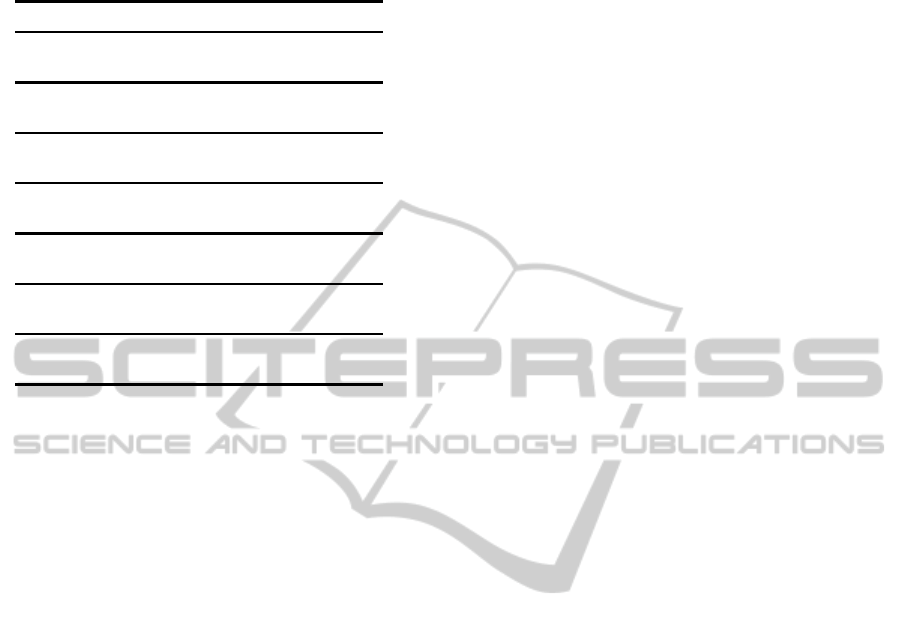
Table 8: The classification of positive positions from ran-
domly selected negative positions.
PB2 PB1 PA HA NP NA NS
K
1
F-value 0.999 1 1 1 1 0.999 1
AUC 1 1 1 1 1 1 1
K
2
F-value 0.999 1 1 1 1 0.999 1
AUC 1 1 1 1 1 1 1
K
×
F-value 1 1 0.999 1 0.998 1 0.994
AUC 1 1 1 1 0.999 1 0.995
K
∩
F-value 1 1 0.999 1 0.997 1 0.995
AUC 1 1 1 1 0.999 1 0.996
K
t
AM
F-value 0.909 0.935 0.721 0.959 0.916 0.825 –
AUC 0.981 0.961 0.745 0.984 0.988 0.918 –
K
t
LP
F-value 0.966 0.912 0.818 0.603 0.984 0.944 0.521
AUC 0.986 0.933 0.856 0.585 0.999 0.952 0.330
K
r
LP
F-value 1 1 1 1 1 1 0.916
AUC 1 1 1 1 1 1 0.966
and positions in them of influenza A viruses by using
the phylogenetic tree and the nucleotide sequence ker-
nels. Then, we have observed that both the nucleotide
sequence kernels and the phylogenetictree kernels are
effective to the pandemic classification. Also the nu-
cleotide sequence kernels and the leaf-path kernel are
effective to the packaging signal analysis. Further-
more, the phylogenetic tree kernels and none of nu-
cleotide sequence kernels are effective to the regional
analysis.
In the case that the phylogenetic tree kernels suc-
ceed to classify, two different phylogenetic trees re-
constructed from positive and negative examples or
positions work well as background knowledge in our
classification. This is typical for regional analysis
which the nucleotide sequence kernels fail to classify.
It is a future work to apply the regional analysis
to influenza A (H3N2) viruses and the analysis of po-
sitions in packaging signals to influenza A (H1N1)
viruses. It is also an important future work to compare
the correlated mutations (Shimada et al., 2012) with
our results and to analyze our results from the view-
points of Virology. Furthermore, it is a future work
to analyze, classify and evaluate another nucleotide
sequences by using the phylogenetic tree kernels and
the nucleotide sequence kernels.
REFERENCES
Bao, Y., Bolotov, P., Dernovoy, D., Kiryutin, B., Za-
slavsky, L., Tatusova, T., Ostell, J., and Lip-
man, D. (2008). The influenza virus resource
at the National Center for Biotechnology Informa-
tion. J. Virol., 82:596–601. Also available at:
http://www.ncbi.nlm.gov/genomes/FLU/.
Chang, C.-C. and Lin, C.-J. (2013). LIBSVM – A library
for support vector machine (version 3.17). Available
at http://www.csie.ntu.edu.tw/˜cjlin/libsvm.
Durbin, R., Eddy, S., Krogh, A., and Mitchison, G. (1998).
Biological sequence analysis: Probabilistic models
of proteins and nucleic acids. Cambridge University
Press.
G¨artner, T. (2008). Kernels for structured data. World Sci-
entific.
Hamada, I., Shimada, T., Hirata, K., and Kuboyama, T.
(2013). Agreement subtree mapping kernel for phy-
logenetic trees. In Proc. DDS 13, pages 1–8.
Hutchinson, E. C., von Kirchbach, J. C., Gog, J. R., and
Digard, P. (2010). Genome packaging in influenza A
virus. J. Gen. Virol., 91:313–328.
Leslie, C. S., Eskin, E., and Noble, W. S. (2002). The spec-
trum kernel: A string kernel for svm protein classifi-
cation. In Proc. PSB 2002, pages 566–575.
Makino, S., Shimada, T., Hirata, K., Yonezawa, K., and
Ito, K. (2012a). A trim distance between positions as
packaging signals in H3N2 influenza viruses. In Proc.
SCIS-ISIS 2012, pages 1702–1707.
Makino, S., Shimada, T., Hirata, K., Yonezawa, K., and Ito,
K. (2012b). A trim distance between positions in nu-
cleotide sequences. In Proc. DS 2012 (LNAI 2569),
pages 81–94.
Shimada, T., Hamada, I., Hirata, K., Kuboyama, T.,
Yonezawa, K., and Ito, K. (2013). Clustering of po-
sitions in nucleotide sequences by trim distance. In
Proc. IIAI AAI 2013, pages 129–134.
Shimada, T., Hazemoto, T., Makino, S., Hirata, K., and Ito,
K. (2012). Finding correlated mutations among rna
segments in H3N2 influenza viruses. In Proc. SCIS-
ISIS 2012, pages 1696–1705.
Sung, W.-K. (2009). Algorithms in bioinformatics: A prac-
tical introduction. Chapman & Hall/CRC.
ClassifyingNucleotideSequencesandtheirPositionsofInfluenzaAVirusesthroughSeveralKernels
347