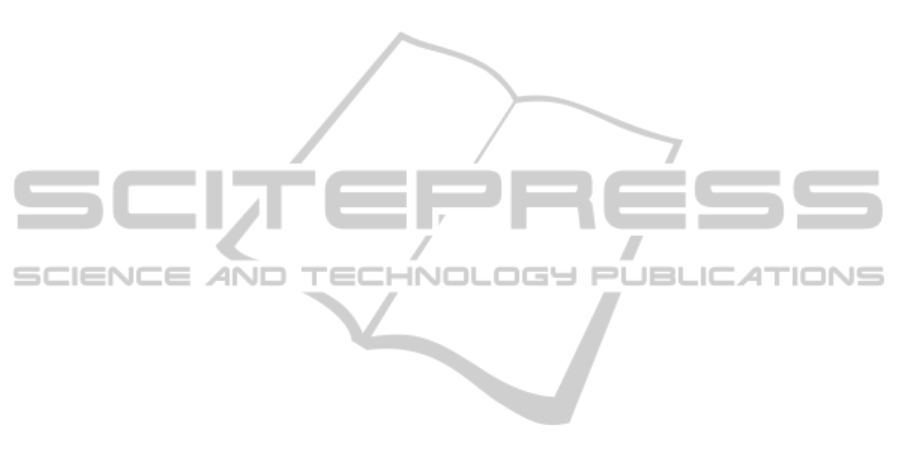
fit between healthcare service and mHealth tool
characteristics from a matching perspective, the
study findings can better inform mHealth tool use by
CHWs and enhance performance in their capture,
storage, transmission, and retrieval of health data
(Liu et al., 2011). In the areas of Information
Dependency and Interdependence, ‘Fit as Matching’
provides the best explanations for performance
outcomes. However, findings also indicate that just
because CHWs have needs does not mean that a
highly functional tool necessarily results in
increased dependency on Use or enhanced User
Performance. Similarly, just because CHWs do not
recognize a need, does not mean a high functioning
tool cannot influence their dependence on Use or
enhanced User Performance. The tool could be
compensating for those who have not recognized a
need and therefore have not already established
routines and coping mechanisms. However, for
those who have recognized a need, the tool may be
unimportant given already established preferred
practices. The study confirms that mobile
technologies could improve mHealth tool use and
CHW performance in low-resource community
household settings (Earth Institute, 2010). However,
designers should be cautious of excessive functional
support that may hinder CHW performance with
established routines, and that despite high mobility
and time criticality needs, an mHealth tool may not
always provide the best support. If function support
is excessive, users may depend less on the tool, and
its impacts may not be favourable at all levels of
need. These results can nevertheless add to the
growing interest in directly supporting CHWs at the
point of care. Future research may wish to consider
cost implications as instrumental to the successful
deployment of mHealth platforms in the Kenyan
context. Future work may also consider assessing
the match between CHW needs and mHealth tool
functions in other contexts and settings.
ACKNOWLEDGEMENTS
We sincerely thank Kenya’s Ministry of Health
(MOH) Division of Community Health Services
(DCHS) and all Community Health Workers
(CHWs) who participated in the study.
REFERENCES
Dishaw, M. T. and Strong, D. M. (1998) Supporting
Software Maintenance With Software Engineering
Tools: A Computed Task- Technology Fit Analysis.
The Journal of Systems and Software, 44, 107-
120.
Earth Institute, “One Million Community Health Workers:
Technical Taskforce Report”, 2010, http://
www.milleniumvillages.org/uploads/ReportPaper/1m
CHW_Technical TaskForceReport.pdf [last accessed
30
th
August 2014].
Torkzadeh, G. and Doll, W. 1999. The Development of a
Tool for Measuring the Perceived Impact of
Information Technology on Work. The International
Journal of Management Science, 27, 1, 327-339.
Gatara, M., and Cohen, J. F., 2014a. Mobile-Health Tool
Use and Community Health Worker Performance in
the Kenyan Context: A Task-Technology Fit
Perspective. In Proceedings of the South African
Institute for Computer Scientists and Information
Technologists SAICSIT Conference (Centurion,
Gauteng, South Africa, September 28 – October 01
2014).
Gatara, M., and Cohen, J. F., 2014b. The Mediating Effect
of Task-Technology Fit on mHealth Tool Use and
Community Health Worker Performance in the
Kenyan Context. In Proceedings of the 8th
International Development Informatics Association
IDIA Conference (Port Elizabeth, South Africa,
November 03-04, 2014).
Gebauer, J. and Tang, Y. 2008. Applying the Theory of
Task-Technology Fit to Mobile Technology: The
Role of User Mobility. Working Paper Abstract, 1-23.
Goodhue, D. L. and Thompson, R. L. 1995. Task-
Technology Fit and Individual Performance. MIS
Quarterly 19, 2, 213-236.
Gupta, S. 2003. Knowledge Management and
Performance: A Fit Perspective. In Proceedings of the
Ninth Americas Conference on Information Systems
(Tampa, Florida, USA, August 04-06, 2003).
Hair, J. F, Hult, G. T, Ringle, C. M. and Sarstedt, M. 2014.
A Primer on Partial Least Squares Structural
Equation Modeling (PLS-SEM), Sage, Thousand
Oaks, California.
Junglas, I., Abraham, C., and Ives, B. 2009. Mobile
Technology at the Frontlines of Patient Care:
Understanding Fit and Human Drives in Utilization
Decisions and Performance, Decision Support
Systems. 46, 534- 647.
LeMaire, J. “Scaling Up Mobile Health: Elements for the
Successful Scale Up of mHealth in Developing
Countries”, 2011 http://www.k4health.org/sites/sites/
default/files/ADA_mHealth%20White%20Paper.pdf
[last accessed 2
nd
May 2013].
Lin, T. and Huang, C. 2008. Understanding Knowledge
Management System Usage Antecedents: An
Integration of Social Cognitive Theory and Task-
Technology Fit. Information and Management, 45,
410-417.
Liu, A, Sullivan, S, Khan, M. Sachs, G. and Singh, P.
2011. Community Health Workers in Global Health:
Scale and Scalability, Mount Sinai Journal of
Medicine, 78, 1, 419-435.
HEALTHINF2015-InternationalConferenceonHealthInformatics
460