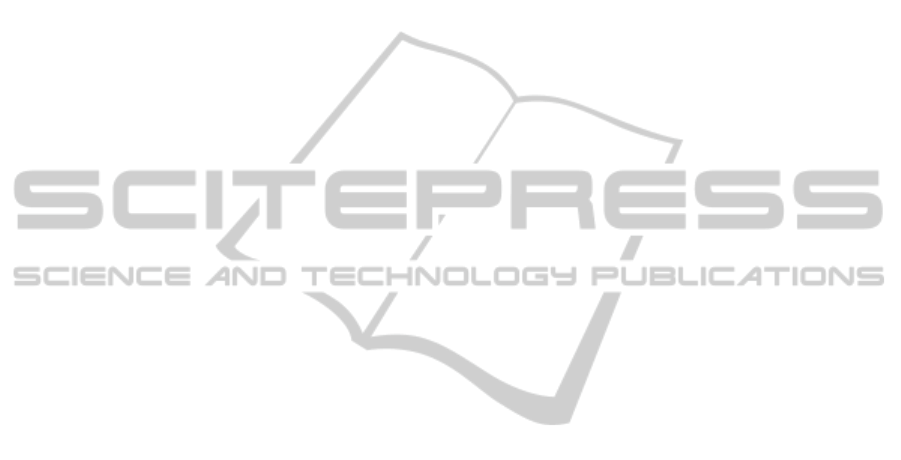
5 CONCLUSION
In this paper, a real-time self-scaling kinematic hand
skeleton model based approach for full hand-finger
pose estimation while determining hand and finger
joints’ angles was presented. The model was itera-
tively adapted on the 3D data of the hand delivered by
a depth camera using a least-squares optimization ap-
proach. Therefore, the data-model distance was sim-
plified allowing the whole pose estimation process
to be done in an optimization process without prior
steps. Further, the model was equipped with a self-
scaling ability to handle different hand sizes automat-
ically.
A detailed evaluation of the approach was given
including quantitative and qualitative results. It was
shown that the approach allows to track the hand’s
skeleton under hard conditions such as turning the
hand and presenting complex finger gestures. Fur-
thermore, the tracking performance on standard hard-
ware without using the GPU is up to 30 FPS, limited
by the camera’s speed. In addition, there are no train-
ing data or prior calculations required. Thus, the pre-
sented method is more efficient than most of the other
known hand-finger tracking approaches.
Future work will focus on the remaining problems
like handling very fast hand or finger movements and
adaptable hand proportions. Some improvements on
the support vector machine based gesture classifier
and a quantitative evaluation are planned. In addition,
an extension of the presented approach to estimating
the arm pose or even the full body pose is intended.
This would enable human robot interaction applica-
tions like controlling an industrial robot or a robotic
hand and could be used for a simple teach-in proce-
dure. Even simultaneous tracking of both hands and
the body is planned.
REFERENCES
Aristidou, A. and Lasenby, J. (2010). Motion Capture
with Constrained Inverse Kinematics for Real-Time
Hand Tracking. In International Symposium on Com-
munications, Control and Signal Processing, number
March, pages 3–5.
Athitsos, V. and Sclaroff, S. (2003). Estimating 3D hand
pose from a cluttered image. 2003 IEEE Computer
Society Conference on Computer Vision and Pattern
Recognition 2003 Proceedings, 2:II–432–9.
Ballan, L., Taneja, A., Gall, J., Gool, L. V., and Pollefeys,
M. (2012). Motion Capture of Hands in Action Us-
ing Discriminative Salient Points. In Fitzgibbon, A.,
Lazebnik, S., Perona, P., Sato, Y., and Schmid, C., ed-
itors, Computer Vision – ECCV 2012, volume 7577 of
Lecture Notes in Computer Science, pages 640–653.
Springer.
ElKoura, G. and Singh, K. (2003). Handrix: animating the
human hand. Eurographics symposium on Computer
animation.
Erol, A., Bebis, G., Nicolescu, M., Boyle, R. D., and
Twombly, X. (2007). Vision-based hand pose estima-
tion: A review. Computer Vision and Image Under-
standing, 108(1-2):52–73.
Gorce, M. D. L., Fleet, D. J., and Paragios, N. (2011).
Model-Based 3D Hand Pose Estimation from Monoc-
ular Video. In IEEE Transactions on Pattern Analysis
and Machine Intelligence, volume 33, pages 1793–
1805. Laboratoire MAS, Ecole Centrale de Paris,
Chatenay-Malabry, IEEE.
Han, J., Shao, L., Xu, D., and Shotton, J. (2013). Enhanced
computer vision with Microsoft Kinect sensor: a re-
view. IEEE transactions on cybernetics, 43(5):1318–
34.
Horaud, R., Forbes, F., Yguel, M., Dewaele, G., and Zhang,
J. (2011). Rigid and articulated point registration with
expectation conditional maximization. IEEE Trans-
actions on Pattern Analysis and Machine Intelligence,
33(3):587–602.
Keskin, C., , Kirac, F., Kara, Y. E., and Akarun, L. (2011).
Real time hand pose estimation using depth sensors.
In Computer Vision Workshops (ICCV Workshops),
2011 IEEE International Conference on, pages 1228–
1234.
Keskin, C., , Kirac, F., Kara, Y. E., and Akarun, L. (2012).
Hand Pose Estimation and Hand Shape Classification
Using Multi-layered Randomized Decision Forests,
volume 7577. Springer Berlin Heidelberg.
Lee, J. and Kunii, T. (1995). Model-based analysis of hand
posture. Computer Graphics and Applications, IEEE,
15(5):77–86.
Liang, H., Yuan, J., and Thalmann, D. (2012). Hand Pose
Estimation by Combining Fingertip Tracking and Ar-
ticulated ICP. In Proceedings of the 11th ACM SIG-
GRAPH International Conference on Virtual-Reality
Continuum and Its Applications in Industry, VRCAI
’12, pages 87–90, New York, NY, USA. ACM.
Oikonomidis, I., Kyriazis, N., and Argyros, A. (2011). Ef-
ficient model-based 3D tracking of hand articulations
using Kinect. Procedings of the British Machine Vi-
sion Conference, pages 101.1–101.11.
Oikonomidis, I., Kyriazis, N., and Argyros, A. A. (2010).
Markerless and Efficient 26-DOF Hand Pose Recov-
ery. Hand The, pages 744–757.
Qian, C., Sun, X., Wei, Y., Tang, X., and Sun, J. (2014).
Realtime and Robust Hand Tracking from Depth. In
IEEComputer Vision and Pattern Recognition.
Raheja, J. L., Chaudhary, A., and Singal, K. (2011). Track-
ing of Fingertips and Centers of Palm Using KINECT.
Third International Conference on Computational In-
telligence Modelling Simulation, pages 248–252.
Ren, Z., Meng, J., and Yuan, J. (2011). Depth Camera
Based Hand Gesture Recognition and its Applications
in Human-Computer-Interaction. IEEE International