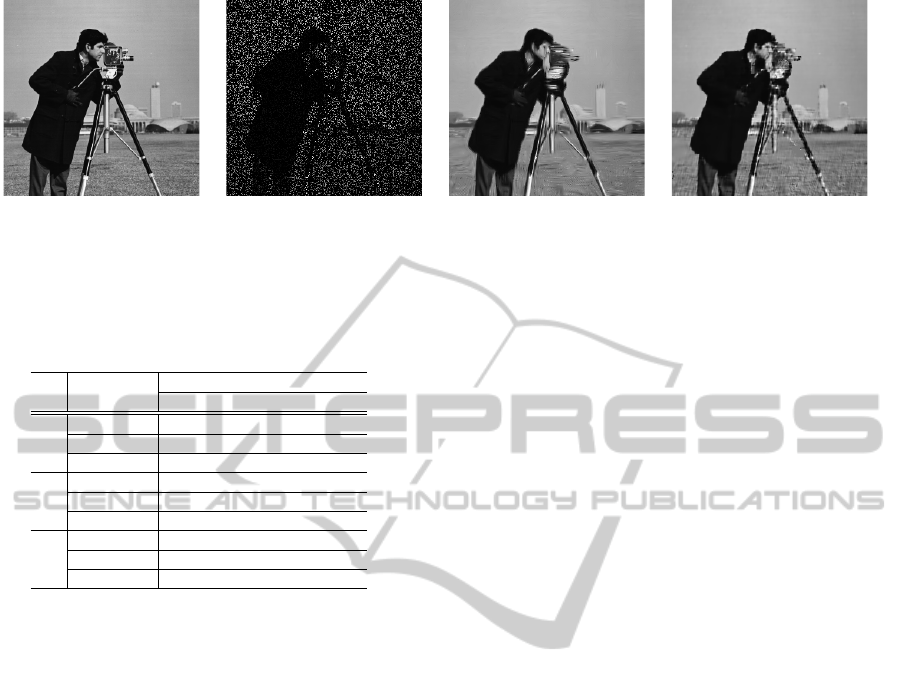
(a) (b) (c) (d)
Figure 3: Inpainting: (a) original image; (b) corrupted image (80% missing pixels; PSNR = 6.56dB); (c) SOP (Ram et al.,
2013); (d) Proposed.
Table 3: Simultaneous inpainting and denoising results in
terms of PSNR - Methods: BP (Zhou et al., 2012); Basic
algorithm (Section 2); Subimages framework (Section 4.3).
σ Data Ratio
Barbara256 (256 × 256)
BP Basic Subimages
5
80% 36.80 37.16 37.25
50% 33.61 33.82 34.02
20% 26.73 27.45 28.04
15
80% 31.24 31.63 31.79
50% 29.31 28.92 29.14
20% 25.17 25.24 25.52
25
80% 28.40 28.92 29.05
50% 26.79 26.46 26.61
20% 23.49 23.53 23.74
pixel estimate, which provide the optimal weights to
combine the patches when assembling the final im-
age. The experimental results shows that the proposed
method is competitive with the state-of-the-art.
REFERENCES
Aharon, M., Elad, M., and Bruckstein, A. (2006). K-SVD:
an algorithm for designing overcomplete dictionaries
for sparse representation. IEEE Trans. on Signal Pro-
cessing, 54:4311–4322.
Bernardo, J. and Smith, A. (1994). Bayesian Theory. Wiley.
Buades, A., Coll, B., and Morel, J. M. (2006). A review of
image denoising methods, with a new one. Multiscale
Modeling and Simulation, 4:490–530.
Cao, Y., Luo, Y., and Yang, S. (2008). Image denoising with
Gaussian mixture model. In Congress on Image and
Signal Proc., vol. 3, pages 339–343.
Chatterjee, P. and Milanfar, P. (2012). Patch-based near-
pptimal image denoising. IEEE Trans. Image Proc.,
21:1635–1649.
Dabov, K., Foi, A., Katkovnik, V., and Egiazarian, K.
(2007). Image denoising by sparse 3D transform-
domain collaborative filtering. IEEE Trans. Image
Proc., 16(8):2080–2095.
Dempster, A. P., Laird, N. M., and Rubin, D. B. (1977).
Maximum likelihood from incomplete data via the
EM algorithm. Journal of the Royal Statistical So-
ciety, Series B, 39(1):1–38.
Eirola, E., Lendasse, A., Vandewalle, V., and Biernacki, C.
(2014). Mixture of Gaussians for distance estimation
with missing data. Neurocomputing, 131:32–42.
Elad, M. and Aharon, M. (2006). Image denoising via
sparse and redundant representations over learned dic-
tionaries. IEEE Trans. Image Proc., 15:3736–3745.
Elad, M., Figueiredo, M., and Ma, Y. (2010). On the role
of sparse and redundant representations in image pro-
cessing. Proceedings of the IEEE, 98:972–982.
Figueiredo, M. and Jain, A. K. (2002). Unsupervised learn-
ing of finite mixture models. IEEE Trans. on Pattern
Analysis and Machine Intelligence, 24:381–396.
Lebrun, M., Buades, A., and Morel, J.-M. (2013). A non-
local Bayesian image denoising algorithm. SIAM J.
Imaging Sciences, 6(3):1665–1688.
Liu, X., Tanaka, M., and Okutomi, M. (2012). Noise level
estimation using weak textured patches of a single
noisy image. In 19th IEEE International Conference
on Image Processing, 2012, pages 665–668.
Ram, I., Elad, M., and Cohen, I. (2013). Image processing
using smooth ordering of its patches. IEEE Trans. on
Image Processing, 22:2764–2774.
Yu, G., Sapiro, G., and Mallat, S. (2012). Solving inverse
problems with piecewise linear estimators: From
Gaussian mixture models to structured sparsity. IEEE
Trans. Image Processing, 21:2481–2499.
Zhou, M., Chen, H., Paisley, J., Ren, L., Li, L., Xing, Z.,
Dunson, D., Sapiro, G., and Carin, L. (2012). Non-
parametric Bayesian Dictionary Learning for Analysis
of Noisy and Incomplete Images. Image Processing,
IEEE Trans. on, 21(1):130–144.
Zoran, D. and Weiss, Y. (2012). Natural Images, Gaussian
Mixtures and Dead Leaves. In Advances in Neural In-
formation Processing Systems 25, pages 1736–1744.
ICPRAM2015-InternationalConferenceonPatternRecognitionApplicationsandMethods
288