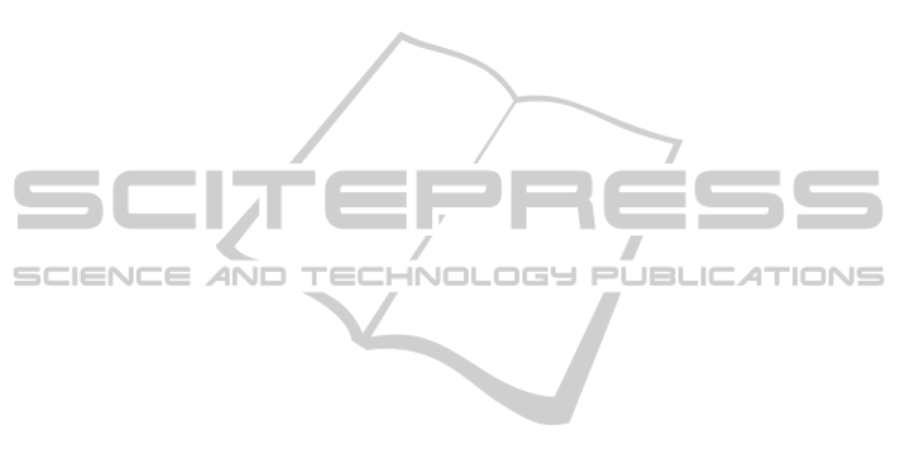
reason for the many existing professions. The basic
structure for handling different task domains may be
the same to a large extent. However, environments,
objects and subjects likely to be encountered as well
as typical behaviors of subjects may vary widely.
Within each task domain there are characteristic
maneuvers to be expected; therefore, driving on
highways, on city roads, on the country side or in the
woods requires different types of attention control
and subjects likely to be detected.
Learning which ones of these subjects with which
parameter sets are to be expected in which situations
is what constitutes “experience in the field”. This
experience allows recognizing snapshots as part of a
process; on this basis expectations can be derived that
allow a) focusing attention in feature extraction on
special events (like occlusion or uncovering of
features in certain regions of future images) or b)
increased resolution in some region of the real world
by gaze control for a bifocal system.
Crucial situation-dependent decisions have to be
made for transitions between mission phases where
switching between behavioral capabilities for the
maneuver is required. That is why representation of
specific knowledge of “maneuvers” is important.
6 CONCLUSIONS
In view of the supposition that human drivers will
expect from ‘autonomous driving’ at least coming
close to their performance levels in the long run, the
discrepancies between systems intended for first
introduction until 2020 and the features needed in the
future for this purpose have been discussed. A
proposal for a “Bifocal active road vehicle Eye” that
seems to be an efficient compromise between
mechanical complexity and perceptual performance
achievable has been reviewed and improved.
‘BarvEye’ needs just one tele-camera instead of more
than seventy mounted fix on the vehicle body to cover
the same high-resolution field of view. With respect
to hardware components needed, there is no
insurmountable barrier any more for volume or price
of such a system, as compared to the beginnings. The
software development in a unified design for detailed
perception of individuals with their specific habits
and limits continues to be a demanding challenge
probably needing decades to be solved. Learning
capabilities on all three levels of knowledge (visual
features, objects / subjects, and situations in task
domains) require advanced vision systems as
compared to those used in the actual introductory
phase.
REFERENCES
Bayerl S.F.X., Wuensche H.-J., 2014. Detection and
Tracking of Rural Crossroads Combining Vision and
LiDAR Measurements. In Proc. IEEE Int'l Conf. on
Intelligent Transportation Systems, 2014.
Bertozzi M., Broggi A, Fascioli A., 2000. Vision-based
intelligent vehicles: State of the art and perspectives.
Robotics and Autonomous Systems 32, pp 1–16.
Burt P., Wixson L., Salgian G., 1995. Electronically
directed “focal” stereo. Proc., Fifth Internat. Conf. on
Computer Vision, pp 94–101.
Dickmanns E.D, 1987: 4-D-Dynamic Scene Analysis with
Integral Spatio-Temporal Models. In: Bolles RC, Roth
B. 1988. Robotics Research, MIT Press, Cambridge.
Dickmanns E.D., Graefe V., 1988. a) Dynamic monocular
machine vision. Machine Vision and Applications,
Springer International, Vol. 1, pp 223-240. b)
Applications of dynamic monocular machine vision.
(ibid), pp 241–261.
Dickmanns E.D., 2007. Dynamic Vision for Perception and
Control of Motion. Springer-Verlag, (474 pp).
Gehrig S., Eberli F., Meyer T., 2009. A Real-time Low-
Power Stereo Vision Engine Using Semi-Global
Matching on an automotive compliant FPGA. ICVS.
Hirschmueller H., 2011 (Sept.). Semi-Global Matching -
Motivation, Developments and Applications. Photo-
grammetric Week, Stuttgart, Germany, pp. 173-184.
IJVAS-1, 2002. Vision for ground vehicles: history and
prospects. Int. Journal of Vehicle Autonomous Systems
(IJVAS), Vol.1 No.1, pp 1-44.
IV’00, 2000. Proc. Internat. Symp. on Intelligent Vehicles,
Dearborn (MI), with six contributions to Expectation-
based, Multi-focal, Saccadic (EMS-) vision:
1. Gregor R. et al.: EMS-Vision: A Perceptual System for
Autonomous Vehicles.
2. Gregor R., Dickmanns E.D.: EMS-Vision: Mission
Performance on Road Networks.
3. Hofmann U.; Rieder A., Dickmanns, E.D.: EMS-
Vision: Applic. to ‘Hybrid Adaptive Cruise Control’.
4. Luetzeler M., Dickmanns E.D.: EMS-Vision: Recog-
nition of Intersections on Unmarked Road Networks.
5. Pellkofer M., Dickmanns E.D.: EMS-Vision: Gaze
Control in Autonomous Vehicles.
6. Siedersberger K.-H., Dickmanns E.D.: EMS-Vision:
Enhanced Abilities for Locomotion.
Matthies L., 1992. Stereo vision for planetary rovers:
Stochastic modeling to near realtime
implementation. IJCV, vol. 8.
Niebles J.C., Han B., Li Fei-Fei, 2010. Efficient Extraction
of Human Motion Volumes by Tracking. IEEE
Computer Vision and Pattern Recogn. (CVPR).
Pellkofer M., Luetzeler M., Dickmanns E.D., 2001.
Interaction of Perception and Gaze Control in
Autonomous Vehicles. Proc. SPIE: Intelligent Robots
and Computer Vision XX; Newton, USA, pp 1-12.
Roland A., Shiman P., 2002. Strategic Computing: DARPA
and the Quest for Machine Intelligence, 1983–1993.
MIT Press.
BarvEye-BifocalActiveGazeControlforAutonomousDriving
435