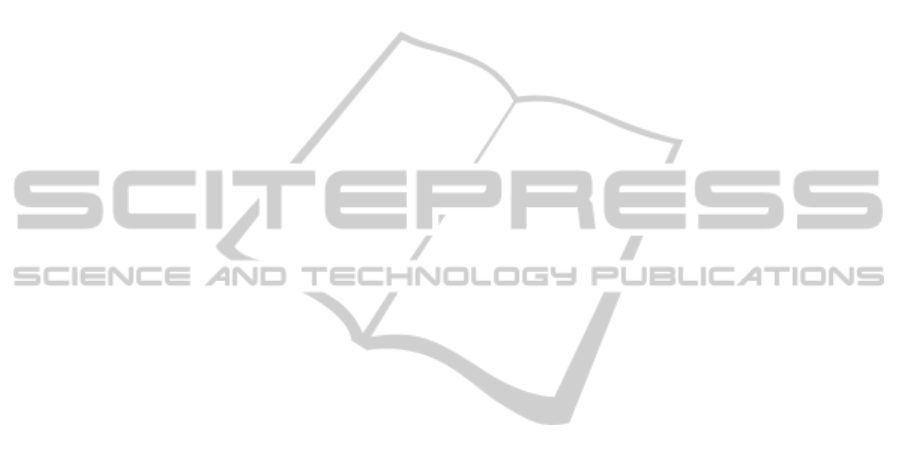
mation for epidemiological data (Klemm et al., 2014).
Outlook. Combining the power of statistical
analyses, visual analytics and classification tech-
niques is essential for analyzing increasingly complex
heterogenous population data. These methods do
not aim to replace the traditional epidemiological
workflow, but rather complement the weak points of
standard statistical methods. Our method provides
a novel way to gain insight into these complex data
sets and amplifies hypotheses generation.
ACKNOWLEDGEMENTS
SHIP is part of the Community Medicine Research net of
the University of Greifswald, Germany, which is funded
by the Federal Ministry of Education and Research (grant
no. 03ZIK012), the Ministry of Cultural Affairs as well as
the Social Ministry of the Federal State of Mecklenburg-
West Pomerania. Whole-body MR imaging was supported
by a joint grant from Siemens Healthcare, Erlangen, Ger-
many and the Federal State of Mecklenburg-Vorpommern.
The University of Greifswald is a member of the Centre of
Knowledge Interchange program of the Siemens AG. This
work was supported by the DFG Priority Program 1335:
Scalable Visual Analytics. This work was supported by the
federal state of Saxony-Anhalt under grant number ’I 60’
within the Forschungscampus STIMULATE.
REFERENCES
Deng, H., Runger, G., and Tuv, E. (2011). Bias of im-
portance measures for multi-valued attributes and so-
lutions. In Artificial Neural Networks and Machine
Learning–ICANN 2011, pages 293–300. Springer.
Emerson, J. W., Green, W. A., Schloerke, B., Crowley, J.,
Cook, D., Hofmann, H., and Wickham, H. (2013).
The generalized pairs plot. Journal of Computational
and Graphical Statistics, 22(1):79–91.
Fletcher, R. H., Fletcher, S. W., and Fletcher, G. S. (2012).
Clinical epidemiology: the essentials. Lippincott
Williams & Wilkins.
Glaßer, S., Niemann, U., Preim, B., and Spiliopoulou, M.
(2013). Can we Distinguish Between Benign and Ma-
lignant Breast Tumors in DCE-MRI by Studying a Tu-
mors Most Suspect Region Only? In Proc. of Sympo-
sium on Computer-Based Medical Systems (CBMS),
pages 59–64.
Hegenscheid, K., Seipel, R., Schmidt, C. O., V¨olzke, H.,
K¨uhn, J.-P., Biffar, R., Kroemer, H. K., Hosten, N.,
and Puls, R. (2013). Potentially relevant incidental
findings on research whole-body MRI in the general
adult population: frequencies and management. Eu-
ropean Radiology, 23(3):816–826.
Hoy, D., Brooks, P., Blyth, F., and Buchbinder, R. (2010).
The epidemiology of low back pain. Best Practice and
Research Clinical Rheumatology, 24(6):769 – 781.
Keim, D. A., Mansmann, F., Schneidewind, J., Thomas, J.,
and Ziegler, H. (2008). Visual analytics: Scope and
challenges. Springer.
Klemm, P., Lawonn, K., Rak, M., Preim, B., T¨onnies, K.,
Hegenscheid, K., V¨olzke, H., and Oeltze, S. (2013).
Visualization and Analysis of Lumbar Spine Canal
Variability in Cohort Study Data. In Proc. of Vision,
Modeling, Visualization 2013, pages 121–128.
Klemm, P., Oeltze, S., Lawonn, K., Hegenscheid, K.,
V¨olzke, H., and Preim, B. (2014). Interactive vi-
sual analysis of image-centric cohort study data.
IEEE Trans. on Visualization and Computer Graph-
ics, 20(12):1673–1682.
Mitchell, T. M. (1997). Machine learning. 1997. Burr
Ridge, IL: McGraw Hill, 45.
Niemann, U., V¨olzke, H., K¨uhn, J.-P., and Spiliopoulou,
M. (2014). Learning and inspecting classification
rules from longitudinal epidemiological data to iden-
tify predictive features on hepatic steatosis. Expert
Systems with Applications.
Preim, B., Klemm, P., Hauser, H., Hegenscheid, K., Oeltze,
S., Toennies, K., and V¨olzke, H. (2015). Visual Ana-
lytics of Image-Centric Cohort Studies in Epidemiol-
ogy, chapter Visualization in Medicine and Life Sci-
ences III, page in print. Springer.
Rak, M., Engel, K., and Toennies, K. (2013). Closed-form
hierarchical finite element models for part-based ob-
ject detection. In Proc. of Vision, Modeling, Visual-
ization 2013, pages 137–144.
Szpalski, M., Gunzburg, R., M´elot, C., and Aebi, M. (2005).
The aging of the population: a growing concern for
spine care in the twenty-first century. In The Aging
Spine, pages 1–3. Springer.
Turkay, C., Lundervold, A., Lundervold, A. J., and Hauser,
H. (2013). Hypothesis generation by interactive vi-
sual exploration of heterogeneous medical data. In
Human-Computer Interaction and Knowledge Dis-
covery in Complex, Unstructured, Big Data, pages 1–
12. Springer.
V¨olzke, H., Alte, D., Schmidt, C., et al. (2011). Cohort Pro-
file: The Study of Health in Pomerania. International
Journal of Epidemiology, 40(2):294–307.
Zhang, Z., Gotz, D., and Perer, A. (2012). Interactive vi-
sual patient cohort analysis. In Proc. of IEEE VisWeek
Workshop on Visual Analytics in Health Care.
IVAPP2015-InternationalConferenceonInformationVisualizationTheoryandApplications
92