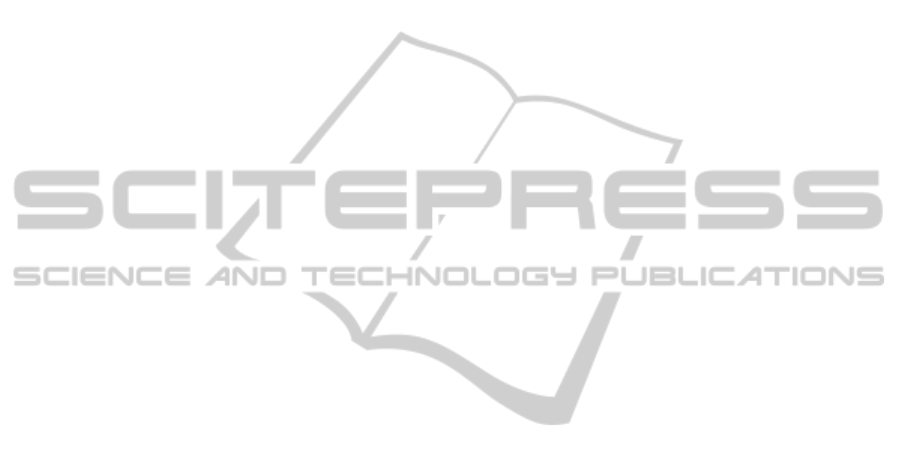
are concerned as well.
4 CONCLUSION
We proposed an original statistical model for fourth
order tensor ODF modeling of DW-MRI. This models
incorporates a suitable metric in the parameter space.
It relies on nonlinear dimension reduction, and on
an original statistic in the reduced space, allowing to
compare two populations and to extract biomarkers.
This approach has shown better ability to discriminate
two populations, as compared to models relying on
other metrics, on T2 ODF profiles, or on the Hotelling
test. It has thus a potential for early diagnosis.
Resorting to T4 ODF profiles will also enable us
to identify more precisely the changes effectively tak-
ing place between the two populations, as compared
to changes identified by T2 models where the profiles
are described less accurately. This is left for future
work.
REFERENCES
Arsigny, V., Fillard, P., Pennec, X., and Ayache, N. (2006).
Log-Euclidean metrics for fast and simple calculus on
diffusion tensors. Magnetic Resonance in Medicine,
56(2):411–421.
Barmpoutis, A., Bing, J., and Vemuri, B. (2009). Adap-
tive kernels for multi-fiber reconstruction. In Proceed-
ings of the Information Processing in Medical Imag-
ing conference (IPMI), volume 21, pages 338–349.
Barmpoutis, A., Vemuri, B., and Forder, J. (2007). Regis-
tration of high angular resolution diffusion MRI im-
ages using 4th order tensors. In Int. Conf. on Med-
ical Image Computing and Computer Assisted Inter-
vention (MICCAI), volume 4791 of Lecture Notes in
Computer Science, pages 908–915. Springer.
Du, J., Goh, A., Kushnarev, S., and Qiu, A. (2014).
Geodesic regression on orientation distribution func-
tions with its application to an aging study. NeuroIm-
age, 87:416–426.
Duarte-Carvajalino, J., Sapiro, G., Harel, N., and Lenglet,
C. (2013). A framework for linear and non-linear reg-
istration of diffusion-weighted MRIs using angular in-
terpolation. Frontiers in Neuroscience, 7.
Hastie, T., Tibshirani, R., and Friedman, J. (2011). The ele-
ments of statistical learning. Springer, second edition.
He, X. and Niyogi, P. (2003). Locality preserving projec-
tions. In Advances in Neural Information Processing
Systems 16 (NIPS), volume 16, pages 153–160.
He, X., Yan, S., Hu, Y., Niyogi, P., and Zhang, H.-J. (2005).
Face recognition using Laplacianfaces. IEEE Trans-
actions on Pattern Analysis and Machine Intelligence,
27(3):328–340.
Horsfield, M. A. and Jones, D. K. (2002). Applications of
diffusion-weighted and diffusion tensor MRI to white
matter diseases – a review. NMR in Biomedicine,
15(7-8):570–577.
Jenkinson, M., Beckmann, C., Behrens, T., Woolrich, M.,
and Smith, S. (2012). FSL. NeuroImage, 62(2):782–
790.
Jones, D. K. (2011). Diffusion MRI: theory, methods, and
applications. Oxford University Press.
Ozarslan, E. and Mareci, T. H. (2003). Generalized dif-
fusion tensor imaging and analytical relationships be-
tween diffusion tensor imaging and high angular res-
olution diffusion imaging. Magnetic Resonance in
Medicine, 50(5):955–965.
Scott, D. (1992). Multivariate density estimation: theory,
practice, and visualization. Wiley.
Sfikas, G., Constantinopoulos, C., Likas, A., and Galat-
sanos, N. (2005). An analytic distance metric for
Gaussian mixture models with application in image
retrieval. In 15th International Conference on Arti-
ficial Neural Networks (IEEE ICANN 2005), volume
3697 of Lecture Notes in Computer Science, pages
835–840. Springer.
Smith, S., Jenkinson, M., Johansen-Berg, H., Rueckert, D.,
Nichols, T., Mackay, C., Watkins, K., Ciccarelli, O.,
Cader, M. Z., Matthews, P., and Behrens, T. (2006).
Tract-based spatial statistics: voxelwise analysis of
multi-subject diffusion data. NeuroImage, 31(4):1487
– 1505.
Tao, X. and Miller, J. (2006). A method for registering
diffusion weighted magnetic resonance images. In
Int. Conf. on Medical Image Computing and Com-
puter Assisted Intervention (MICCAI), volume 4191
of Lecture Notes in Computer Science, pages 594–
602. Springer.
Tarantola, A. (2005). Elements for physics: quantities,
qualities, and intrinsic theories. Springer.
Tenenbaum, J. B., Silva, V., and Langford, J. C. (2000). A
global geometric framework for nonlinear dimension-
ality reduction. Science, 290(5500):2319–2323.
Tuch, D. S. (2004). Q-ball imaging. Magnetic Resonance
in Medicine, 52(6):1358–1372.
Verma, R., Khurd, P., and Davatzikos, C. (2007). On an-
alyzing diffusion tensor images by identifying mani-
fold structure using isomaps. IEEE Transactions on
Medical Imaging, 26(6):772–778.
Weinberger, K. and Saul, L. (2006). Unsupervised learning
of image manifolds by semidefinite programming. In-
ternational Journal of Computer Vision, 70(1):77–90.
Weldeselassie, Y., Barmpoutis, A., and Atkins, M. (2012).
Symmetric positive-definite Cartesian tensor fiber ori-
entation distribution (CT-FOD). Medical Image Anal-
ysis, 16(6):1121–1129.
ICPRAM2015-InternationalConferenceonPatternRecognitionApplicationsandMethods
282