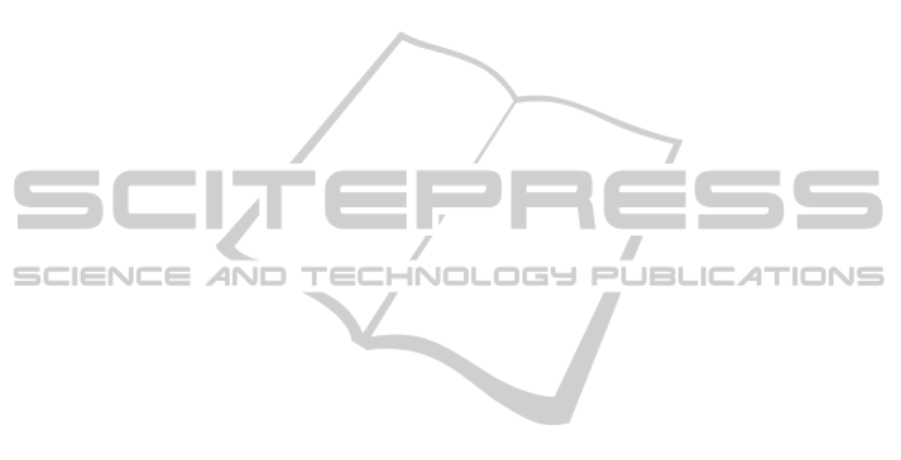
local structure of metabolic networks presented in
this article shows several desirable features.
First, it represents local topological structures as
bipartite and directed graphs, which permits an
unambiguous description of the chemical reaction
patterns involved.
Second, it presents a comprehensive enumeration
of the structures within a prescribed scope and
highlights the topological relationships among
structures, facilitating a comprehensive examination
of statistical trends.
Third, it prompts a comprehensive visualization
of structure statistics and of statistic relationships
among topologically related structures (e.g. Figure
2). This will facilitate direct comparisons among the
local-topological characteristics of the metabolic
networks of distinct organisms and organelles,
which can be put in relation to their environments
and functions.
Fourth, the approach to detecting potential
selective constraints does not hinge on null models
based on physically meaningless random networks.
It emphasises not so much the absolute frequencies
of the structures as the relative frequencies among
topologically related structures. Therefore it puts in
relief the local-context dependence of the
presence/absence of additional reactions,
reversibility, etc. We presented above several
statistics that help detecting strong bias.
Applied to the S. cerevisiae metabolic network,
the approach highlights a preference for sparse
structures. It also highlights some very strong
context-dependence of the reversibility of reactions
and of the presence/absence of some types of
reactions. The underpinnings of these trends deserve
further investigation as a way to reveal functional
(e.g. dynamic) properties underlying an evolutionary
preference for some reaction-coupling
configurations, with the potential to guide synthetic
biology and metabolic engineering approaches.
Ongoing algorithmic developments include the
expansion of the topological scope of the analysis
and strategies to efficiently navigate the network of
topologically related structures towards highly
represented complex structures.
ACKNOWLEDGEMENTS
We acknowledge grants PEst-C/SAU/LA0001/2013-
2014, PEst-OE/MAT/UI0152 and FCOMP-01-0124-
FEDER-020978 financed by FEDER through the
“Programa Operacional Factores de
Competitividade, COMPETE” and by national funds
through “FCT, Fundação para a Ciência e a
Tecnologia” (project PTDC/QUI-
BIQ/119657/2010).
REFERENCES
Aittokallio, T. & Schwikowski, B. 2006. Graph-based
methods for analysing networks in cell biology.
Briefings in Bioinformatics, 7, 243-255.
Artzy-Randrup, Y., Fleishman, S. J., Ben-Tal, N. & Stone,
L. 2004. Comment on "Network motifs: simple
building blocks of complex networks" and
"Superfamilies of evolved and designed networks".
Science (New York, N.Y.), 305, 1107; author reply
1107.
Barabasi, A. L. & Oltvai, Z. N. 2004. Network biology:
Understanding the cell's functional organization.
Nature Reviews Genetics, 5, 101-U15.
Forster, J., Famili, I., Fu, P., Palsson, B. O. & Nielsen, J.
2003. Genome-scale reconstruction of the
Saccharomyces cerevisiae metabolic network. Genome
Research, 13, 244-253.
Milo, R., Shen-Orr, S., Itzkovitz, S., Kashtan, N.,
Chklovskii, D. & Alon, U. 2002. Network Motifs:
Simple Building Blocks of Complex Networks.
Science, 298, 824-827.
Noor, E., Eden, E., Milo, R. & Alon, U. 2010. Central
Carbon Metabolism as a Minimal Biochemical Walk
between Precursors for Biomass and Energy.
Molecular Cell, 39, 809-820.
Shellman, E. R., Burant, C. F. & Schnell, S. 2013.
Network motifs provide signatures that characterize
metabolism. Molecular BioSystems, 9, 352-360.
BIOINFORMATICS2015-InternationalConferenceonBioinformaticsModels,MethodsandAlgorithms
212