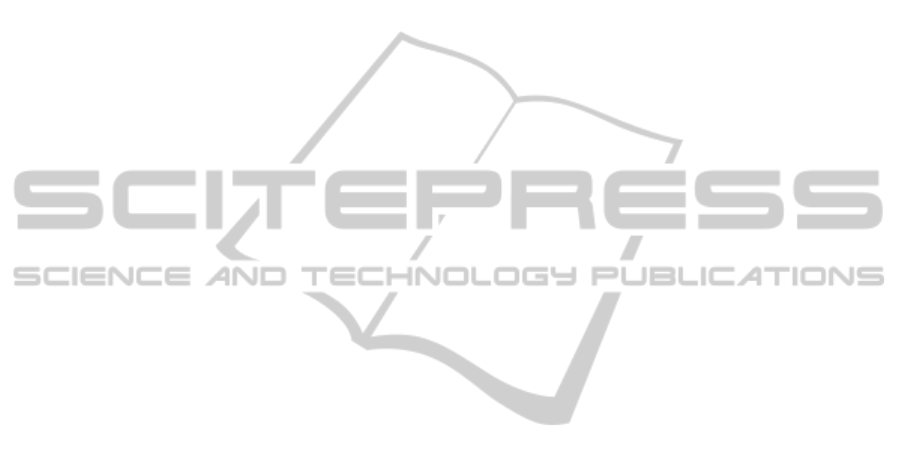
stroke onset, varies significantly among the three
clusters. This provides additional evidence that
further thorough analysis of these clusters from a
medical point of view may lead to better
understanding of stroke physiology and more
informed management of stroke patients.
Furthermore, the information from these clusters
may be utilized to address other research problems,
such as the construction of computational models for
identifying people at risk of stroke, and for
predicting the outcome of patients after stroke.
REFERENCES
Aluru, V., Lu, Y., Leung, A., Verghese, J. and Raghavan,
P., 2014. Effect of auditory constraints on motor
performance depends on stage of recovery post-stroke.
Frontiers in neurology, 5.
Banfield, J. D. and Raftery, A. E., 1993. Model-based
Gaussian and non-Gaussian clustering. Biometrics,
pp.803-821.
Baumgartner, C., Gautsch, K., Böhm, C. and Felber, S.,
2005. Functional cluster analysis of CT perfusion
maps: a new tool for diagnosis of acute stroke?.
Journal of digital imaging, 18(3), pp.219-226.
Benjamini, Y. and Hochberg, Y., 1995. Controlling the
false discovery rate: a practical and powerful approach
to multiple testing. Journal of the Royal Statistical
Society. Series B (Methodological), pp. 289-300.
Brott, T., Adams, H. P., Olinger, C. P., Marler, J. R.,
Barsan, W. G., Biller, J., Spilker, J., Holleran, R.,
Eberle, R. and Hertzberg, V., 1989. Measurements of
acute cerebral infarction: a clinical examination
scale. Stroke, 20(7), pp.864-870.
Bruehl, S., Lofland, K. R., Semenchuk, E. M., Rokicki, L.
A. and Penzien, D. B., 1999. Use of Cluster Analysis
to Validate IHS Diagnostic Criteria for Migraine and
Tension Type Headache. Headache: The Journal of
Head and Face Pain, 39(3), pp.181-189.
De Haan, R. J., Limburg, M., Van der Meulen, J. H. P.,
Jacobs, H. M. and Aaronson, N. K., 1995. Quality of
life after stroke impact of stroke type and lesion
location. Stroke, 26(3), pp.402-408.
Dempster, A. P., Laird, N. M. and Rubin, D. B., 1977.
Maximum likelihood from incomplete data via the EM
algorithm. Journal of the Royal Statistical Society.
Series B (Methodological), pp.1-38.
Donnan, G. A., Fisher, M., Macleod M., Davis, S.M.,
2008, Stroke, The Lancet 371 (9624).
Dyken, M. L., 1991. Stroke risk factors. In Prevention of
stroke, pp. 83-101. Springer New York.
Fraley, C. and Raftery, A. E., 2006. MCLUST version 3:
an R package for normal mixture modeling and
model-based clustering. Washington Univ Seattle Dept
of Statistics.
Haldar, P., Pavord, I. D., Shaw, D. E., Berry, M. A.,
Thomas, M., Brightling, C. E., Wardlaw, A. J. and
Green, R. H., 2008. Cluster analysis and clinical
asthma phenotypes. American journal of respiratory
and critical care medicine, 178(3), pp.218-224.
Hirano, S., Sun, X. and Tsumoto, S., 2004. Comparison of
clustering methods for clinical databases. Information
Sciences, 159(3), pp.155-165.
Jain, A. K., 2010. Data clustering: 50 years beyond K-
means. Pattern Recognition Letters,
31(8), pp.651-
666.
Jain, A. K. and Maheswari, S., 2012. Survey of recent
clustering techniques in data mining. Int. J. Comput.
Sci. Manage. Res, 1, pp.72-78.
Kruskal, W. H. and Wallis, W. A., 1952. Use of ranks in
one-criterion variance analysis. Journal of the
American statistical Association, 47(260), pp.583-621.
MacQueen, J., 1967. Some methods for classification and
analysis of multivariate observations. In Proceedings
of the fifth Berkeley symposium on mathematical
statistics and probability (Vol. 1, No. 14, pp. 281-
297).
Moore, W. C., Meyers, D. A., Wenzel, S. E., Teague, W.
G., Li, H., Li, X., ... and Bleecker, E. R., 2010.
Identification of asthma phenotypes using cluster
analysis in the Severe Asthma Research
Program. American journal of respiratory and critical
care medicine, 181(4), pp.315-323.
Mulroy, S., Gronley, J., Weiss, W., Newsam, C. and
Perry, J., 2003. Use of cluster analysis for gait pattern
classification of patients in the early and late recovery
phases following stroke. Gait & posture, 18(1),
pp.114-125.
Neal, R. M. and Hinton, G. E., 1998. A view of the EM
algorithm that justifies incremental, sparse, and other
variants. Learning in graphical models. Springer
Netherlands, pp.355-368.
Omar, W. R. W., Taib, M. N., Jailani, R., Fuad, N., Isa, R.
M., Jahidin, A. H. and Sharif, Z., 2013. Acute
Ischemic Stroke Brainwave Classification Using
Relative Power Ratio Cluster Analysis. Procedia-
Social and Behavioral Sciences, 97, pp.546-552.
Pang-Ning, T., Steinbach, M. and Kumar, V., 2005.
Introduction to data mining. Addison-Wesley. 2
nd
edition.
Quinlan, J. R., 1996, Improved use of continuous
attributes in C4.5. Journal of Artificial Intelligence
Research, 4:77-90.
Rankin, J., 1957. Cerebral vascular accidents in patients
over the age of 60. II. Prognosis. Scottish medical
journal, 2(5), pp.200-215.
Schwarz, G., 1978. Estimating the dimension of a model.
The annals of statistics, 6(2), pp.461-464.
Shapiro, S.S. and Wilk, M.B., 1965. An analysis of
variance test for normality (complete samples).
Biometrika, pp.591-611.
Witten, I. H., Frank, E. and Hall, M. A., 2011. Data
Mining: Practical Machine Learning Tools and
Techniques. Morgan Kaufmann. 3
rd
edition.
Model-basedClusteringofIschemicStrokePatients
181