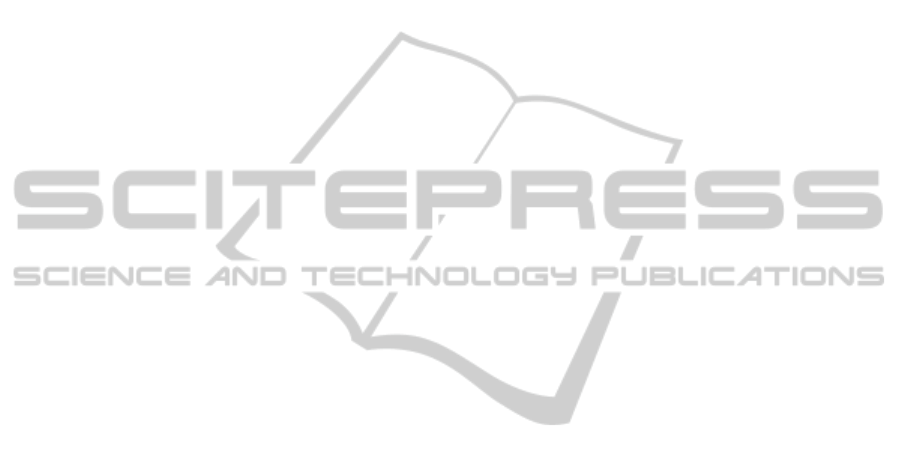
expensive black box optimization where a single eval-
uation of the objective function can easily take many
hours or even days and/or cost a considerable amount
of money (Hole
ˇ
na et al., 2008).
In the case of two-dimensional problems, the
MGSO performs far better than CMA-ES on
quadratic sphere and Rosenbrock function. The re-
sults on Rastrigin function are comparable, although
with greater variance (see Fig. 2: the descent of the
medians is slightly slower within the first 200 func-
tion evaluations, but faster thereafter). The Tomlab’s
implementation of EGO performs almost equally well
as the MGSO on sphere function, but on Rosenbrock
and Rastrigin, the convergence of EGO is extremely
slowed down after few iterations, which can be seen in
5D and 10D, too. The positive effect of ARD covari-
ance function can be seen quite clearly, especially on
Rosenbrock function. The difference between ARD
and non-ARD results are hardly to see on sphere func-
tion, probably because its symmetry means no im-
provement in ARD covariance employment.
The performance of the MGSO on five-
dimensional problems is similar to 2D cases.
The MGSO descends notably faster on non-rugged
sphere and Rosenbrock functions, especially if we
concentrate on depicted cases with a very low num-
ber of objective function evaluations (up to 250 · D
evaluations). The drawbacks of the MGSO is shown
on 5D Rastrigin function where it is outperformed
by CMA-ES, especially between ca. 200 and 1200
function evaluations.
Results of optimization in the case of ten-
dimensional problems show that the MGSO works
better than CMA-ES only on the most smooth sphere
function which is very easy to regress by Gaussian
process model. On more complicated benchmarks,
the MGSO is outperformed by CMA-ES.
The graphs on Fig. 2 show that the MGSO is
usually slightly slower than EGO in the very first
phases of the optimization, but EGO quickly stops its
progress and does not descent further. This is exactly
what can be expected from the MGSO in comparison
to EGO – sampling the PoI instead of searching for
the maximum can easily overcome situations where
EGO gets stuck in a local optimum.
5 CONCLUSIONS AND FUTURE
WORK
The MGSO, the optimization algorithm based on a
Gaussian process model and the sampling of the prob-
ability of improvement, is intended to be used in the
field of expensive black-box optimization. This pa-
per summarizes its properties and evaluates its perfor-
mance on several benchmark problems. Comparison
with Gaussian-process based EGO algorithm shows
that the MGSO is able to easily escape from local
optima. Further, it has been shown that the MGSO
can outperform state-of-the-art continuous black-box
optimization algorithm CMA-ES in low dimension-
alities or on very smooth functions. On the other
hand, CMA-ES performs better on rugged or high-
dimensional benchmarks.
ACKNOWLEDGEMENTS
This work was supported by the Czech Science Foun-
dation (GA
ˇ
CR) grants P202/10/1333 and 13-17187S.
REFERENCES
Bajer, L., Hole
ˇ
na, M., and Charypar, V. (2013). Improv-
ing the model guided sampling optimization by model
search and slice sampling. In Vinar, T. e. a., editor,
ITAT 2013 – Workshops, Posters, and Tutorials, pages
86–91. CreateSpace Indp. Publ. Platform.
Buche, D., Schraudolph, N., and Koumoutsakos, P. (2005).
Accelerating evolutionary algorithms with gaussian
process fitness function models. IEEE Transactions
on Systems, Man, and Cybernetics, Part C: Applica-
tions and Reviews, 35(2):183–194.
Hansen, N., Finck, S., Ros, R., and Auger, A. (2009).
Real-parameter black-box optimization benchmark-
ing 2009: Noiseless functions definitions. Technical
Report RR-6829, INRIA. Updated February 2010.
Hansen, N. and Ostermeier, A. (2001). Completely deran-
domized self-adaptation in evolution strategies. Evo-
lutionary Computation, 9(2):159–195.
Hole
ˇ
na, M., Cukic, T., Rodemerck, U., and Linke, D.
(2008). Optimization of catalysts using specific, de-
scription based genetic algorithms. Journal of Chem-
ical Information and Modeling, 48:274–282.
Jin, Y. (2005). A comprehensive survey of fitness approxi-
mation in evolutionary computation. Soft Computing,
9(1):3–12.
Jones, D. R. (2001). A taxonomy of global optimiza-
tion methods based on response surfaces. Journal of
Global Optimization, 21(4):345–383.
Jones, D. R., Schonlau, M., and Welch, W. J. (1998).
Efficient global optimization of expensive black-
box functions. Journal of Global Optimization,
13(4):455–492.
Larrañaga, P. and Lozano, J. A. (2002). Estimation of distri-
bution algorithms: A new tool for evolutionary com-
putation. Kluwer.
Rasmussen, C. E. and Williams, C. K. I. (2006). Gaussian
Processes for Machine Learning. Adaptative compu-
tation and machine learning series. MIT Press.
ICAART2015-InternationalConferenceonAgentsandArtificialIntelligence
456