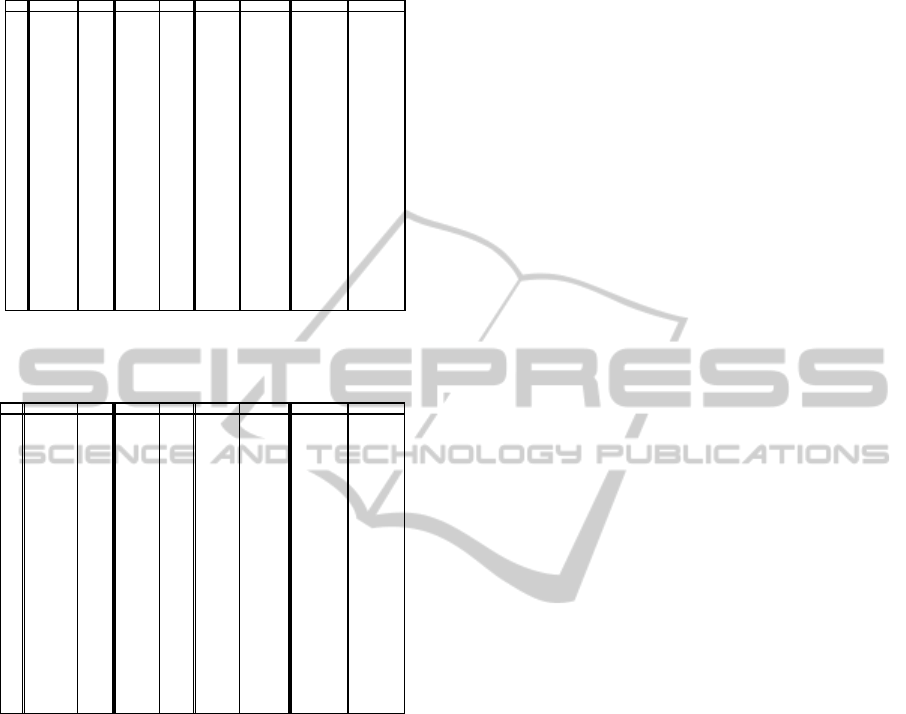
Table 2: Comparing the VNS algorithm with the tree and
star topology approaches for L = 5Mbps.
|V| P
1
cpu P
1
P
2
cpu P
2
TVNS cpu TVNS Gap
Ini
TV NS
(%) Gap
TVNS
(%)
4 162.7889 0.15 181.7955 0.10 162.7889 0.28 11.68 0.00
6 130.7177 0.13 237.5767 0.09 130.7177 4.89 81.75 0.00
8 138.2804 0.46 253.2526 0.11 138.2804 27.48 83.14 0.00
10 204.3663 48.08 239.1874 0.11 204.3663 17.71 17.04 0.00
12 161.4248 162.49 232.9846 0.11 161.4248 74.87 44.33 0.00
14 109.1878 536.17 242.8569 0.13 173.1598 300 122.42 58.59
16 125.7083 625.16 251.7890 0.13 175.4427 300 100.30 39.56
18 104.7611 3600 246.8710 0.13 169.0028 300 135.65 61.32
20 136.3206 3600 220.1141 0.12 157.5897 300 61.47 15.60
22 132.9221 3600 241.1231 0.13 205.2670 300 81.40 54.43
24 238.3949 3600 255.3129 0.13 238.3949 2.11 7.10 0.00
26 243.4611 3600 252.3117 0.12 243.4611 1.78 3.64 0.00
28 182.2605 3600 234.3619 0.12 212.9248 300 28.59 16.82
30 243.6324 3600 247.7179 0.13 243.6324 3.25 1.68 0.00
32 4054.8263 3600 255.4642 0.14 226.9286 300 < 0 < 0
34 1736.2377 3600 245.4668 0.16 242.9136 300 < 0 < 0
36 6688.2599 3600 253.5267 0.16 249.3354 300 < 0 < 0
38 5507.4285 3600 251.5471 0.33 231.1319 300 < 0 < 0
40 4404.3266 3600 252.7837 0.16 248.3448 300 < 0 < 0
42 3997.2096 3600 254.4504 0.19 244.3861 300 < 0 < 0
44 409.0830 3600 247.5290 0.17 247.5290 300 < 0 < 0
46 - 3600 253.1534 0.17 250.1164 300 - -
48 - 3600 255.8555 0.17 255.8555 300 - -
50 4383.5935 3600 251.9520 0.19 249.4831 300 < 0 < 0
52 - 3600 254.4080 0.17 249.7041 300 - -
54 3970.1243 3600 254.5704 0.19 245.4474 300 < 0 < 0
56 - 3600 254.4691 0.20 254.4691 300 - -
58 - 3600 244.6565 0.23 244.6565 300 - -
60 - 3600 255.9535 0.34 249.3236 300 - -
-: No solution found.
< 0: Negative gap.
