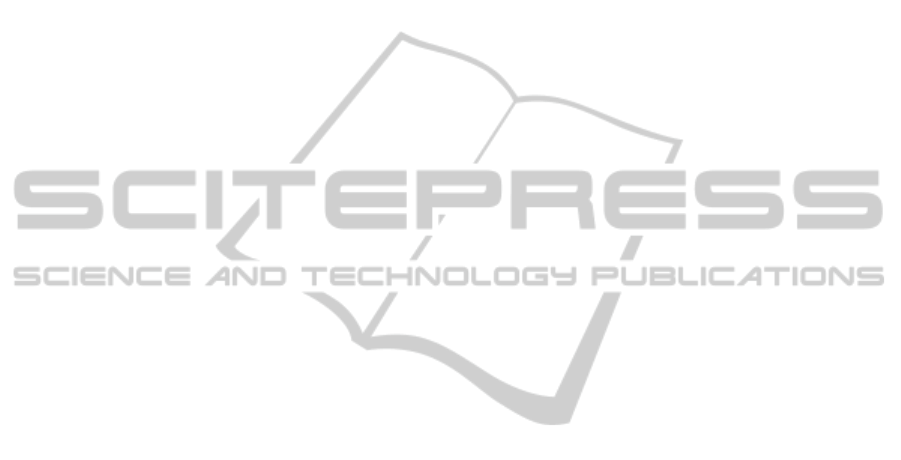
raw exams data instead of combined scores, adding
potentially important measurements, enlightenment
of the data by expert knowledge such as differing
questions to the different cognitive function domain
measured could all help in creating more subtle and
fine clusters of patient’s disease presentation. From
a statistical point of view, different clustering
procedures and/or different selection procedures
may yield better results under different settings, an
issue we have not started to address at all.
We believe that the attempt to predict from very
specific potential biomarker is futile. The route we
have taken is to predict more subtle disease
manifestation classes. Such a process needs further
exploration but has the potential to fit a small
biomarker arrow to the clinical bull’s eye.
In many studies and definitely in the ADNI
study a vast amount of measurable information is
collected. Is it enough? The tacit knowing held and
applied by proficient practitioners represents a
valuable form of clinical knowledge, which has been
acquired through experience, and which should be
investigated, shared, and contested (Malterud 2001).
In clinical work, tacit knowing constitutes an
important part of diagnostic reasoning and judgment
of medical conditions. We made an effort to
incorporate this knowledge into the process so that a
valuable aspect of analysis and interpretation of the
results could be added. Further exploration is needed
of both the data nuances and methods, before trying
to scale to the much harder problem associated with
regular hospital data. We do believe that the strategy
we have outlined in this work is capable of
achieving that.
ACKNOWLEDGEMENTS
**Data used in preparation of this article were
obtained from the Alzheimer’s Disease Neuro-
imaging Initiative (ADNI) database (adni.loni.usc.
edu). As such, the investigators within the ADNI
contributed to the design and implementation of
ADNI and/or provided data but did not participate in
analysis or writing of this report. A complete listing
of ADNI investigators can be found
at:http://adni.loni.usc.edu/wpontent/uploads/how_to
_apply/ADNI_Acknowledgement_List.pdf
The research leading to these results has received
funding from the European Union Seventh
Framework Programme (FP7/2007-2013) under
grant agreement no. 604102 (Human Brain Project).
REFERENCES
American Psychiatric Association. (2013). DSM-5 criteria
for major neurocognitive disorder due to AD (Fifth
Edit.). Arlington, VA.
Sonnen, J. A., Montine, K. S., Quinn, J. F., Kaye, J. A.,
Breitner, J. C. S., & Montine, T. J. (2008). Biomarkers
for cognitive impairment and dementia in elderly
people. Lancet Neurology, 7(8), 704–14. doi:10.1016/
S1474-4422(08)70162-5.
Evans, M. C., Barnes, J., Nielsen, C., Kim, L. G., Clegg,
S. L., Blair, M., Fox, N. C. (2010). Volume changes in
Alzheimer’s disease and mild cognitive impairment:
cognitive associations. European Radiology, 20(3),
674–82. doi:10.1007/s00330-009-1581-5.
Gupta, V. B., Laws, S. M., Villemagne, V. L., Ames, D.,
Bush, A. I., Ellis, K. A., Martins, R. N. (2011).
Plasma apolipoprotein e and Alzheimer disease risk:
The AIBL study of aging. Neurology, 76(12), 1091–
1098.
Hinrichs, C., Singh, V., Xu, G., & Johnson, S. C. (2011).
Predictive markers for AD in a multi-modality
framework: An analysis of MCI progression in the
ADNI population. NeuroImage, 55(2), 574–589.
Kohannim, O., Hua, X., Hibar, D. P., Lee, S., Chou, Y.-
Y., Toga, A. W., Thompson, P. M. (2010). Boosting
power for clinical trials using classifiers based on
multiple biomarkers. Neurobiology of Aging, 31(8),
1429–42. doi: 10.1016/j.neurobiolaging.2010.04.022.
Langbaum, J. B. S., Chen, K., Lee, W., Reschke, C.,
Fleisher, A. S., Alexander, G. E., Reiman, E. M.
(2010). categorial and correlational analyses of
baseline flourodeoxyglucose positron emission
tomography images from the Alzheimer's disease
NeuroImage, 45(4), 1107–1116. doi:10.1016/
j.neuroimage.2008.12.072.
Liaw, A., & Wiener, M. (2002). Classification and
Regression by randomForest. R News, 2(December),
18–22.
Maechler, M., Rousseeuw, P., Struyf, A., Hubert, M., &
Hornik, K. (2013). Cluster Analysis Basics and
Extensions. R package version 1.14.4. CRAN.
Retrieved from http://cran.r-project.org/web/packages/
cluster/index.html.
Malterud, K. (2001). The art and science of clinical
knowledge: evidence beyond measures and numbers.
Lancet, 358(9279), 397–400. doi:10.1016/S0140-6736
(01)05548-9.
R Core Team. (n.d.). R: A language and environment for
statistical computing. Retrieved from http://www.r-
project.org/
Revelle, W. (2010). psych: Procedures for psychological,
psychometric, and personality research. Northwestern
University: Evanston, Illinois, 0–90.
Shadlen, Marie-Florence, MD; Larson, Eric B, MD, M.
(2014). UpToDate: Evaluation of cognitive
impairment and dementia. Retrieved from http://www.
uptodate.com/contents/evaluation-of-cognitive-
impairment-and-dementia.
HEALTHINF2015-InternationalConferenceonHealthInformatics
572