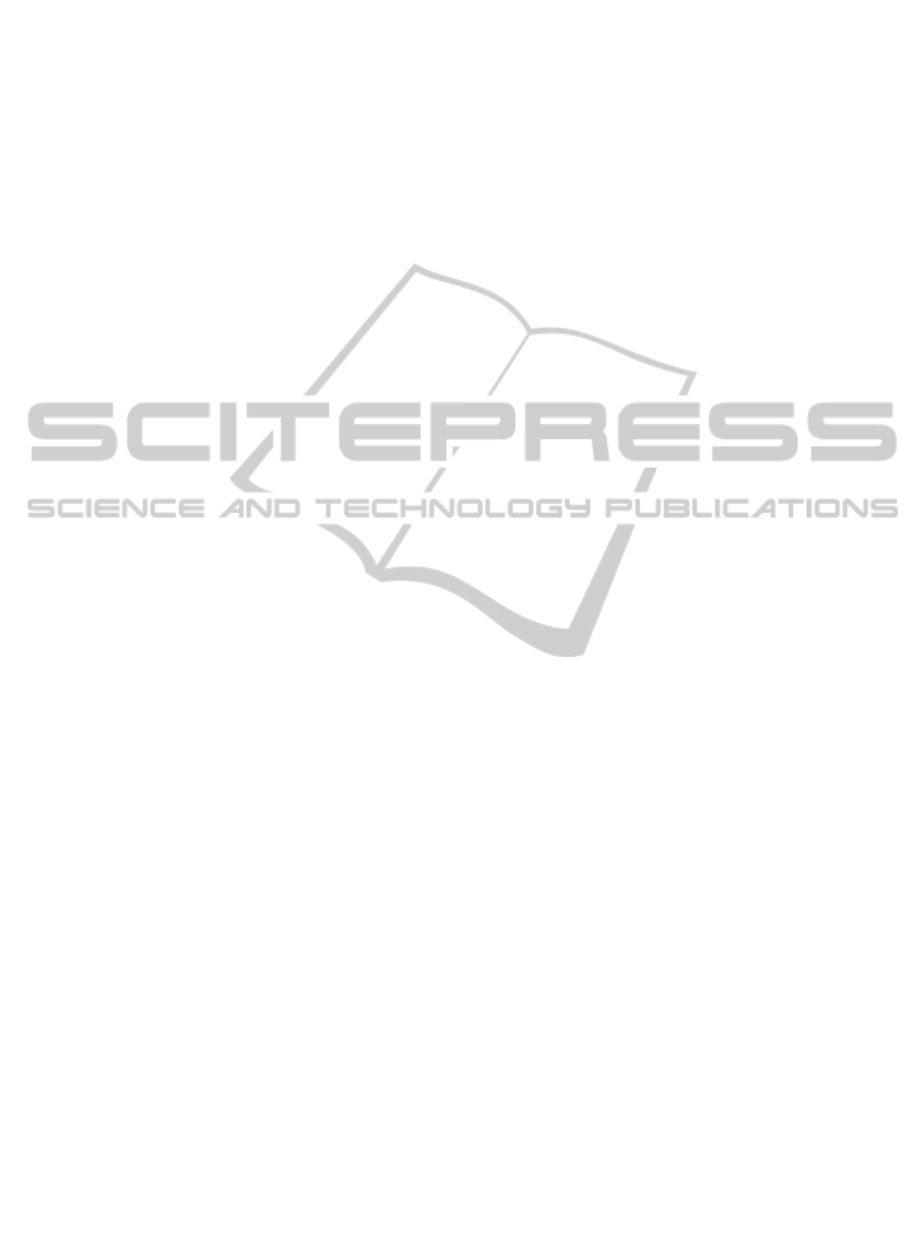
produced a correct identity instance in 75% of cases,
in comparison to 61% and 54% for TPS-RPM and
SC methods, respectively; confirming the usefulness
of saliency-biased registration in animal biometrics.
