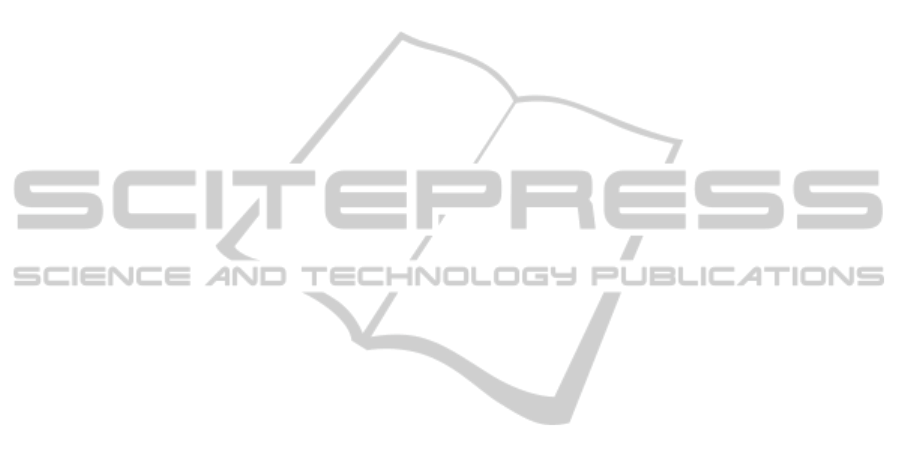
The experimental results show that the proposed
method is capable of tracking a moving object
equipped with a sensor node device. In contrast to
RIPS (Maroti, 2005) or SRIPS (Dil, 2011), the
proposed tracking algorithm utilizes only one
measurement frequency, resulting shorter
measurement times for each measurement round.
Thus the phase difference measurement errors,
caused by the object movement, can be minimized
and the proposed method is able to provide a robust
tracking, while preserving the few centimeters of
accuracy of the interferometric-based localization
techniques.
5 CONCLUSIONS
In this paper a novel object tracking method was
proposed, which utilizes radio-interferometric phase
measurements and confidence maps. The presented
solution enhances the robustness and quality of the
position estimations when bad measurements are
present or measurements are temporarily missing,
using (1) a new peak detection method utilizing a
dynamic threshold, and (2) by a novel prediction
method, which is able to substitute missing estimates
with predicted positions. The proposed prediction
method examines the evolution of the confidence
map in time and calculates the predicted position
from it, without any external model on the
movement of the tracked object.
The complexity of the proposed algorithm is
moderate thus it can be implemented in real time
using ordinary computers.
The performance of the proposed algorithm was
illustrated and evaluated by real measurements. The
proposed prediction method was validated: the mean
prediction error was typically 5 mm, while the
maximum error was 21 mm during the experiment.
The increased robustness of the algorithm was
clearly shown in the experiments where the
proposed algorithm was able to follow the object
movement with maximum error of 91 mm, despite
of the measurement noise and errors.
ACKNOWLEDGEMENTS
This work was partially supported by the Hungarian
State and the European Union under project
TAMOP-4.2.2.-C-11/1/KONV- 2012-0004.
REFERENCES
Au, A.W.S., et al, 2012. Indoor Tracking and Navigation
Using Received Signal Strength and Compressive
Sensing on a Mobile Device. IEEE Transactions on
Mobile Computing, Vol. 12, No. 10, pp. 2050–2062.
Dil B.J., Havinga, P.J.M., 2011. Stochastic Radio
Interferometric Positioning in the 2.4 GHz Range.
Proceedings of the 9th ACM Conference on Embedded
Networked Sensor Systems (SenSys 11), Seattle, WA,
pp. 108-120.
Kai Ni, Kannan, A., Criminisi, A., Winn, J., 2009.
Epitomic Location Recognition. IEEE Transactions on
Pattern Analysis and Machine Intelligence, Vol.31,
No.12, pp. 2158-2167.
Lanzisera, S., Zats, D., Pister, K. S. J., 2011. Radio
Frequency Time-of-Flight Distance Measurement for
Low-Cost Wireless Sensor Localization. IEEE Sensors
Journal, Vol. 11, No. 3, pp.837-845.
Maroti M., et al, 2005. Radio Interferometric Geolocation.
In ACM Third International Conference on Embedded
Networked Sensor Systems (SenSys 05), San Diego,
CA, pp. 1-12.
Mulloni, A., Wagner, D., Barakonyi, I., Schmalstieg,D.,
2009. Indoor Positioning and Navigation with Camera
Phones, IEEE Pervasive Computing, Vol. 8, No. 2, pp.
22-31.
Peng, C., Shen, G., Zhang, Y., Li, Y., Tan, K., 2007.
BeepBeep: a high accuracy acoustic ranging system
using COTS mobile devices. In Proceedings of the 5th
international conference on Embedded networked
sensor systems pp. 1-14.
Se, S., Lowe, D., Little, J., 2001. Vision-based mobile
robot localization and mapping using scale-invariant
features. Proceedings of 2001 IEEE International
Conference on Robotics and Automation, Vol.2, pp.
2051-2058.
Zachár, G., Simon, G., 2014. Radio-Interferometric Object
Trajectory Estimation. Proc. 3rd International
Conference on Sensor Networks, SENSORNETS 2014,
pp. 268-273.
SENSORNETS2015-4thInternationalConferenceonSensorNetworks
168