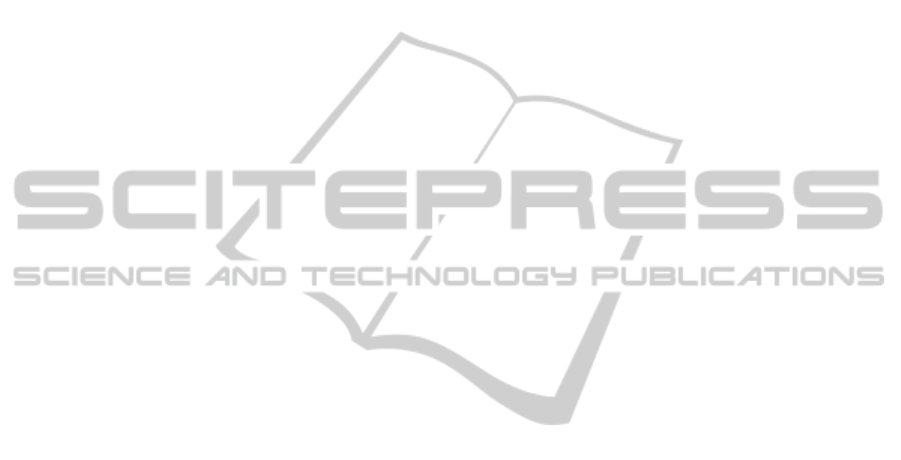
the aim to get closer to real-life implementation, a
thorough ecological task battery setup should be
designed to better ascertain workload modulation in
work settings.
REFERENCES
Allison, B. Z., and Polich, J. (2008). Workload assessment
of computer gaming using a single stimulus event-
related potential paradigm. Biological Psychology,
77(3), pp. 277-283.
Berka, C., Levendowski, D. J., Lumicao, M. N., Yau, A.,
Davis, G., Zivkovic, V. T., Olmstead, R. E., et al.
(2007). EEG correlates of task engagement and mental
workload in vigilance, learning, and memory tasks.
Aviation, Space, and Environmental Medicine, 78,
231-244.
Blankertz, B., Tangermann, M., Vidaurre, C., Fazli, S.,
Sannelli, C., Haufe, S., Maeder, C., Ramsey, L.,
Sturm, I., Curio, G., and Müller, K.-R.. (2010). The
Berlin Brain–Computer Interface: Non-Medical Uses
of BCI Technology. Frontiers in Neuroscience, vol. 4.
Brouwer, A.-M., Hogervorst, M. A. , van Erp, J. B. F.,
Heffelaar, T., Zimmerman, P. H. and Oostenveld, R.
(2012). Estimating workload using EEG spectral
power and ERPs in the n-back task. Journal of Neural.
Enineering, 9(4).
Cain, B. (2007). A review of the mental workload
literature. Defense Research and Development,
Toronto, Canada, [Online]. Available:
http://ftp.rta.nato.int/public//PubFullText/RTO/TR/RT
O-TR-HFM-121-PART-II///TR-HFM-121-Part-II-
04.pdf.
Chen, D. and Vertegaal, R.(2004). Using mental load for
managing interruptions in physiologically attentive
user interfaces. In Human factors in computing
systems, pp. 1513-1516.
van Erp,, J., Lotte, F., and Tangermann, M. (2012). Brain-
Computer-Interfaces: Beyond Medical Applications.
Computer, vol. 45, pp.26-34.
Filipe, S., Charvet, G., Foerster, M., Porcherot, J., Bêche,
J.-F., Bonnet, S., Audebert, P., Régis, G., Zongo, B.,
Robinet, S., Condemine, C., Mestais, C., Guillemaud,
R. (2011). A wireless multichannel EEG recording
platform. In Conf. Proc. of the IEEE Eng. in Med. and
Biol. Soc., Boston, Massachusetts USA, pp. 6319-
6322.
Gevins, A., and Smith, M. E. (2003). Neurophysiological
measures of cognitive workload during human-
computer interaction. Theoretical Issues in
Ergonomics Science, 1, 113-131.
Gevins, A., and Smith, M. E. (2007).
Electroencephalography (EEG) in Neuroergnomics. In
Neuroergonomics: The brain at work, R. Parasuraman,
R and M. Rizzo Eds. New York, NY: Oxford.
Gomarus, H. K., Althaus, M., Wijers, A. A., and
Minderaa, R. B. (2006). The effects of memory load
and stimulus relevance on the EEG during a visual
selective memory search task: An ERP and ERD/ERS
study. Clinical Neurophysiology, 117, 871-884.
Halgren, E., Marinkovic, K., and Chauvel, P. (1998).
Generators of the late cognitive potentials in auditory
and visual oddball tasks. Electroencephalography and
clinical Neurophysiology. 106, pp. 156-164.
Holm, A., Lukander, K., Korpela, J., Sallinen, M. and
Müller, K. M. I. (2009). Estimating Brain Load from
the EEG. TheScientificWorldJOURNAL, vol. 9, pp.
639-651.
Kok, A. (2001). On the utility of P3 amplitude as a
measure of processing capacity. Psychophysiology, 38,
557–577.
Miller, M. W., Rietschel, J. C., McDonald, C. G., and
Hatfield, B. D. (2011). A novel approach to the
physiological measurement of mental workload.
International Journal of Psychophysiology, 80, 75-78.
Näätänen, R. Gaillard, A. W. K., and Varey, C. A. (1981).
Attention effects on auditory EPs as a function of
inter-stimulus interval. Biological Psychology, 13, pp.
173-187.
Natani, K., and Gomer, F. E. (1981). Electrocortical
activity and operator workload: A comparison of
changes in the electroencephalogram and in event-
related potentials. (McDonnell Douglas Technical
Report E2427). Long Beach, CA: McDonnell Douglas
Corporation.
Roy, R. N., Bonnet, S., Charbonnier, S. and Campagne, A.
(2013). Mental fatigue and working memory load
estimation: Interaction and implications for EEG-
based passive BCI. In Conf. Proc. of the IEEE Eng.
Med. Biol. Soc., Osaka, Japan, pp. 6607-6610.
Schultheis, H. and Jameson, A. (2004) Assessing
Cognitive Load in Adaptive Hypermedia Systems:
Physiological and Behavioral Methods. Lecture Notes
in Computer Science, 313, 225-234.
Smith, M. E., Halgren, E., Sokolik, M., Baudena, P.,
Musolino, A., Liegeois-Chauvel, C., and Chauvel, P.
(1990). The intracranial topography of the P3 event-
related potential elicited during auditory oddball.
Electroencephalography and clinical neuro-
physiology, 76, pp. 235-248.
Squires, N. K., Squires, K. C., and Hillyard, S. A. (1975).
Two varieties of long-latency positive waves evoked
by unpredictable auditory stimuli in man.
Electroencephalography and clinical neuro-
physiology, 38, pp. 987-401.
Sternberg, S. (1966). High-speed scanning in human
memory. Science, vol. 153, pp. 652-654.
Strobel, A., Debener, S., Sorger, B., Peters, J. C.,
Kranczioch, C., Hoechstetter, K., Engel, A. K.,
Brocke, B., and Goebel, R. (2008). Novelty and target
processing during an auditory novelty oddball: A
simultaneous event-related potential and functional
magnetic resonance imaging study. NeuroImage, 40,
869-883.
Sugimoto, F. and Katayama, J. (2013). Somatosensory P2
reflects resource allocation in a game task: assessment
with an irrelevant probe technique using electrical
probe stimuli to shoulders. International Journal of
PhyCS2015-2ndInternationalConferenceonPhysiologicalComputingSystems
110