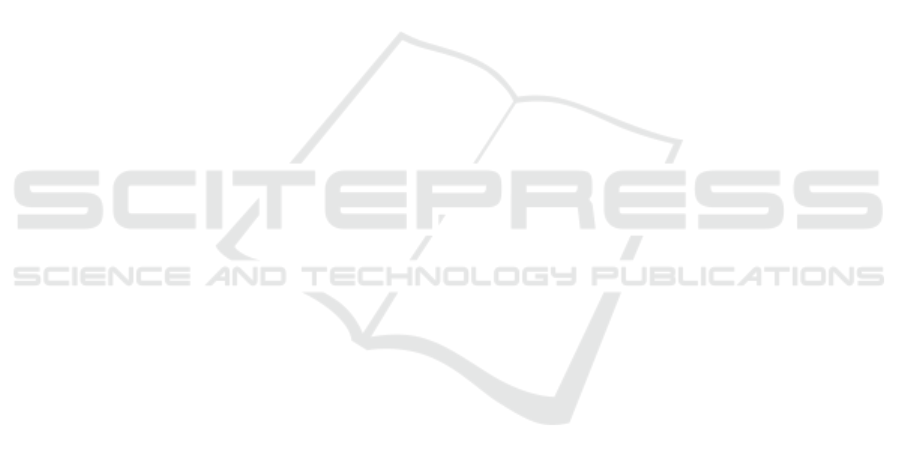
cadence rate are increased. Freezing of gait (FOG) is
typically a transient episode, lasting less than a
minute, in which gait is halted and the patient
complains that feet are glued to the ground. FOG
can be experienced in narrow or tight quarters such
as a doorway or in the presence of an obstacle along
the path and in stressful situations such as when the
telephone or the doorbell rings or when the elevator
door opens. During FOG, PD patients undergo trunk
fluctuations back and forth, move hazardously the
body mass center and load in the forefoot regions,
sometimes resulting in stability loss. At an advanced
stage of the disease, FOG leads to falls in many
instances, in fact, about the 45% of falls of PD
patients occurs forward, due to trunk fluctuations
back and forth. Very recently, some authors have
proposed a IMU based-system which gives an alarm
feedback to assistants or relatives in the case of
patient’s fall (Cabestany, 2013). There are some
evidences that audio stimulations may help the
patient’s to reduce the tendency to undergo FOG.
Auditory stimulations are commonly rhythmic cues,
sometimes embedded in music, set at or slightly
above the patient’s usual cadence. An IMU based-
system has been recently proposed, which gives an
audio feedback to the patient in the case of FOG, to
help the subject to overcome the involuntary block
and prevent the risk of falls (Cabestany, 2013; Sama,
2013; Rodríguez-Martín, 2013). It is evident that the
correct identification of the FOG is crucial, since in
this case any misevaluation of the patient behavior
can be deleterious. For all these reasons, first of all it
is of most relevance to monitor FOG events,
unequivocally distinguishing them from any kind of
voluntary movement, quantifying the daily
frequency, identifying the environmental and the
individual conditions which lead that specific patient
to manifest FOG, finding correlations with the drug
administration and, finally, trying to prevent
catastrophic events such as falls. Optimized drug
therapy can be very effective, especially at an early
stage of the disease. However, drug therapy
optimization is difficult since the response of PD
patients to drugs may vary according to a number of
factors. 24 hours monitoring is the only way to
optimize the therapy and prevent worsening of
symptoms or catastrophic accidents (as falls) due to
incomplete clinical analysis of symptoms during the
day and a consequent not-optimized therapy. On the
other hand, hospitalization is really exceptional
today, due to finance cuts imposed by governments
to national health services. The sensing system
proposed here has the final topic of making possible
the long-time monitoring of specific motor
symptoms of PD while the patient is at home. The
system is composed by a network of several
biosensors disseminated on the patient body which
embed units for the direct non-invasive
measurement of the muscles activity (surface
electromyography, S-EMG). It is being used in the
real-time detection and analysis of PD motor
symptoms. The biosensors are wearable and not
invasive, easy to use and do not need any technical
skill from the patient side. Clinical advantage lies in
the optimization and customization of the drug
therapy for each individual patient. Social benefits
lye in a better quality of life of the patient and the
assisting family.
2 THE SYSTEM
The sensors network system presented in this paper
is designed for both collecting movement signals
and preliminarily analysing them in real-time. This
system is a flexible platform useful for collecting
data via a triaxial accelerometer, a gyroscope and a
magnetometer, with the possibility to incorporate
other information sources in real-time, as the S-
EMG which detects the muscle activity. The Flash
memory stores all inertial data and a Bluetooth
module sends information to other external devices.
The system allows pattern reconstruction of the
kinetic components of movements, discriminates
between voluntary and involuntary movements,
selects only those associated to specific PD
symptoms, reconstructs and, finally, quantifies their
amplitude and frequency. The great challenge of this
work is the automatic association of electronic
signals to specific PD symptoms, filtering all the
signals deriving from voluntary movements. In this
work engineers and neurologists are involved
contemporarily. The engineers develop the
hardware/software system, while the doctors carry
on the clinical research. Patients are clinically
evaluated and movements classified with standard
protocols and compared with voluntary movements
of healthy subjects. This step allows identifying
specific patterns correlated to PD symptoms and is
fundamental for the system calibration. On their
side, engineers optimize the hardware (type of
inertial sensors, protocol of the wireless
communication, entity of the data storage, power
consumption, battery, integration of the EMG, other)
and develop algorithms for data acquisition and
processing. Electrical signals from biosensors are
compared with clinical observations, in order to
achieve the automatic recognition of the disordered
Wearable Wireless Inertial Sensors for Long-Time Monitoring of Specific Motor Symptoms in Parkinsonâ
˘
A
´
Zs Disease
169