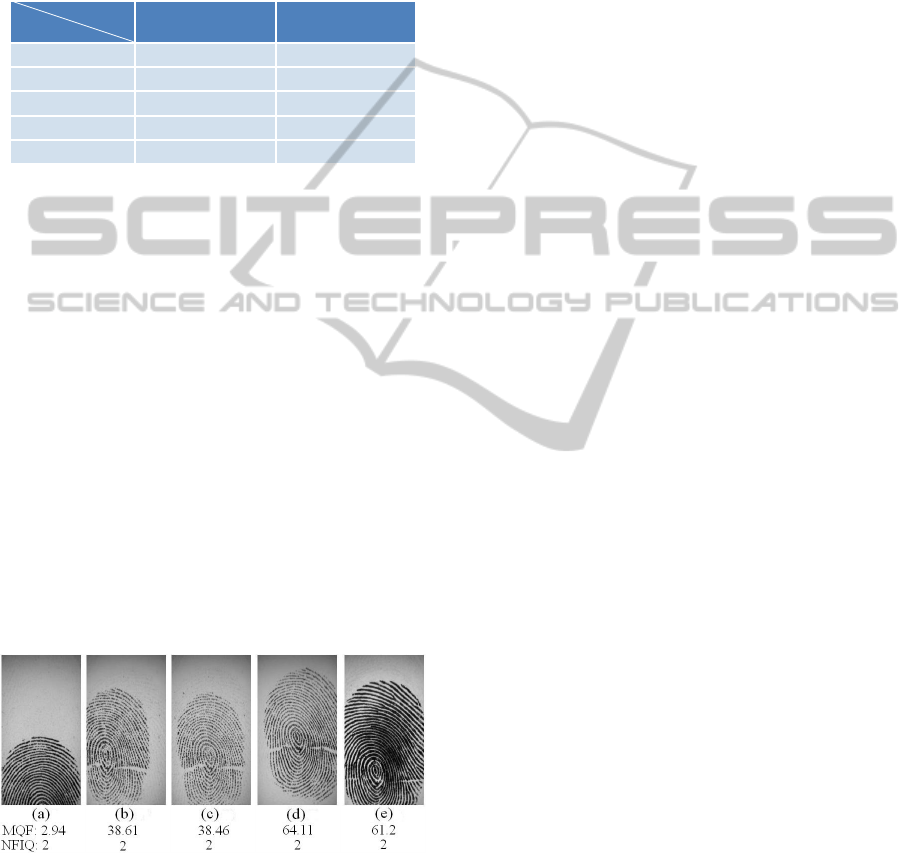
samples after enrollment selection. The size of each
kind of randomly selected matching scores is 500, and
hence the 95% CI is obtained with 1000 samples of
the EER values, i.e. this random selection has been
performed for 1000 iterations to calculate EER sam-
ples. Table 4 provides the average EER values of the
other 3 databases.
Table 5: The 95% confidence interval of the EERs.
DB
QM
MQF NFIQ
00DB2A (CI) [0.0498 0.0510] [0.0492 0.0502]
02DB2A (CI) [0.1101 0.1118] [0.1323 0.1337]
04DB1A (CI) [0.1493 0.1509] [0.1531 0.1548]
04DB2A (CI) [0.1509 0.1535] [0.1318 0.1340]
04DB3A (CI) [0.0683 0.0694] [0.0742 0.0754]
In table 5, the CI of the EER values based on the
two quality metrics statistically illustrates the validity
of the proposed quality metric. The overlap happened
to FVC2000DB2A, for the EER values obtained from
this database is nearly the same. Moreover, we sim-
ply choose several images to illustrate the differences
between the two quality metrics and their drawbacks.
For instance, the MQF values of the samples given in
figure 6 are (2.94, 38.61, 38.46, 64.11, 61.2), and
their NFIQ values are all level 2. According to sam-
ple (a), one can find that it contains only a partial of
the fingerprint image which is not suitable for match-
ing. In addition, the samples with MQF values under
40 are not as good as a level 2 sample in this exam-
ple. The samples (b) and (c) would result in spurious
minutiae. On the other hand, an obvious shortage of
MQF is the area measurement which would generate
outliers in many cases, such as the samples illustrated
by figure 6 (d) and (e).
Figure 6: Illustration of the different between MQF and
NFIQ.
The experiments are implemented via Matlab
7.12. The computation takes approximately 0.423
seconds per sample according to a calculation of 1600
samples when the input is image, and 0.122 seconds
for template inputs.
4 CONCLUSION
This study mainly focuses on estimating fingerprint
quality simply with a minutiae template. By investi-
gating the relation between image-based quality crite-
ria and the structure of minutiae template, we calcu-
late the area of minutiae region via a convex hull and
the Delaunay triangulation. The uniqueness of Delau-
nay triangulation provides a possibility that some un-
reasonable minutiae could be further eliminated from
template, and the particular rules for calculating con-
vex hull enables estimating a relatively minimum area
of the detected minutiae. Accordingly, we define
a simple yet efficient quality metric for fingerprint
and minutiae template. In another aspect, the quality
metric could be affected by some bad samples with
large minutiae region. The lack of image informa-
tion makes this problem inevitable. Correspondingly,
we analyzed the proposed quality metric via Pearson
correlation coefficients and evaluated its performance
with an enrollment phase approach. Therefore, it is
reasonable to conclude that the proposed quality met-
ric is an effective measurement for assessing the qual-
ity of a various of fingerprint samples when their as-
sociated templates are available only.
As fingerprint quality metric is generally defined
with multiple features, this quality metric therefore
could be a candidate for those composite metrics. The
future work of this study tends to concentrate on com-
bining this quality metric with some others extracted
from minutiae template to obtain better performance.
REFERENCES
Alonso-Fernandez, F., Fierrez, J., Ortega-Garcia, J.,
Gonzalez-Rodriguez, J., Fronthaler, H., Kollreider,
K., and Bigun, J. (2007). A comparative study of
fingerprint image-quality estimation methods. Infor-
mation Forensics and Security, IEEE Transactions on,
2(4):734–743.
Andrew, A. M. (1979). Another efficient algorithm for con-
vex hulls in two dimensions. Information Processing
Letters, 9(5):216–219.
Aufmann, R., Barker, V., and Nation, R. (2007). College
Trigonometry. Cengage Learning.
Bolle, R. M., Pankanti, S. U., and Yao, Y.-S. (1999). System
and method for determining the quality of fingerprint
images. US Patent 5,963,656.
Chen, T., Jiang, X., and Yau, W. (2004). Fingerprint image
quality analysis. In Image Processing, 2004. ICIP ’04.
2004 International Conference on, volume 2, pages
1253–1256 Vol.2.
Delaunay, B. (1934). Sur la sphere vide. Izv. Akad.
Nauk SSSR, Otdelenie Matematicheskii i Estestven-
nyka Nauk, 7(793-800):1–2.
ICISSP2015-1stInternationalConferenceonInformationSystemsSecurityandPrivacy
320