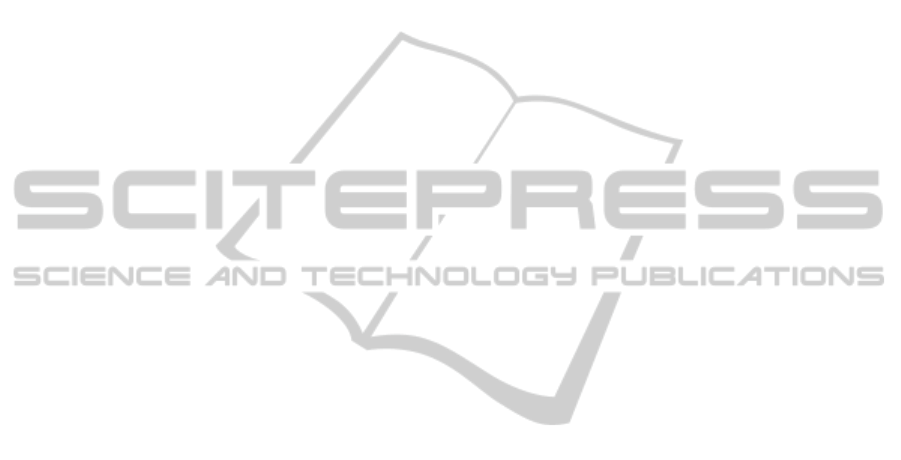
features).
The immediate work is to extend the proposed de-
scriptor FLDP to use higher order membership func-
tions such as Gaussian and Trapezoidal functions. Fu-
ture work will focus on the use of the neutrosophic
logic instead of fuzzy logic. The neutrosophic logic
is a general framework for unification of many ex-
isting logics including fuzzy logic. Thus, principles
such as neutrosophic sets and neutrosophic probabil-
ity will be used instead of fuzzy sets and the degree
of membership.
ACKNOWLEDGEMENTS
This work was partly supported by the Spanish Gov-
ernment through project TIN2012-37171-C02-02.
REFERENCES
Abdel-Nasser, M., Puig, D., and Moreno, A. (2014). Im-
provement of mass detection in breast X-ray images
using texture analysis methods. In Artificial Intelli-
gence Research and Development: Proceedings of the
17th International Conference of the Catalan Associ-
ation for Artificial Intelligence, Barcelona, Spain, vol-
ume 269, pages 159–168. IOS Press.
Ahonen, T. and Pietik
¨
ainen, M. (2007). Soft histograms
for local binary patterns. In Proceedings of the
Finnish signal processing symposium, FINSIG, vol-
ume 5, page 1.
Chang, C.-C. and Lin, C.-J. (2011). Libsvm: a library for
support vector machines. ACM Transactions on Intel-
ligent Systems and Technology (TIST), 2(3):27.
Chen, J., Kellokumpu, V., Zhao, G., and Pietik
¨
ainen, M.
(2013). Rlbp: Robust local binary pattern. In Proc. of
the British Machine Vision Conference (BMVC 2013),
Bristol, UK.
DeSantis, C., Ma, J., Bryan, L., and Jemal, A. (2014).
Breast cancer statistics, 2013. CA: A Cancer Journal
for Clinicians, 64(1):52–62.
Garc
´
ıa-Manso, A., Garc
´
ıa-Orellana, C., Gonz
´
alez-Velasco,
H., Gallardo-Caballero, R., and Mac
´
ıas-Mac
´
ıas, M.
(2013). Study of the effect of breast tissue density on
detection of masses in mammograms. Computational
and Mathematical Methods in Medicine, 2013.
Gonzalez, R. C. and Woods, R. E. (2002). Digital image
processing. Prentice hall Upper Saddle River, New
Jersey, USA.
Hanley, J. A. and McNeil, B. J. (1982). The meaning and
use of the area under a receiver operating characteris-
tic (ROC) curve. Radiology, 143(1):29–36.
Jabid, T., Kabir, M. H., and Chae, O. (2010). Facial expres-
sion recognition using local directional pattern (LDP).
In IEEE International Conference on Image Process-
ing (ICIP), pages 1605–1608.
Keramidas, E., Iakovidis, D., and Maroulis, D. (2011).
Fuzzy binary patterns for uncertainty-aware texture
representation. Electronic Letters on Computer Vision
and Image Analysis, 10(1):63–78.
Lokate, M., Kallenberg, M. G., Karssemeijer, N., Van den
Bosch, M. A., Peeters, P. H., and Van Gils, C. H.
(2010). Volumetric breast density from full-field
digital mammograms and its association with breast
cancer risk factors: a comparison with a threshold
method. Cancer Epidemiology Biomarkers & Preven-
tion, 19(12):3096–3105.
Mohamed, M., Rashwan, H., Mertsching, B., Garcia, M.,
and Puig, D. (2014). Illumination-robust optical
flow using local directional pattern. IEEE Transac-
tions on Circuits and Systems for Video Technology,
24(9):1499–1508.
Ojala, T., Pietikainen, M., and Maenpaa, T. (2002). Mul-
tiresolution gray-scale and rotation invariant texture
classification with local binary patterns. IEEE Trans-
actions on Pattern Analysis and Machine Intelligence,
24(7):971–987.
Oliver, A., Llad
´
o, X., Freixenet, J., and Mart
´
ı, J. (2007).
False positive reduction in mammographic mass de-
tection using local binary patterns. In Medical Im-
age Computing and Computer-Assisted Intervention
(MICCAI), pages 286–293. Springer.
Pomponiu, V., Hariharan, H., Zheng, B., and Gur, D.
(2014). Improving breast mass detection using his-
togram of oriented gradients. In SPIE Medical Imag-
ing, pages 90351R–90351R. International Society for
Optics and Photonics.
Ramirez Rivera, A., Castillo, R., and Chae, O. (2013). Lo-
cal directional number pattern for face analysis: Face
and expression recognition. IEEE Transactions on Im-
age Processing, 22(5):1740–1752.
Shi, X., Cheng, H., Hu, L., Ju, W., and Tian, J. (2010).
Detection and classification of masses in breast ultra-
sound images. Digital Signal Processing, 20(3):824–
836.
Soltanian, H., Rafiee-Rad, F., and Pourabdollah-Nejad D,
S. (2004). Comparison of multiwavelet, wavelet,
haralick, and shape features for microcalcification
classification in mammograms. Pattern Recognition,
37(10):1973–1986.
Suckling, J., Parker, J., Dance, D., Astley, S., Hutt, I., Bog-
gis, C., Ricketts, I., Stamatakis, E., Cerneaz, N., Kok,
S.-L., et al. (1994). The mammographic image analy-
sis society digital mammogram database. In 2nd Inter-
national Workshop on Digital Mammography, pages
375–378. Excerta Medica.
Zadeh, L. A. (1965). Fuzzy sets. Information and control,
8(3):338–353.
Zheng, Y. (2010). Breast cancer detection with Ga-
bor features from digital mammograms. Algorithms,
3(1):44–62.
VISAPP2015-InternationalConferenceonComputerVisionTheoryandApplications
394