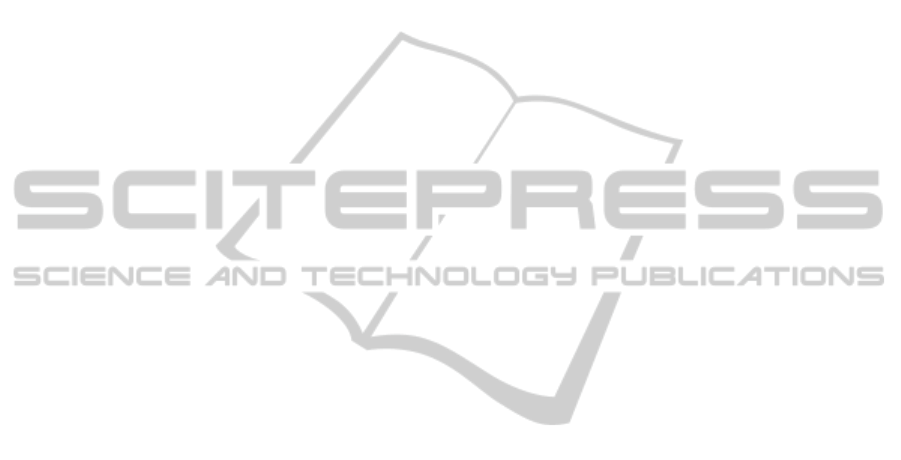
5 CONCLUSION AND FUTURE
WORK
In this paper, we presented HSRM-Tracking, a
method for robustly estimating and tracking the pose
of multiple infrared markers with a single monocu-
lar camera. Thereby, individual markers can be cor-
rectly recognized in each single camera frame and
distinguished based on the cross ratio of four collinear
LEDs. Our evaluation results show that HSRM-
Tracking is able to precisely capture fine and rapid
movement up to 1000 Hz in a large area neglecting
bandwidth limitations of current cameras.
The proposed method could easily be adapted for
use in a multi-camera system where each camera runs
in parallel in a separate tracking thread. Thus, cam-
eras with different frame rates could be combined to
track the markers asynchronously and contribute to
a synchronized result whenever a new measurement
is available, making camera synchronization unnec-
essary. Being able to estimate the marker pose from a
single camera would also vastly increase the track-
ing volume of a multi camera setup and could be
used in conjunction with stereo methods, whenever
the marker is visible in more than one camera. Such a
setup would also benefit from the LED identification
scheme, since the markers could be used in order to
dynamically calibrate the multi-camera system with-
out having to solve stereo correspondence problems.
REFERENCES
Faessler, M., Mueggler, E., Schwabe, K., and Scaramuzza,
D. (2014). A monocular pose estimation system based
on infrared LEDs. In IEEE International Conference
on Robotics and Automation (ICRA).
Fitzgerald, D., Foody, J., Kelly, D., Ward, T., Markham, C.,
McDonald, J., and Caulfield, B. (2007). Development
of a wearable motion capture suit and virtual reality
biofeedback system for the instruction and analysis of
sports rehabilitation exercises. In EMBS 2007, pages
4870–4874.
Herout, A., Szentandrasi, I., Zacharia, M., Dubska, M., and
Kajan, R. (2013). Five shades of grey for fast and
reliable camera pose estimation. In IEEE Conference
on Computer Vision and Pat. Rec., pages 1384–1390.
Lakemond, R., Fookes, C., and Sridharan, S. (2013).
Resection-intersection bundle adjustment revisited.
ISRN Machine Vision, 2013:8.
Levenberg, K. (1944). A method for the solution of cer-
tain non-linear problems in least squares. Quarterly
Journal of Applied Mathmatics, II(2):164–168.
Lourakis, M. A. and Argyros, A. (2009). SBA: A Software
Package for Generic Sparse Bundle Adjustment. ACM
Trans. Math. Software, 36(1):1–30.
MacKay, D. J. C. (2002). Information Theory, Inference
& Learning Algorithms. Cambridge University Press,
New York, NY, USA.
Marquardt, D. W. (1963). An algorithm for least-squares
estimation of nonlinear parameters. SIAM Journal on
Applied Mathematics, 11(2):431–441.
Naimark, L. and Foxlin, E. (2005). Encoded led system for
optical trackers. In Proceedings of the 4th IEEE/ACM
International Symposium on Mixed and Augmented
Reality, pages 150–153.
Nister, D. (2004). An efficient solution to the five-point
relative pose problem. IEEE Transactions on Pattern
Analysis and Machine Intelligence, 26(6):756–770.
Olson, E. (2011). AprilTag: A robust and flexible vi-
sual fiducial system. In Proceedings of the IEEE In-
ternational Conference on Robotics and Automation
(ICRA), pages 3400–3407. IEEE.
Ong, S. K. and Nee, A. (2004). Virtual Reality and
Augmented Reality Applications in Manufacturing.
Springer Verlag.
Ozuysal, M., Calonder, M., Lepetit, V., and Fua, P. (2010).
Fast keypoint recognition using random ferns. Pat-
tern Analysis and Machine Intelligence, IEEE Trans-
actions on, 32(3):448–461.
Prisacariu, V. and Reid, I. (2012). Pwp3d: Real-time seg-
mentation and tracking of 3d objects. International
Journal of Computer Vision, 98(3):335–354.
Schmaltz, C., Rosenhahn, B., Brox, T., and Weickert, J.
(2012). Region-based pose tracking with occlusions
using 3d models. Machine Vision and Applications,
23(3):557–577.
Schweighofer, G. and Pinz, A. (2006). Robust pose
estimation from a planar target. Pattern Analy-
sis and Machine Intelligence, IEEE Transactions on,
28(12):2024–2030.
Vito, L., Postolache, O., and Rapuano, S. (2014). Mea-
surements and sensors for motion tracking in motor
rehabilitation. Instrumentation Measurement Maga-
zine, IEEE, 17(3):30–38.
Wagner, D., Reitmayr, G., Mulloni, A., Drummond, T., and
Schmalstieg, D. (2008). Pose tracking from natural
features on mobile phones. In Proceedings of the 7th
IEEE/ACM International Symposium on Mixed and
Augmented Reality, pages 125–134.
Welch, G. and Foxlin, E. (2002). Motion tracking: No sil-
ver bullet, but a respectable arsenal. IEEE Comput.
Graph. Appl., 22(6):24–38.
Xu, C., Kuipers, B., and Murarka, A. (2009). 3d pose esti-
mation for planes. In ICCV Workshop on 3D Repre-
sentation for Recognition (3dRR-09).
Yang, H., Wang, F., Xin, J., Zhang, X., and Nishio, Y.
(2012). A robust pose estimation method for nearly
coplanar points. In Proceedings NCSP ’12., pages
345–348.
Zetu, D., Banerjee, P., and Thompson, D. (2000). Extended-
range hybrid tracker and applications to motion and
camera tracking in manufacturing systems. IEEE
Transactions on Robotics and Aut., 16(3):281–293.
High-SpeedandRobustMonocularTracking
471