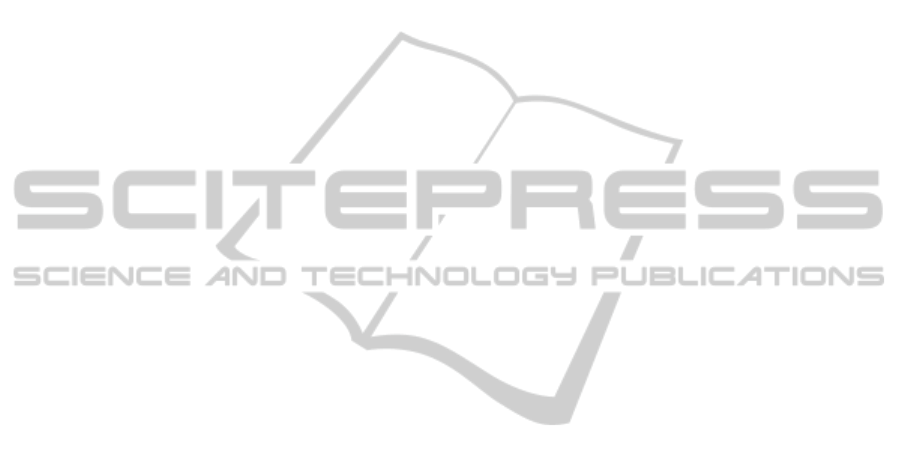
20% for Setups 1 and 2, while it requires 30% for the
standard CS scheme to achieve the same SNR value.
Consequently, our proposed systems can achieve
better video quality using lower complexity
acquisition devices.
5 CONCLUSION AND FUTURE
WORK
In this paper, an efficient perceptual weighting
strategy is adopted into CS framework for video
acquisition to improve the perceived quality of the
reconstructed signal. This goal has been achieved
through three different setups. The performance
evaluation for different setups demonstrates
remarkable improvements over standard CS in terms
of both SNR and SSIM metrics. While Setup 1 and 2
are competing, Setup3 shows the best performance
among all setups. Setup 3 can achieve a peak SSIM
gain of ~ 61% on FS for Container sequence and ~
70% on FS for News sequence. In addition, Setup 3
can achieve a peak SNR gain of ~ 15.62 dB for
Container sequence, and ~ 10.7 dB for News
sequence. The efficacy of our proposed systems for
low complexity acquisition devices has been
demonstrated.
This work is applied for single view video and
exploits only sparsity in spatial direction for separate
frames. As a future work, we aim to give attention to
inter-frame correlation to exploit the sparsity in the
temporal direction of the video rather than exploiting
only the sparsity in the spatial direction. This can
improve the compression order obtained through
compressed sensing. In addition, we aim to extend
our perceptual based system to multi-view video
coding. Quantization effects also can be considered
for future work.
ACKNOWLEDGEMENTS
This work has been supported by the Egyptian
Mission of Higher Education (MoHE). I am grateful
to Egypt-Japan University of Science and
Technology (E-JUST) for offering the tools and
equipment needed.
REFERENCES
Arizona State University, 2014. YUV video sequences
[online]. Available from: http://trace.eas.asu.edu/yuv/
[Accessed 26 Nov 2014].
Candes, E., Romberg, J., and Tao, T., 2006a. Robust
uncertainty principles: exact signal reconstruction from
highly incomplete frequency information. IEEE
transactions on information theory, 52 (2), 489 – 509.
Candes, E., Romberg, J., and Tao, T., 2006b. Stable signal
recovery from incomplete and inaccurate
measurements. Communications on pure and applied
mathematics, 59 (8), 1207–1223.
Candes, E., Wakin, M., and Boyd, S., 2008. Enhancing
sparsity by reweighted ℓ 1 minimization. Fourier
analysis and applications, special issue on sparsity, 14
(5), 877–905.
Donoho, D. L., 2006. Compressed sensing. IEEE
transactions on information theory, 52 (4), 1289–1306.
Figueiredo, M., Nowak, R. D., and Wright, S. J., 2009.
GPSR: Gradient Projection for Sparse Reconstruction:
Matlab source code [online]. Available from:
http://www.lx.it.pt/~mtf/GPSR/ [Accessed 22 Sep
2014].
Figueiredo, Má. a. T., Nowak, R. D., and Wright, S. J.,
2007. Gradient Projection for Sparse Reconstruction:
Application to Compressed Sensing and Other Inverse
Problems. IEEE Journal of Selected Topics in Signal
Processing, 1 (4), 586–597.
Friedlander, M. P., Mansour, H., Saab, R., and Yilmaz, O.,
2011. Recovering Compressively Sampled Signals
Using Partial Support Information. IEEE Transactions
on Information Theory, 58 (2), 1122–1134.
ITU-T, 2003. Advanced video coding for generic audio-
visual services. H.264 and ISO/IEC 14496-10 (AVC),
ITU-T and ISO/IEC JTC 1, May 2003 (and subsequent
editions).
Lee, H., Oh, H., Lee, S., and Bovik, A. C., 2013. Visually
weighted compressive sensing: measurement and
reconstruction. IEEE transactions on image processing,
22 (4), 1444–1455.
Lee, J. and Ebrahimi, T., 2012. Perceptual video
compression: a survey. IEEE Journal of selected topics
in signal processing, 6 (6), 684–697.
Lin, Y. and Zhang, X., 2013. Recent development in
perceptual video coding. In: International conference
on wavelet analysis and pattern recognition (ICWAPR).
Tianjin, 259–264.
Mansour, H. and Yilmaz, O., 2012. Adaptive compressed
sensing for video acquisition. In: IEEE international
conference on acoustics, speech and signal processing
(ICASSP). Kyoto, 3465 – 3468.
MathWorks, 2013. MATLAB - the Language of technical
computing [online]. Available from:
http://www.mathworks.com/products/matlab/.
Park, J.Y., Yap, H.L., Rozell, C.J., and Wakin, M.B., 2011.
Concentration of measure for block diagonal matrices
with applications to compressive sensing. IEEE
transaction on signal processing
, 5859 – 5875.
PerceptuallyWeightedCompressedSensingforVideoAcquisition
215