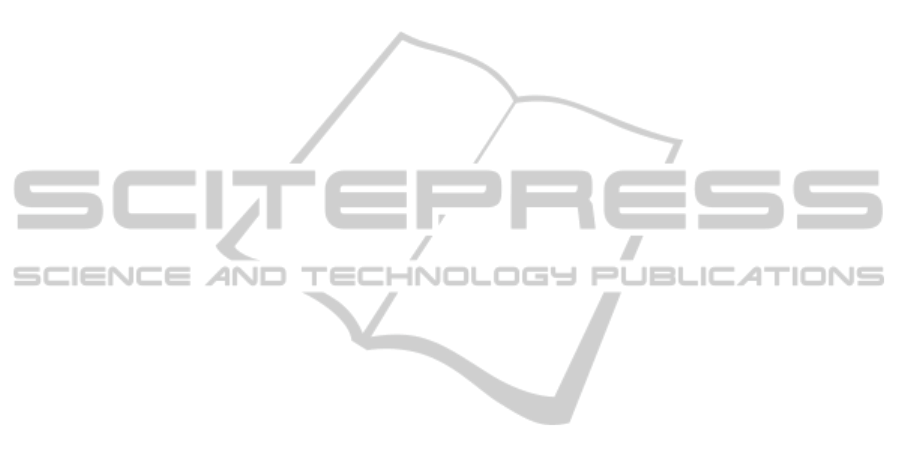
their neighborhood. Thus, beads with a small in-
tensity may be overilluminated by a strong effect
from their neighborhood (Figure 1).
• Errors in the process of bead localization on the
microarray. They are typical for all beads, but
larger for beads with a lower expression level.
• Errors in the process of bead type identification.
• Urealistic assumption that the expressions of each
gene have the same variability.
• Poisson distribution is more adequate for model-
ing the noise because the measured fluorescence
intensities actually correspond to counts of indi-
vidual photons.
• Sensitivity to discretization of coordinates. The
result is highly influenced by fractional coordi-
nates of the bead, because the assumption of lin-
earity of the intensities of the image between
neighboring pixels is strongly violated.
ACKNOWLEDGEMENTS
The work was financially supported by the Neuron
Foundation for Supporting Science. The work of
A. Schlenker was also supported by the specific re-
search project 260034 (Semantic Interoperability in
Biomedicine and Health Care) of Charles University
in Prague.
REFERENCES
Cardona, A. and Tomancak, P. (2012). Current challenges in
open-source bioimage informatics. Nature Methods,
9:661–665.
Davies, P. and Gather, U. (2005). Breakdown and groups.
Annals of Statistics, 33:997–1035.
Dunning, M., Barbosa-Morais, N., Lynch, A., Tavar´e, S.,
and Ritchie, M. (2008). Statistical issues in the analy-
sis of Illumina data. BMC Bioinformatics, 9(85).
Dunning, M., M.L. Smith, M. R., and Tavar´e, S. (2007).
Beadarray: R classes and methods for Illumina bead-
based data. Bioinformatics, 23:2183–2184.
Filzmoser, P. and Todorov, V. (2011). Review of robust mul-
tivariate statistical methods in high dimension. Ana-
lytica Chinica Acta, 705:2–14.
Fraser, K., Wang, Z., and Liu, X. (2010). Microarray im-
age analysis: An algorithmic approach. Chapman &
Hall/CRC, Boca Raton.
G¨ohlmann, H. and Talloen, W. (2009). Gene expression
studies using Affymetrix microarrays. Chapman &
Hall/CRC, Boca Raton.
Kalina, J. (2012). Implicitly weighted methods in robust
image analysis. Journal of Mathematical Imaging and
Vision, 44:449–462.
Kalina, J. (2014). Classification analysis methods for
high-dimensional genetic data. Biocybernetics and
Biomedical Engineering, 34:10–18.
Kalina, J. and Zv´arov´a, J. (2013). Decision support systems
in the process of improving patient safety. In E-health
Technologies and Improving Patient Safety: Exploring
Organizational Factors. IGI Global, Hershey, 71–83.
Kuhn, K., Baker, S., Chudin, E., Lieu, M.-H., Oeser, S.,
Bennett, H., Rigault, P., Barker, D., McDaniel, T., and
Chee, M. (2004). A novel, high-performance random
array platform for quantitative gene expression profil-
ing. Genome Research, 14:2347–2356.
Rueda, L. (2014). Microarray image and data analysis:
Theory and practice. CRC Press, Boca Raton.
Shi, W., Banerjee, A., Ritchie, M., Gerondakis, S., and
Smyth, G. (2009). Illumina WG-6 beadchip strips
should be normalized separately. BMC Bioinformat-
ics, 10(372).
Smith, M., Dunning, M., Tavar´e, S., and Lynch, A. (2010).
Identification and correction of previously reported
spatial phenomena using raw Illumina BeadArray
data. BMC Bioinformatics, 11(208).
Tanaka, G., Suetake, N., and Uchino, E. (2010). Im-
age enhancement based on nonlinear smoothing and
sharpening for noisy images. Journal of Advanced
Computational Intelligence and Intelligent Informat-
ics, 14:200–207.
Tibshirani, R., Hastie, T., and Narasimhan, B. (2003). Class
prediction by nearest shrunken centroids, with ap-
plications to DNA microarrays. Statistical Science,
18:104–117.
Xanthopoulos, P., Pardalos, P., and Trafalis, T. (2013). Ro-
bust data mining. Springer, New York.
BIOIMAGING2015-InternationalConferenceonBioimaging
94