Table 3: Comparing the VNS algorithm with the tree and
star topology approaches for L = 10Mbps.
|V| P
1
cpu P
1
P
2
cpu P
2
TVNS cpu TVNS Gap
Ini
TVNS
(%) Gap
TV NS
(%)
4 224.9149 0.11 252.7684 0.11 224.9149 1.16 12.38 0.00
6 157.9910 0.13 249.5453 0.08 157.9910 6.47 57.95 0.00
8 122.0700 0.86 223.3105 0.11 140.3444 300 82.94 14.97
10 86.0596 4.26 245.3015 0.13 91.4057 300 185.04 6.21
12 154.3654 219.71 235.0383 0.13 156.7696 300 52.26 1.56
14 228.2618 569.32 253.9770 0.13 239.0206 300 11.27 4.71
16 96.0876 511.14 236.5652 0.13 156.4360 300 146.20 62.81
18 148.2626 3600 244.0291 0.31 174.1759 300 64.59 17.48
20 212.1160 3600 254.9278 0.11 212.1160 50.25 20.18 0.00
22 276.3054 3600 244.1628 0.17 218.1632 300 < 0 < 0
24 412.0215 3600 249.2979 0.14 245.7859 300 < 0 < 0
26 4005.5097 3600 249.7173 0.31 209.0481 300 < 0 < 0
28 340.8418 3600 236.4095 0.14 155.4772 300 < 0 < 0
30 3220.0005 3600 250.7632 0.34 223.3664 300 < 0 < 0
32 3501.5596 3600 252.3360 0.14 236.5049 300 < 0 < 0
34 2926.9978 3600 254.4703 0.14 251.3070 300 < 0 < 0
36 4534.1685 3600 251.3342 0.16 223.2100 300 < 0 < 0
38 6013.2241 3600 253.5718 0.19 248.8768 300 < 0 < 0
40 4641.5615 3600 244.5667 0.14 244.5667 300 < 0 < 0
42 5388.5247 3600 255.5524 0.16 237.7339 300 < 0 < 0
44 4038.1242 3600 245.8233 0.19 228.4913 300 < 0 < 0
46 8611.3871 3600 252.3020 0.36 245.9307 300 < 0 < 0
48 5434.4153 3600 243.5083 0.37 240.8507 300 < 0 < 0
50 4998.2235 3600 254.3199 0.19 254.3199 300 < 0 < 0
52 8623.4855 3600 253.8108 0.19 248.0597 300 < 0 < 0
54 6383.7045 3600 255.2982 0.36 252.4182 300 < 0 < 0
56 10061.0697 3600 255.8105 0.39 255.8105 300 < 0 < 0
58 8297.8535 3600 254.9113 0.39 254.9113 300 < 0 < 0
60 4660.1790 3600 254.1911 0.22 254.1911 300 < 0 < 0
-: No solution found.
< 0: Negative gap.
less than one hour. On the opposite, for instances with
more than 28 nodes in Table 2 and with more than 22
nodes in Table 3, the solutions obtained with P
1
in one
hour are significantly deteriorated since solving these
instances with CPLEX becomes rapidly prohibitive.
Next, we observe that the cpu time required to solve
P
2
is less than one second for all the instances in Ta-
bles 2 and 3, respectively. Finally, we see that the ma-
jor improvements for the VNS approach occur when
solving small and medium size instances with up to 40
nodes. The latter suggests that the star configuration
is not a bad choice when the instances dimensions in-
crease. We believe that VNS can not find significantly
better solutions for large size instances of the prob-
lem because there are more infeasible solutions in the
WBAN when the number of nodes increase. The in-
feasibility can be explained by the fact that having a
larger number of nodes in the network implies send-
ing a larger amount of data through the network, and
then the edge capacities are rapidly saturated. Ob-
viously, this can be fixed by incrementing the edge
capacities in the network.
5.1 A Flooding Network Scenario
We also consider the case where all nodes can be di-
rectly connected to more than one node acting as a
star node. For this purpose, we relax the condition
imposed for the parameter P = 1 in P
2
and allow it
to vary from P = 1 to P = |V|. Notice that when
P = |V|, it means that all nodes in the network are
fully connected. In this case, the optimal solutions of
P
2
are equal to those obtained with LP
2
.
From a practical point of view, this situation
would provide some insight about how many nodes
acting as stars are required to obtain a minimum cost
energy consumption in the network. In Figure 2, we
solve four instances of P
2
with different number of
nodes while varying P . The horizontal axes show
the parameter P while vertical axes show the optimal
objective function values of P
2
and LP
2
, respectively.
From this figure, we mainly observe that the optimal
solutions of P
2
decrease rapidly when incrementing P
which means that very low energy consumption levels
can be obtained at the cost of low flooding levels as
well.
6 CONCLUSIONS
In this paper, we proposed a minmax robust formula-
tion for routing in healthcare wireless body area net-
works (WBAN). The model minimizes the worst case
power consumption of each bio-sensor node placed
in the body of a patient subject to flow rate and net-
work topology constraints. So far we considered three
topologies in the problem: a spanning tree, a star,
and a ring topology as well. In particular, we used
an equivalent polynomial formulation of the spanning
tree polytope (Yannakakis, 1991) to avoid having an
exponential number of cycle elimination constraints
in the model. For the ring topology approach, we
used constraints from the well known mixed integer
linear programming(MILP) formulation of the travel-
ing salesman problem (Pataki, 2003). Thus, we com-
puted optimal solutions and lower bounds directly us-
ing the MILP and LP relaxations. Finally, we pro-
posed a Kruskal-based variable neighborhood search
metaheuristic to improve the solutions obtained with
the star topology approach. Our preliminary numeri-
cal results showed that the tree approach is the most
convenient while the ring approach is the most expen-
sive one. We also noticed that the difference between
AComparativeStudyofNetwork-basedApproachesforRoutinginHealthcareWirelessBodyAreaNetworks
131