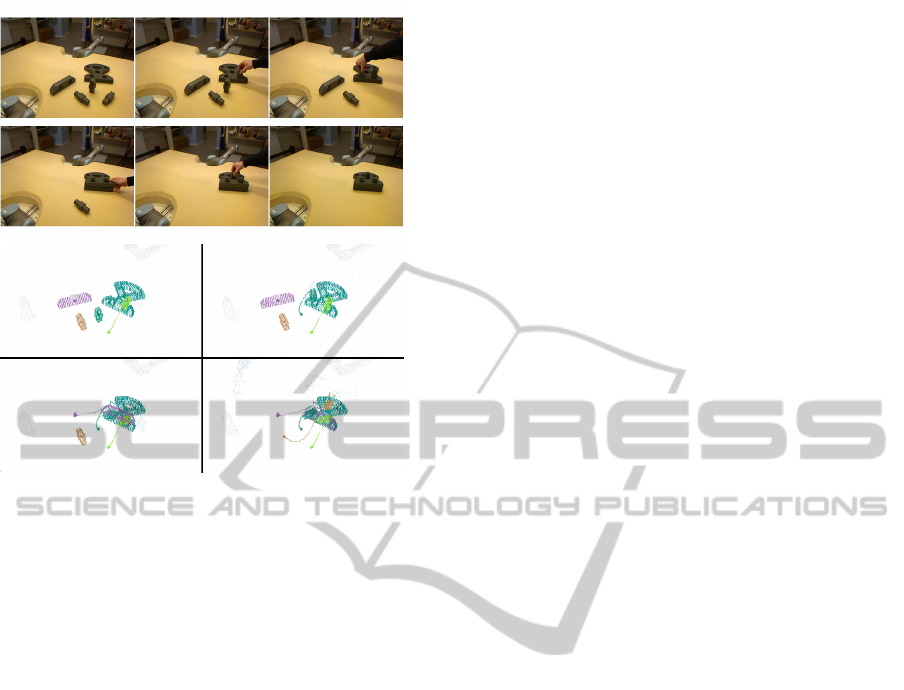
Figure 9: Tracker results for a complex assembly sequence.
The bottom images show the tracked object models, rep-
resented by point clouds, at the key-frames which are also
shown in the four intermediate top images.
shows a higher degree of robustness to disturbances
in the form of both partial and total occlusions.
ACKNOWLEDGEMENTS
This work has been funded by the EU project ACAT
(ICT-2011.2.1, grant agreement number 600578) and
the DSF project patient@home.
REFERENCES
Aksoy, E. E., Abramov, A., D
¨
orr, J., Ning, K., Dellen,
B., and W
¨
org
¨
otter, F. (2011). Learning the semantics
of object-action relations by observation. The Inter-
national Journal of Robotics Research, 30(10):1229–
1249.
Aldoma, A., Tombari, F., Di Stefano, L., and Vincze, M.
(2012). A global hypotheses verification method for
3d object recognition. In Computer Vision–ECCV
2012, pages 511–524. Springer.
Besl, P. and McKay, N. D. (1992). A method for registration
of 3-d shapes. PAMI, 14(2):239–256.
Buch, A., Kraft, D., Kamarainen, J.-K., Petersen, H.,
and Kr
¨
uger, N. (2013). Pose estimation using local
structure-specific shape and appearance context. In
ICRA, pages 2080–2087.
Choi, C. and Christensen, H. (2013). Rgb-d object track-
ing: A particle filter approach on gpu. In Intelligent
Robots and Systems (IROS), 2013 IEEE/RSJ Interna-
tional Conference on.
Drost, B., Ulrich, M., Navab, N., and Ilic, S. (2010). Model
globally, match locally: Efficient and robust 3d object
recognition. In Computer Vision and Pattern Recogni-
tion (CVPR), 2010 IEEE Conference on, pages 998–
1005. IEEE.
Fox, D. (2003). Adapting the sample size in particle filters
through kld-sampling. The International Journal of
Robotics Research, 22(12):985–1003.
Kootstra, G., Popovic, M., Jørgensen, J., Kuklinski, K., Mi-
atliuk, K., Kragic, D., and Kruger, N. (2012). En-
abling grasping of unknown objects through a syner-
gistic use of edge and surface information. The Inter-
national Journal of Robotics Research, 31(10):1190–
1213.
Mustafa, W., Pugeault, N., and Kr
¨
uger, N. (2013). Multi-
view object recognition using view-point invariant
shape relations and appearance information. In ICRA,
pages 4230–4237.
Newcombe, R. A., Davison, A. J., Izadi, S., Kohli, P.,
Hilliges, O., Shotton, J., Molyneaux, D., Hodges, S.,
Kim, D., and Fitzgibbon, A. (2011). Kinectfusion:
Real-time dense surface mapping and tracking. In IS-
MAR, pages 127–136. IEEE.
Papon, J., Kulvicius, T., Aksoy, E., and Worgotter, F.
(2013). Point cloud video object segmentation using
a persistent supervoxel world-model. In IROS, pages
3712–3718.
Ramirez-Amaro, K., Beetz, M., and Cheng, G. (2014). Au-
tomatic Segmentation and Recognition of Human Ac-
tivities from Observation based on Semantic Reason-
ing. In IEEE/RSJ International Conference on Intelli-
gent Robots and Systems.
Ren, C., Prisacariu, V., Murray, D., and Reid, I. (2013).
Star3d: Simultaneous tracking and reconstruction
of 3d objects using rgb-d data. In Computer Vi-
sion (ICCV), 2013 IEEE International Conference on,
pages 1561–1568.
Rusu, R. B. and Cousins, S. (2011). 3D is here: Point Cloud
Library (PCL). In ICRA, Shanghai, China.
Savarimuthu, T., Liljekrans, D., Ellekilde, L.-P., Ude, A.,
Nemec, B., and Kruger, N. (2013). Analysis of hu-
man peg-in-hole executions in a robotic embodiment
using uncertain grasps. In Robot Motion and Control
(RoMoCo), 2013 9th Workshop on, pages 233–239.
Tombari, F., Salti, S., and Di Stefano, L. (2010). Unique
signatures of histograms for local surface description.
In ECCV, pages 356–369.
Yang, Y., Ferm
¨
uller, C., and Aloimonos, Y. (2013). De-
tection of manipulation action consequences (mac).
In Computer Vision and Pattern Recognition, pages
2563–2570.
Zhang, B., Wang, J., Rossano, G., Martinez, C., and Kock,
S. (2011). Vision-guided robot alignment for scalable,
flexible assembly automation. In ROBIO, pages 944–
951.
AnOnlineVisionSystemforUnderstandingComplexAssemblyTasks
461