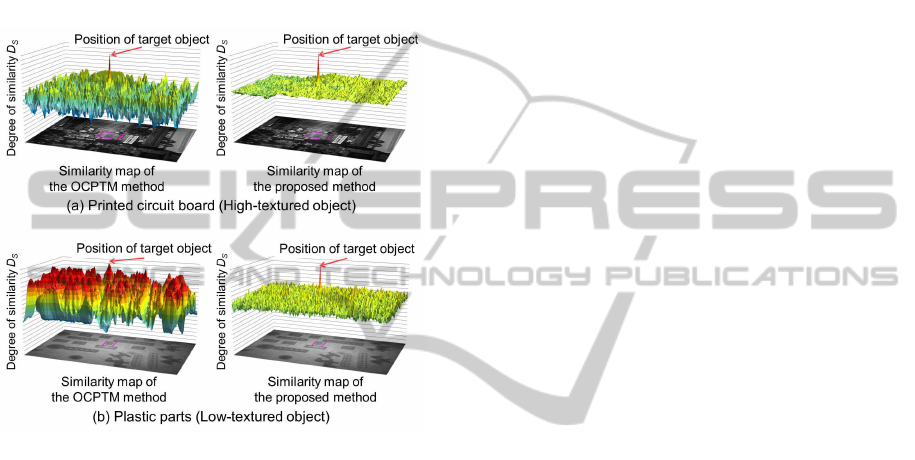
3.3 Similarity Map Analysis
We confirmed the effectiveness of the binarized gra-
dient features by analyzing the similarity map ob-
tained for the proposed method. The experimental
images used were images of the printed circuit board
and plastic parts shown in Figure 6. The compari-
son method used was the OCPTM method. Figure 7
shows the similarity maps obtained for the proposed
method and the OCPTM method.
Figure 7: Similarity maps obtained for the proposed method
and the OCPTM method.
In a high-textured object (the printed circuit
board), the similarity maps obtained by the two meth-
ods showed a sharp peak at the position of the target
object. However, in low-textured objects (the plas-
tic parts), the similarity map of the OCPTM method
showed a high degree of similarity in positions other
than the position of target object, while the score map
of the proposed method showed a high degree of sim-
ilarity only in the position of target object. These re-
sults confirmed the effectiveness of the binarized gra-
dient features.
4 CONCLUSION
We have proposed binarized gradient features that re-
flect the concavo-convexshape of an object and an ob-
ject detection method using these features. By using
the features in the matching process, we confirmed
that our method is able to achieve reliable object de-
tection even if a target object is low-textured. Ex-
periments using 200 actual images confirmed that our
method achievesa 97.5% recognition success rate and
a 4.62 sec processing time. In future work, we will at-
tempt to even further speed up the processing time.
REFERENCES
Akizuki, S. and Hashimoto, M. (2013). Robust match-
ing for low-texture images based on co-occurrence of
geometry-optimized pixel patterns. In Proc. QCAV,
pages 113–116.
Barrow, H., Tenenbaum, J., Bolles, R., and Wolf, H. (1977).
Parametric correspondence and chamfer matching:
Two new techniques for image matching. In Proc. of
IJCAI, pages 659–663.
Bay, H., Tuytelaars, T., and Gool, L. (2006). Surffspeeded
up robust features. In Proc. of ECCV, pages 404–417.
Drost, B. and Ilic, S. (2012). 3d object detection and local-
ization using multimodal point pair features. In Proc.
3DIMPVT, pages 9–16.
Hashimoto, M., Fujiwara, T., Koshimizu, H., Okuda, H.,
and Sumi, K. (2010). Extraction of unique pix-
els based on co-occurrence probability for high-speed
template matching. In Proc. of ISOT, pages 1–6.
Hinterstoisser, S., Cagniart, C., Ilic, S., Sturm, P., Navab,
N., Fua, P., and Lepetit, V. (2012). Gradient response
maps for real-time detection of texture-less objects. In
IEEE Trans. on PAMI, pages 876–888.
Hinterstoisser, S., Lepetit, V., Ilic, S., Fua, P., and Navab,
N. (2010). Dominant orientation templates for real-
time detection of texture-less objects. In Proc. CVPR,
pages 2257–2264.
Lowe, D. (2004). Distinctive image features from scale-
invariant keypoints. In IJCV, volume 60, pages 91–
110.
Raskar, R., Tan, K., Feris, R., Yu, J., and M.Turk (2004).
Non-photorealistic camera: Depth edge detection and
stylized rendering using multi-flash imaging. In ACM
Trans. on Graphics, volume 23, pages 679–688.
Rublee, E., Rabaud, V., Konolige, K., and Bradski, G.
(2011). ORB : An efficient alternative to SIFT or
SURF. In Proc. of ICCV, pages 2564–2571.
Tombari, F., Franchi, A., and Stefano, L. D. (2013). Bold
features to detect texture-less objects. In Proc. of
ICCV, pages 1265–1272.
VISAPP2015-InternationalConferenceonComputerVisionTheoryandApplications
264