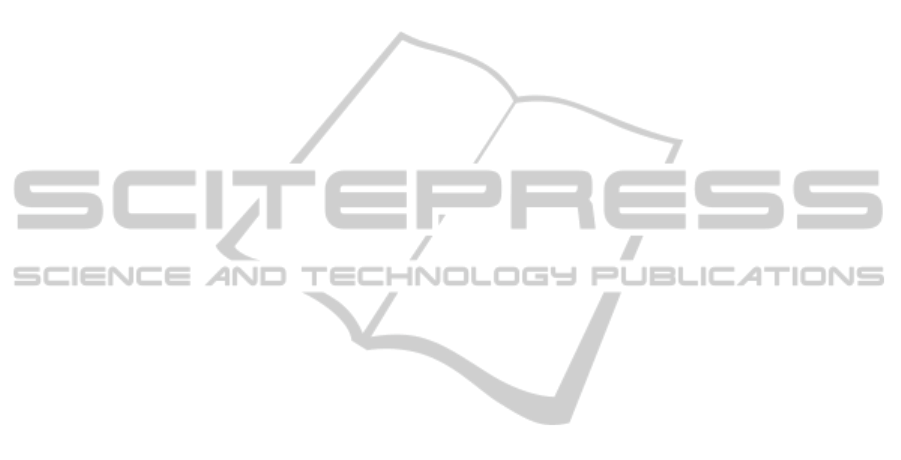
5 DISCUSSION
Several points of this work could be discussed. First
of all, the platform targets fields such as marketing
and medical research, which means its subject could
be the major population. Yet, our experimentations
conducted on subjects ageing from 20 to 30 years
old. Models built for this population are certainly not
accurate for subjects’ ageing between 40 and 50
years old. What’s more, most of the subjects came
because they were intrigued by the experiment: half
of the sample has a psychology background, making
them interested in research concerning emotions.
These people, knowing the research topic, might
cause a certain social desirability bias. The
experimental conditions also play an important role
on how people react: subjects might not have the
same physiological reactions at different time of the
day.
6 CONCLUSIONS
Today, the areas of emotion recognition are seen as
an alternative to the discrimination of different
human feelings, thanks to physiological signals
collected by easy-to-handle, embedded, electronic
equipment. In this paper, we showed the use of
different types of physiological signals to assess
emotions. Electrodermal activity was collected by an
EDA sensor (TEA), and heart activity was measured
through a biofeedback sensor (Nonin). An
experiment involving 35 subjects was carried out to
identify the physiological signals corresponding to
the six basic emotions proposed by Ekman (joy,
sadness, fear, surprise, anger, disgust). Participants
were exposed to a set of 12 videos (2 for each
emotion). Videos set was partially validated through
a subjective rating system. In our future work, we
focus on confirming the benefits of multimodality
for emotions recognition with bio-signals, as well as
on integrating an electrocardiogram sensor (ECG)
which could bring more swiftness to the system.
REFERENCES
Ang, K., Wahab, A., Quek, H. & Khosrowabadi, R., 2010.
Eeg-based emotion recognition using self-organizing
map for boundary detection. In: I. C. Society, ed.
Proceedings of the 2010 20th International Conference
on Pattern Recognition,. Washington, DC, USA: s.n.,
p. 4242–4245.
Barreto, A. & Zhai, J., 2006. Stress detection in computer
users through noninvasive monitoring of physiological
signals. In: Biomedical Science Instrumentation.
s.l.:s.n., p. 495–500.
Bartolini, E. E., 2011. Eliciting Emotion with Film:
Development of a Stimulus Set, Wesleyan University:
s.n.
Bednarski, J. D., March 29, 2012. Eliciting Seven Discrete
Positive Emotions Using Film Stimuli, Vanderbilt
University: s.n.
Bercher, J.-F. & Baudoin, G., 2001. Transformée de
Fourier Discrète,» École Supérieure d’Ingénieurs en
Électrotechnique et Électronique. s.l.:s.n.
Carvalho, S., Leite, J., Galdo-Álvarez, S. & Gonçalves,
O., 2012. The Emotional Movie Database (EMDB): a
self-report and psychophysiological study.,
Neuropsychophysiology Lab Cipsi, School of
Psychology, University of Minho, Campus de Gualtar,
Braga, Portugal.: s.n.
Cowie, R. et al., n.d. Emotion recognition in human-
computer interaction. Signal Processing Magazine,
IEEE.
Croucher, M. & Sloman, A., 1981. Why robots will have
emotions. Originally appeared in Proceedings IJCAI
1981 ed. Vancouver, Sussex University as Cognitive
Science: s.n.
Darwin, C., 1872. The expression of emotion in man and
animal. Chicago: University of Chicago Press
(reprinted in 1965).
Ekman, P., 1999. In: Basic emotions. New York: Sussex
U.K.: John Wiley and Sons, Ltd, p. 301–320.
Ekman, P. & Friesen, W., 1982. Measuring facial
movement with the facial action coding system. In:
Emotion in the human face (2nd ed.). s.l.:New York:
Cambridge University Press.
Friesen, W. & Ekman, P., 1978. Facial Action Coding
System: A Technique for Measurement of Facial
Movement.. Palo Alto, California: Consulting
Psychologists Press.
Gray, J., 1982. The neuropsychology of anxiety - an
inquiry into the functions of the septo-hippocampal
system. s.l.:s.n.
Hamdi, H., 2012. Plate-forme multimodale pour la
reconnaissance d’émotions via l’analyse de signaux
physiologiques : Application à la simulation
d’entretiens d’embauche, Université d’Angers, France:
s.n.
Hamdi, H., Richard, P., Suteau, A. & Saleh, M., 2011.
Virtual reality and affective computing techniques for
face-to-face communication. In: GRAPP 2011 -
Proceedings of the International Conference on
Computer Graphics Theory and Applications. Algarve,
Portugal: s.n., p. 357–360.
Healey, J., Vyzas, E. & Picard, R., 2001. Toward machine
emotional intelligence: Analysis of affective
physiological state. In: IEEE Transactions on Pattern
Analysis and Machine Intelligence. s.l.:s.n., p. 1175–
1191.
Herbelin, B. et al., 2004. Using physiological measures for
emotional assessment: a computer-aided tool for
EmotionRecognitionbasedonHeartRateandSkinConductance
31