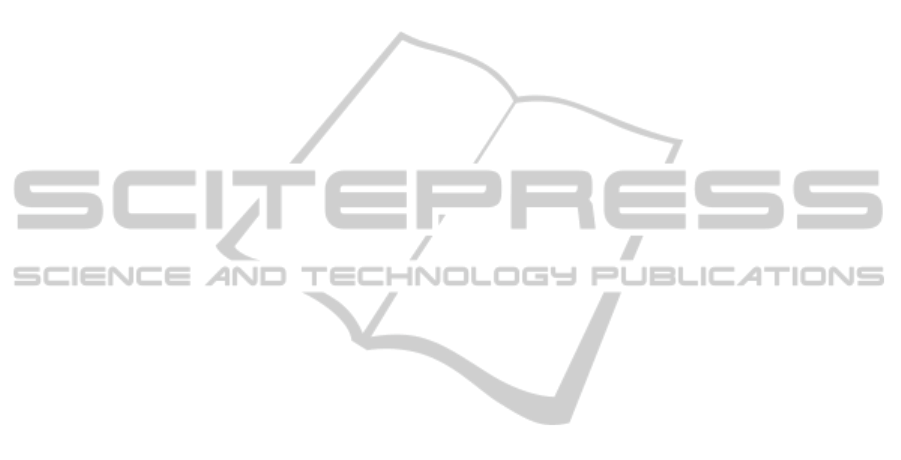
impaired mental state (Miller, 2013). These errors
and accidents assume particular importance when we
consider the domain of high-risk jobs that involve op-
erating vehicles as well as the military, firefighters or
medical personal, just to name a few. Beyond these
immediate problems, fatigue can also lead to health
problems in the long term, such as chronic fatigue
syndrome or depression.
The negative effects of fatigue are thus clear.
Moreover, they are also broad in the sense that affect
many of our cognitive abilities. Learning is one of
the functions that becomes impaired when under fa-
tigue. Hence the importance of addressing this issue,
especially in a time in which the teacher and student
are growing apart due to the increasing use of elec-
tronic tools for learning. Indeed, due to the separation
imposed by technology, it results more and more dif-
ficult for teachers to be sensible to the state of their
students, impairing their ability to adapt both the con-
tents and the teaching strategy accordingly.
This work details a tool for fatigue management
in e-learning scenarios, with or without the teacher’s
presence, through the assessment of mental work-
load quantified in terms of the interaction patterns of
the user with the computer. Through the use of be-
havioural biometrics, specifically Keystroke Dynam-
ics and Mouse Dynamics, we analyse the type of task
performed by each user, the time spent performing it,
as well as the mental workload of the task. With this
information we train classifiers that are able to distin-
guish situations in which users show signs of fatigue
or high mental workload.
This approach can be considered both non-
invasive and non-intrusive, since it is based solely on
the observation of the use of the mouse and keyboard,
which allow for an assessment of the user’s perfor-
mance. This approach opens the door to the develop-
ment of fatigue management initiatives in the context
of e-learning, allowing teachers to not only have a bet-
ter notion about their student’s state but also to more
efficiently adapt and above all personalize teaching
strategies.
1.1 The Need for Monitoring in the
Context of e-Learning
Electronic instruction, more commonly known as e-
learning, is increasingly used as a method of teach-
ing. E-learning differs from classroom-based train-
ing in several ways. Thus, the transition from a tradi-
tional course to a course supported by e-learning can
be complex and difficult. There is the need for a good
course planning and an increased effort in monitoring
and controlling all participants in all the different mo-
ments of the course while at the same time focusing
on getting feedback that may allow to better steer the
course (Hamburg et al., 2008).
Without the obligatory physical presence of a
teacher, the process of e-learning is exposed to some
deficiencies that may result in poor student learning.
Specifically, the teacher is not able to observe the stu-
dents in search of signs evidencing problems such as
doubts, frustration, stress or fatigue, preventing teach-
ers from taking action in such scenarios. The set-
ting up of appropriate monitoring mechanisms in the
context of e-learning is therefore very important in
achieving an efficient learning process.
As shown in the literature, for an efficient mon-
itoring of the student to take place, it is crucial that
the e-learning system allows for a personalized study
strategy and is able to show the needs and strengths
of each student (Cantoni et al., 2004). Thus it be-
comes possible to track the progress of students, as
well as improving their learning, by providing better
personalized learning methods. The identification of
learning problems and the cause of those problems is
another advantage that can be achieved through the e-
learning context, and via monitoring systems, such as
the tool proposed in this paper.
1.2 Including Subjective Measures of
Workload
In this paper we look at the monitoring and managing
of mental workload as a way to improve the quality
of information of the e-learning environment, espe-
cially to improve the teacher’s decision making abili-
ties. One of the important parts of this work is a previ-
ously developed approach, deemed non-invasive and
non-intrusive, for the analysis of the students’ inter-
action patterns.
Indeed, it was established in preliminary work that
one’s patterns of interaction with the computer, mea-
sured in terms of the use of the keyboard and mouse,
change when under fatigue as well as in periods of in-
creased mental workload or even stress. Moreover, it
were also found behavioral differences in performing
different kinds of tasks, allowing to analyze patterns
of attention in the students who participated in the
experimental studies (Pimenta et al., 2015; Pimenta
et al., 2014).
However, the work developed so far has the short-
coming of not considering mental workload, which is
an important aspect when it comes to determining the
actual level of fatigue. It is also important, for ex-
ample, to distinguish between scenarios of boredom
or excess of work (which, in a first instance, are both
characterized by slowed performance).
PECCS2015-5thInternationalConferenceonPervasiveandEmbeddedComputingandCommunicationSystems
26