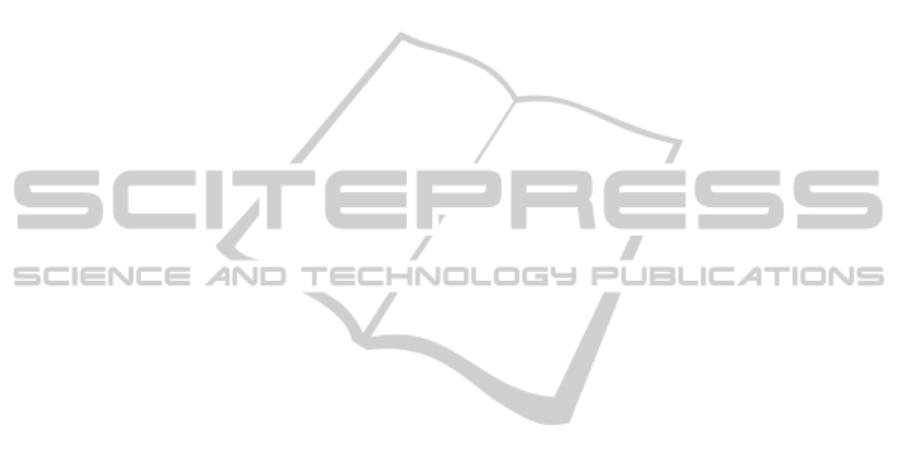
Systems (HCKMS) require a multi-disciplinary
approach to meet an organization’s need to innovate
and thrive. Gotz, Stavropoulos, Sun and Wang
describe the use of a predictive risk-assessment
analytics system that analyzes large volume of
heterogeneous medical data to provide various forms
of risk analytics across the patient population. By
applying text and data mining techniques, pattern
recognition, and cognitive computing techniques on
large volumes of clinical and genomic data, smarter
health informatics and analytics can drive
knowledge discovery that have a direct impact on
patients at the point of care (Gotz et al, 2012).
Polanyi described tacit knowledge as implicit
information that is difficult to capture linguistically.
(Polanyi, 1966) This challenge is further exacerbated
when attempting to automate the capture and process
of healthcare tacit knowledge. Nonaka and Takeuchi
created a variety of models as a means of capturing
and communicating (Nonaka and Takeuchi, 1995).
Healthcare organizations are under constant
pressure to cut costs while improving patient care,
clinical outcomes, and reducing hospital re-
admission rates. IBM’s Patient Care and Insights
solution offers the ability to analyze a wealth of
structured and unstructured data from medical
records and by using similarity analytics can auto-
select a cohort of clinically similar patients for
further analysis (Gourlay, 2002). The solution
leverages healthcare annotators, natural language
processing, and analytics to track trends, patterns,
anomalies, and deviations; it produces a
personalized proactive care solution that can reduce
hospital readmission rates, predict the onset of
ailments based on a patient’s social and behavioral
patterns, ensure drug treatment effectiveness, and
help in aligning physician and care teams to patients.
With the explosion of data in terms of volume,
velocity, variety, and veracity, it is imperative and
time critical to have the ability to analyze a high
number of healthcare data points and correlate the
data in real time. Clinicians need timely and accurate
information so that they can make real time
decisions and proactively intervene to save human
lives, shorten hospital stays, reduce hospital
readmission rates, and improve the overall quality of
care. Medical devices that monitor patients’ health
conditions can generate streams of data related to
heart rate, blood oxygen saturation, and respiratory
rates. The ability to fuse real-time streaming data
from various sources and apply analytics can help
address and prevent many life threatening conditions.
Health Informatics that leverages and processes data
streams in real time with an advanced analytic
engine can provide the ability to quickly ingest,
analyze and correlate information from thousands of
real-time resources to predict and save lives in a
timely fashion across intensive care units (Dollard
2013). Big Data and analytics will play a major role
in the future of healthcare and will have a direct
impact on the medical practitioners and their care of
patients. With the onset of aging population,
managing chronic illness and conditions is becoming
a major factor in healthcare. Providers are under
pressure to deliver better and safer care at lower cost
with increased transparencies in the quality of care
and outcomes.
At the same time, payers including health
insurance companies and the US Government are
under increased pressure to monitor the accuracy of
claims and quickly detect a fraudulent claim.
Historically, a pure ‘pay and chase’ model has been
used to combat healthcare fraud. This model is
expensive to execute and thus not sustainable. A
more reliable solution would be to identify
fraudulent claims on entry by parsing the claim text,
looking for trends and anomalies that depict
fraudulent patterns, and flagging these claims early
in the payment workflow cycle for review and
analysis. Here cognitive computing in the form of
Watson Policy Advisor can play a significant role in
ingesting claim policies and guidelines. Going
through domain adaptation and training to detect
fraudulent patterns can result in significant savings
in cost, time, and resources for the payer, provider,
and the patient.
Healthcare providers are expected to be current
on the latest medical research and advancements.
Physicians are expected to be aware of the 360 view
of the patient’s medical history, allergies, drug
treatments, and clinical and lab work when they
walk into the consulting room. This puts tremendous
pressure on the practitioners’ time as they need to
not only discern their patient’s history and analyze
test results, but also maintain a current medical
knowledge base. (David Dugdale et.al., 1999).
Search is a fundamental element of a HCKMS.
With the growth of multimedia over the past decade,
new search and analytics systems have evolved that
provide the ability to search through pre-processed
metadata and automatically analyze files for the
search criteria (e.g., image recognition, speech-to-
text). These advanced search techniques enable the
capture of tacit knowledge in non-linguistic forms.
Gourlay’s knowledge management (KM) framework
stresses the value of non-verbal modes of
information, such as behaviors and processes, to
convey a variety of perspectives (Gourlay, 2002.
HEALTHINF2015-InternationalConferenceonHealthInformatics
526