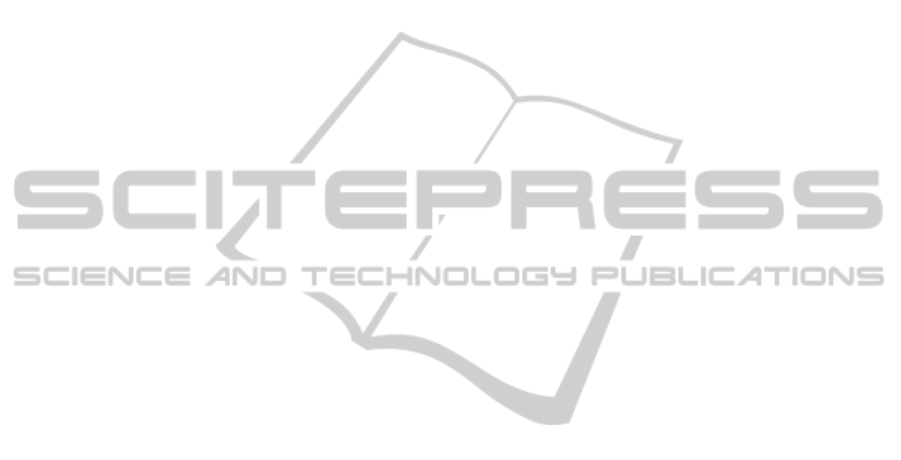
2
i
,
2
s
is monotone increasing as absolute
value of
12
increases.
2
ationR
varies around 1, and its monotone
decreasing as
12
increases is not significant. This
situation indicates that
12
is not strongly related to
the amplification of bullwhip effect going up the
supply chain.
12
has little impact on bullwhip
effect.
(2) Under centralized information, when
ii
>0:
Bullwhip effect of retailer/supplier is monotone
increasing as
12
increases, and the amplification is
significant.
When
12
>0,
1
ationR
2
centr s
M /
2
centr i
M >1,and
1
ationR
is monotone increasing as
12
increases. It
means that the amplification of variance of order in
supplier stage is larger than that in retailer stage,
and this difference increases with the value of
12
.
This situation indicates that larger
12
will increase
the amplification of variance of order quantity
spreading to the upstream supply chain.
When
12
<0,
1
ationR
<1 , and
1
ationR
is
monotone increasing as
12
increases. It means
that the amplification of variance of order in
supplier stage is smaller than that in retailer stage,
and this difference decreases as
12
increases. The
impact of number of stages of supply chain on
bullwhip effect is not effected by
12
.
ij
has little impact on bullwhip effect.
(3) When
ii
<0,
12
and
12
both have little
impact on bullwhip effect.
7.2 Management Insights
To sum up, what we should pay attention to are as
following:
(1) When retailers’ demands are positive
correlated, no matter under centralized or
decentralized information, this correlation has
significant impact on retailers’/supplier’s bullwhip
effect.
(2) Under decentralized information, both
retailers’ and supplier’s bullwhip effect increases as
the absolute value of retailers’ demand correlation
increases, and bullwhip effect in supplier stage and
retailer stage are almost the same.
(3) Under centralized information, when
retailers’ demands are positive correlated, both
retailers’ and supplier’s bullwhip effect increases as
retailers’ demand correlation increases, and
bullwhip effect level in supplier stage is larger than
that in retailer level. It indicates that under
centralized information the impact of number of
supply chain stages on bullwhip effect is related
with the retailers’ demand correlation.
(4) Under centralized information, when and
only when retailers’ demands are negative
correlated (
0
ij
), the supplier’s bullwhip effect
will be less than retailers’. It indicates that under
centralized information supplier’s demand forecast
become more accurate as the result of retailers’
competition.
Hence, when retailers’ demands are correlated,
besides the well-known causes of bullwhip effect
(such as lead time, number of supply chain stages),
any member in the supply chain should consider the
impact of multi-terminals’ demand correlation on
bullwhip effect when making production plan.
Furthermore, under centralized information, when
retailers’ demand are positive correlated, the
bullwhip effect in supplier stage is higher than that
in retailers’ stage; on the contrary, under centralized
information, when retailers’ demand are negative
correlated, the bullwhip effect in supplier stage is
lower than that in retailers’ stage. These conclusions
provide theoretical reference about bullwhip caused
by terminals’ demand correlation for enterprises to
make production plan.
ACKNOWLEDGMENTS
This research is supported in part by National
Science Foundation of China Grants (70732003).
REFERENCES
Chen, F., Drezner, Z., Ryan, J.K., and Simchi-Levi,
D. (2000a), ‘Quantifying the bullwhip effect in a
simple supply chain’
, Management Science, 46 ,
436–443.
Chen, F., Ryan, J.K., and Simchi –Levi, D. (2000),
‘The impact of exponential smoothing forecasts
on the bullwhip effect’,
Naval Research
Logistics
, 47, 269–286.
Heyman, D.P. and Sobel, M.J. (2003), Stochastic
Models in Operations Research: Stochastic
Optimization (Vol.2),
New York: Courier Dover
Publications.
Johnson, G.D. and Thompson, H.E. (1975),
Optimality of myopic inventory policies for
certain dependent demand processes,
Management Science, 21, 1303–1307.
ICORES2015-InternationalConferenceonOperationsResearchandEnterpriseSystems
312