8 CONCLUSIONS
A method for ordering points in a set on a measure
of distinguishability, contextual saliency, has been
introduced in this paper. Ordering on this basis is
shown to be tolerant of noise and perspective
transformation, as well as be predictive of
correspondence, in synthetic experiments.
This information is leveraged in an iterative non-
rigid registration algorithm, Ψ-Match. A case study
on a difficult real-world manta ray data set found
improved performance for a recognition system
using Ψ-Match registration in comparison to the
same setup using either shape context (Belongie et
al., 2002) or TPS-RPM (Chui & Rangarajan, 2003)
registration algorithms.
ACKNOWLEDGEMENTS
We thank Fit4Change Ltd for funding this work. We
would also like to acknowledge Guy Stevens and the
Manta Trust for image provision, and Mike Preager
for help with ground truth annotations.
REFERENCES
Ballard, D. H. 1981. Generalizing the Hough transform to
detect arbitrary shapes. Pattern recognition, 13(2),
111-122.
Belongie, S., Malik, J., & Puzicha, J. 2002. Shape
matching and object recognition using shape
contexts. Pattern Analysis and Machine Intelligence,
IEEE Transactions on, 24(4), 509-522.
Chui, H., & Rangarajan, A. 2003.A new point matching
algorithm for non-rigid registration.Computer Vision
and Image Understanding, 89(2), 114-141.
Dubuisson, M. P., & Jain, A. K. 1994. A modified
Hausdorff distance for object matching.In Pattern
Recognition, 1994. Vol. 1-Conference A: Computer
Vision & Image Processing., Proceedings of the 12th
IAPR International Conference on (Vol. 1, pp. 566-
568).
Gamble, L., Ravela, S., & McGarigal, K. 2008. Multi
scale features for identifying individuals in large
biological databases: an application of pattern
recognition technology to the marbled salamander
Ambystomaopacum. Applied Ecology, 45(1), 170-180.
Kitchen-Wheeler, A. M. 2011. Visual identification of
individual manta ray (Manta alfredi) in the Maldives
Islands, Western Indian Ocean. Marine Biology
Research, 6(4), 351-363.
Kühl, H. S., & Burghardt, T. 2013. Animal biometrics:
quantifying and detecting phenotypic
appearance. Trends in ecology & evolution, 28(7),
432-441.
Metaxas, D., Koh, E., & Badler, N. I. 1997. Multi-level
shape representation using global deformations and
locally adaptive finite elements.International journal
of computer vision, 25(1), 49-61.
Munkres, J. 1957. Algorithms for the assignment and
transportation problems. Journal of the Society for
Industrial and Applied Mathematics, 5, 32-38.
Myronenko, A., & Song, X. 2010. Point set registration:
Coherent point drift. Pattern Analysis and Machine
Intelligence, IEEE Transactions on, 32(12), 2262-
2275.
Rangarajan, A., Chui, H., & Bookstein, F. L. 1997. The
softassignprocrustesmatching
algorithm.In Information Processing in Medical
Imaging. Springer Berlin Heidelberg.
Rueckert, D., & Schnabel, J. A. 2011. Medical image
registration. In Biomedical Image Processing (pp.
131-154).Springer Berlin Heidelberg.
Turing, A. M. 1952. The chemical basis of
morphogenesis. Philosophical Transactions of the
Royal Society of London. Series B, Biological
Sciences, 237(641), 37-72.
Wahba, G. (1990). Spline models for observational
data (Vol. 59). Siam.
Yu, G., & Morel, J. M. 2011. ASIFT: an algorithm for
fully affine invariant comparison. Image Processing
OnLine, 1.
Zhang, Z. 1994. Iterative point matching for registration of
free-form curves and surfaces. International Journal
of Computer Vision, 13, 119-152.
Zheng, Y., & Doermann, D. 2006. Robust point matching
for nonrigid shapes by preserving local neighborhood
structures. Pattern Analysis and Machine Intelligence,
IEEE Transactions on, 28(4), 643-649.
VISAPP2015-InternationalConferenceonComputerVisionTheoryandApplications
410