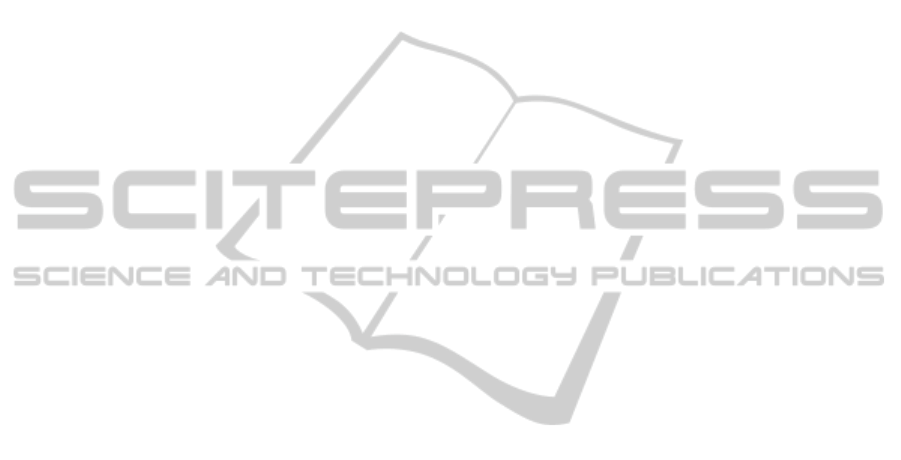
hood of a configuration is by dihedral rotations of one
or several covalent bonds by some, usually small, an-
gle. Atoms on one side of the rotating bond remain
stationary while the others rotate on orbits in parallel
planes and with centers on a common axis. Similarly,
if a covalent bond on a side chain is rotated, only a
very limited number of atoms on the side chain ro-
tates while all other atoms remain stationary.
Kinetic data structures for objects moving on
piecewise continuous trajectories are far from trivial.
The determination of how and when such data struc-
tures must be updated typically involves finding roots
of high-degree polynomials. For DC s, deciding when
a k-simplex T , k ≤ 3, becemes (seizes to be) Delau-
nay involves finding roots of polynomials of 8-th de-
gree (Russel, 2007). In α-complexes, it is necessary
to determine when a k-simplex T , k ≤ 3, becomes
(seizes to be) Gabriel and when it becomes (seizes to
be) short (Kerber and Edelsbrunner, 2013).
Fortunately, when rotating covalent bonds of pro-
teins, the computational effort of updating kinetic
data structures can be significantly reduced. It can
be shown that kinetic DC s and kinetic α-complexes
for this kind of restricted and coordinated movement
of objects (with the same rotational velocity) involve
finding roots of polynomials of degree at most 4. Fur-
thermore, as the results presented in this paper indi-
cate, the depth d of a neighborhood N
∗
d
(i) of vertex i
does not need to be greater than 2 or 3. Hence, these
neighborhoods can be updated efficiently along with
the α-complexes.
In conclusion, α-complexes of proteins with rel-
atively low α do capture the potential energy contri-
butions of nonbonded atoms. Furthermore, kinetic α-
complexes for restricted types of motion can prove
useful in protein structure prediction when searching
through the vast atomic configuration space.
REFERENCES
Edelsbrunner, H. (1992). Weighted alpha shapes. Univer-
sity of Illinois at Urbana-Champaign, Department of
Computer Science.
Edelsbrunner, H., Facello, M., and Liang, J. (1998). On the
definition and the construction of pockets in macro-
molecules. Discrete Applied Mathematics, 88(1):83–
102.
Edelsbrunner, H. and M
¨
ucke, E. P. (1994). Three-
dimensional alpha shapes. ACM Transactions on
Graphics, 13(1):43–72.
Kerber, M. and Edelsbrunner, H. (2013). 3d kinetic al-
pha complexes and their implementation. In ALENEX,
pages 70–77. SIAM.
Kim, D.-S., Seo, J., Kim, D., Ryu, J., and Cho, C.-H.
(2006). Three-dimensional beta shapes. Computer-
Aided Design, 38(11):1179–1191.
Levitt, M., Hirshberg, M., Sharon, R., and Daggett, V.
(1995). Potential energy function and parameters for
simulations of the molecular dynamics of proteins and
nucleic acids in solution. Computer Physics Commu-
nications, 91(1):215–231.
Lotan, I., Schwarzer, F., Halperin, D., and Latombe, J.-
C. (2004). Algorithm and data structures for effi-
cient energy maintenance during monte carlo simula-
tion of proteins. Journal of Computational Biology,
11(5):902–932.
Russel, D. (2007). Kinetic Data Structures in Practice. PhD
thesis, Stanford, CA, USA.
Schlick, T. (2010). Molecular Modeling and Simulation:
An Interdisciplinary Guide, volume 21. Springer.
Seidel, R. (1995). The upper bound theorem for polytopes:
an easy proof of its asymptotic version. Computa-
tional Geometry, 5(2):115–116.
Winter, P. and Fonseca, R. (2012). Adjustable chain
trees for proteins. Journal of Computational Biology,
19(1):83–99.
Winter, P., Sterner, H., and Sterner, P. (2009). Alpha shapes
and proteins. In ISVD’09. Sixth International Sympo-
sium on Voronoi Diagrams, pages 217–224. IEEE.
Zhou, W. and Yan, H. (2014). Alpha shape and delaunay
triangulation in studies of protein-related interactions.
Briefings in Bioinformatics, 15(1):54–64.
BIOINFORMATICS2015-InternationalConferenceonBioinformaticsModels,MethodsandAlgorithms
182