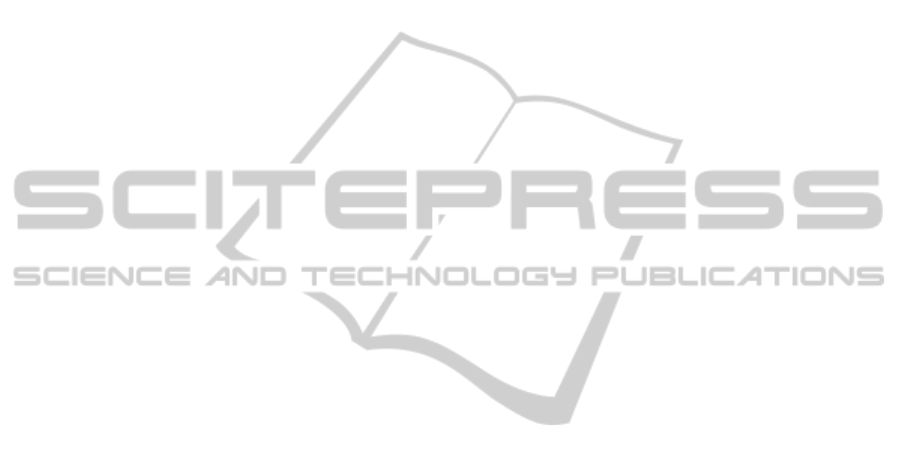
in this work achieves all the above criteria. It will
be interesting to apply this framework to sEMG/force
data collected from amputees. As future work, the
authors are investigating the possibility of a simulta-
neous regression-classification framework for finger
force control.
ACKNOWLEDGEMENTS
Authors T. Baldacchino and W. Jacobs would respec-
tively like to thank the Leverhulme Trust (130986)
and EPSRC (EP/K503149/1) for financial support.
REFERENCES
Atzori, M., Gijsberts, A., Heynen, S., Hager, A.-G. M., De-
riaz, O., van der Smagt, P., Castellini, C., Caputo,
B., and M
¨
uller, H. (2012). Building the NINAPRO
database: A resource for the biorobotics community.
In The Fourth IEEE RAS/EMBS International Conf on
Biomedical Robotics and Biomechatronics.
Baldacchino, T., Cross, E. J., Worden, K., and Rowson, J.
(Under Review, 2014a). Variational Bayesian mixture
of experts models and sensitivity analysis for nonlin-
ear dynamical systems. Mechanical Systems and Sig-
nal Processing.
Baldacchino, T., Worden, K., and Rowson, J. (2014b). Sen-
sitivity analysis using Bayesian mixture of experts for
a bifurcating Duffing oscillator. In International Conf
on Noise and Vibration (ISMA) 2014, Leuven.
Beal, M. J. and Ghahramani, Z. (2003). The variational
Bayesian EM algorithm for incomplete data: with ap-
plication to scoring graphical model structures. In
Bayesian Statistics 7. Oxford University Press.
Castellini, C. and K
˜
oiva, R. (2012). Using surface elec-
tromyography to predict single finger forces. In
The Fourth IEEE RAS/EMBS International Conf on
Biomedical Robotics and Biomechatronics.
Castellini, C. and van der Smagt, P. (2009). Surface EMG
in advanced hand prosthetics. Biological Cybernetics,
100(1):35–47.
Farrell, T. R. and Weir, R. F. (2008). A comparison of
the effects of electrode implantation and targeting on
pattern classification accuracy for prosthesis control.
IEEE Trans on Bio-Medical Electronics, 55(9):2198–
2211.
Ferguson, S. and Dunlop, G. (2002). Grasp recognition
from myoelectric signals. In Proceedings of the Aus-
tralasian Conf on robotics and automation.
Gijsberts, A., Atzori, M., Castellini, C., M
¨
uller, H., and Ca-
puto, B. (2014). The movement error rate for eval-
uation of machine learning methods for sEMG-based
hand movement classification. IEEE Trans on Neural
Systems and Rehabilitation Engineering, 22(4):735–
744.
Hahne, J. M., mann, F. B., Jiang, N., Rehbaum, H., Fa-
rina, D., M
¨
uller, F. C. M. K.-R., and Parra, L. C.
(2014). Linear and non-linear regression techniques
for simultaneous and proportional myoelectric con-
trol. IEEE Trans on Neural Systems and Rehabilita-
tion Engineering.
Jacobs, R. A., Jordan, M. I., Nowlan, S. J., and Hinton, G. E.
(1991). Adaptive mixtures of local experts. Neural
Computation, 3:79–87.
Lebedev, M. A. and Nicolelis, M. A. (2006). Brainmachine
interfaces: past, present and future. Trends in Neuro-
sciences, 29(9):536–546.
Muceli, S. and Farina, D. (2012). Simultaneous and pro-
portional estimation of hand kinematics from EMG
during mirrored movements at multiple degrees-of-
freedom. IEEE Trans on Neural Systems and Reha-
bilitation Engineering, 20(3):371–378.
Peleg, D., Braiman, E., Yom-Tov, E., and Inbar, G. (2002).
Classification of finger activation for use in a robotic
prosthesis arm. IEEE Trans on Neural Systems and
Rehabilitation Engineering, 10(4):290–293.
Saridis, G. N. and Gootee, T. P. (1982). EMG pattern analy-
sis and classification for a prosthetic arm. IEEE Trans
on Biomedical Engineering, 29(6):403–412.
Ueda, N. and Ghahramani, Z. (2002). Bayesian model
search for mixture models based on optimizing vari-
ational bounds. Neural Networks, 15:1223–1241.
Yuksel, S. E., Wilson, J. N., and Gader, P. D. (2012). Twenty
years of mixture of experts. IEEE Trans. Neural Netw.
Learning Syst., 23(8):1177–1193.
BIOSIGNALS2015-InternationalConferenceonBio-inspiredSystemsandSignalProcessing
276