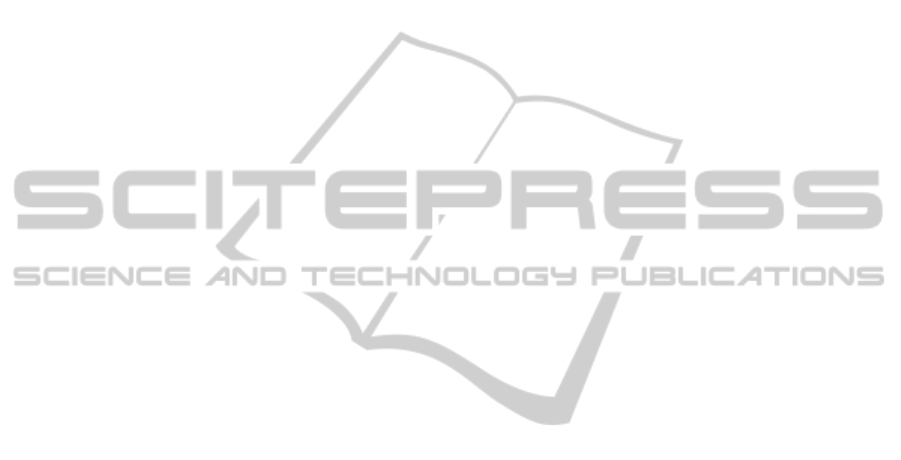
learning environment. The experimental results
showed that the GSR sensors’ measurement was
aligned with the surveys, so that we can use GSR
sensors as an alternative method to measure
students’ engagement. Furthermore, we compared
the resulted non-engaging user experiences to the
previous similar studies, and we found that GSR
readings demonstrated the different patterns that
have not been reported before. In addition, the MDS
result revealed that the GSR response at the remote
location was more synchronized when compared to
the one at the lecture classroom.
We believe that our study is beneficial for E-
learning, as we have shown that GSR sensors can be
used as an alternative tool to provide feedback in a
distributed learning environment. Moreover, we
presented a non-engaging user case, where the
patterns of GSR readings were first reported. Last,
the methodologies incorporated in this study are also
helpful on other sensor studies, e.g., a pulse sensor.
REFERENCES
Daniel D. Garcia and Luke Segars. 2012. Technology that
educators of computing hail (TECH): come, share
your favorites!. In Proceedings of the 43rd ACM
technical symposium on Computer Science.
Education (SIGCSE '12). ACM, New York, NY, USA,
682-682. DOI=10.1145/2157136.2157438.
Erkollar, Alptekin, and B. J. Oberer. "Putting Google+ to
the Test: Assessing Outcomes for Student
Collaboration, Engagement and Success in Higher
Education." Procedia-Social and Behavioral Sciences
83 (2013): 185-189.
Strudler, Neal, and Karen Grove. "I see you: Using the
affordances of Google+ to increase social and teaching
presence in an online undergraduate teacher education
course ISTE 2013." Cynthia Clark doctoral student,
San Antonio, TX. Retrieved from http://www.
isteconferenceorg/uploads/ISTE2013/HANDOUTS/
KEY_80520342/I STE2013ISeeYou_RP. pdf. Accessed
on June 17 (2013): 2013.
Chen Wang, Erik N. Geelhoed, Phil P. Stenton, and Pablo
Cesar. 2014. Sensing a live audience. In Proceedings
of the 32nd annual ACM conference on Human factors
in computing systems (CHI '14). ACM, New York,
NY, USA, 1909-1912.
DOI=10.1145/2556288.2557154.
Young, Forrest W., and Robert M. Hamer (ed.)(1987),
Multidimensional Scaling: History,Theory, and
Applications, Hillsdale, NJ: Erlbaum.
Schiffman, Susan S., M. Lance Reynolds, and Forrest W.
Young (1981), Introduction to Multidimensional
Scaling: Theory, Methods, and Applications, NY:
Academic Press.
Trevor F. Cox and M.A.A. Cox (2000). Multidimensional
Scaling, Second Edition. ISBN 1-58488-094-5.
Ingwer Borg and Patrick J.F. Groenen. Modern
Multidimensional Scaling: Theory and Applications.
2005 Springer Science+Buiness Media, Inc. ISBN-10:
0-387-25150-2.
Foertsch, J., Moses, G., Strikwerda, J. and Litzkow, M.
(2002), Reversing the Lecture/Homework Paradigm
Using eTEACH® Web-based Streaming Video
Software. Journal of Engineering Education, 91: 267–
274. doi: 10.1002/j.2168-9830.2002.tb00703.x.
Mavlankar, A; Agrawal, P.; Pang, D.; Halawa, S.; Ngai-
Man Cheung; Girod, B., "An interactive region-of-
interest video streaming system for online lecture
viewing," Packet Video Workshop (PV), 2010 18th
International , vol., no., pp.64,71, 13-14 Dec. 2010
doi: 10.1109/PV.2010.5706821.
Cha Zhang, Yong Rui, Jim Crawford, and Li-Wei He.
2008. An automated end-to-end lecture capture and
broadcasting system. ACM Trans. Multimedia.
Comput. Commun. Appl. 4, 1, Article 6 (February.
2008), 23 pages. DOI=10.1145/1324287.1324293.
Kathryn Faulkner and Linda McClelland. (2002). Using
videoconferencing to deliver a healthy education
program to women healthy consumers in rural and
remote queensland: an early attempt and future plans.
Aust. J. Rural Health 10, 65-72.
Dmochowski, Jacek P, Bezdek, Matthew A, Abelson,
Brian P, Johnson, John S, Schumacher, Eric H, Parra,
Lucas C. 2014. Audience preferences are predicted by
temporal reliability of neural processing. Nature
Publishing Group, a division of Macmillan Publishers
Limited. http://dx.doi.org/10.1038/ncomms5567.10.
1038/ncomms5567.
Chin-Yeh Wang, Gwo-Dong Chen, Chen-Chung Liu, and
Baw-Jhiune Liu. 2009. Design an empathic virtual
human to encourage and persuade learners in e-
learning systems. InProceedings of the first ACM
international workshop on Multimedia technologies
for distance learning
(MTDL '09). ACM, New York,
NY, USA, 27-32. DOI=10.1145/1631111.1631117.
Cristina Hava Muntean and Gabriel-Miro Muntean. 2009.
Open corpus architecture for personalised ubiquitous.
e-learning. Personal Ubiquitous Comput. 13, 3 (March
2009), 197-205. DOI=10.1007/s00779-007-0189-5.
Thomas Wirtky, Sven Laumer, Andreas Eckhardt, and
Tim Weitzel. 2013. Using social software for
enhancing IS talents' e-learning motivation. In
Proceedings of the 2013 annual conference on
Computers and people research (SIGMIS-CPR '13).
ACM, New York, NY, USA, 63-72.
DOI=10.1145/2487294.2487307.
E. Aljenaa, F. S. Al-Anzi, and M. Alshayeji. 2011.
Towards an efficient e-learning system based on cloud
computing. In Proceedings of the Second Kuwait
Conference on e-Services and e-Systems(KCESS '11).
ACM, New York, NY, USA, Article 13, 7 pages.
DOI=10.1145/2107556.2107569.
Vladimir Kolovski and John Galletly. 2003. Towards E-
learning via the semantic web. InProceedings of the
4th international conference conference on Computer
systems and technologies: e-Learning (CompSysTech
PhysiologicalMeasurementonStudents'EngagementinaDistributedLearningEnvironment
155