser Optical Tomography For Imaging
Of Rheumatoid Finger Joints. Physics In Medicine
And Biology, 49, 1147-1163.
Huttenlocher, D. P., Klanderman, G. A. & Rucklidge, W.
J. 1993. Comparing Images Using The Hausdorff
Distance. Ieee Transactions On Pattern Analysis And
Machine Intelligence, 15, 850-863.
Jolliffe, I. T. 2002. Principal Component Analysis,
Springer.
Klose, A. D., Hielscher, A. H., Hanson, K. M. & Beuthan,
J. 1999. Two- And Three-Dimensional Optical
Tomography Of Finger Joints For Diagnostics Of
Rheumatoid Arthritis. Proceedings Of Spie, 3566,
151-160.
Meier, R., Thuermel, K., Moog, P., Noel, P., Ahari, C.,
Sievert, M., Dorn, F., Waldt, S., Schaeffeler, C.,
Werner, S., Golovko, D., Haller, B., Ganter, C.,
Weckbach, S., Woertler, K. & Rummeny, E. J. 2012.
Detection Of Arthritis In The Hands Of Patients With
Rheumatological Disorders: Diagnostic Performance
Of Optical Imaging In Comparison To Magnetic
Resonance Imaging. Arthritis & Rheumatism, 64,
2489-98.
Meier, R., Thuermel, K., Noël, P. B., Moog, P., Sievert,
M., Ahari, C., Nasirudin, R. A., Golovko, D., Haller,
B., Ganter, C., Wildgruber, M., Schaeffeler, C., Waldt,
S. & Rummeny, E. J. 2014. Synovitis In Patients With
Early Inflammatory Arthritis Monitored With
Quantitative Analysis Of Dynamic Contrast-Enhanced
Optical Imaging And Mr Imaging. Radiology, 270,
176-185.
Michael Levandowsky & Winter, D. 1971. Distances
Between Sets. Nature, 234, 34-35.
Mohajerani, P., Koch, M., Thürmel, K., Haller, B.,
Rummeny, E. J., Ntziachristos, V. & Meier, R. 2014.
Fluorescence-Aided Tomographic Imaging Of
Synovitis In The Human Finger. Radiology.
Mohajerani, P., Meier, R., Noël, P. B., Rummeny, E. J. &
Ntziachristos, V. 2013. Spatiotemporal Analysis For
Indocyanine Green-Aided Imaging Of Rheumatoid
Arthritis In Hand Joints. Journal Of Biomedical
Optics, 18, 097004-097004.
Ostergaard, M., Ejbjerg, B. & Szkudlarek, M. 2005.
Imaging In Early Rheumatoid Arthritis: Roles Of
Magnetic Resonance Imaging, Ultrasonography,
Conventional Radiography And Computed
Tomography. Best Practice & Research In Clinical
Rheumatology, 19, 91-116.
BIOIMAGING2015-InternationalConferenceonBioimaging
42