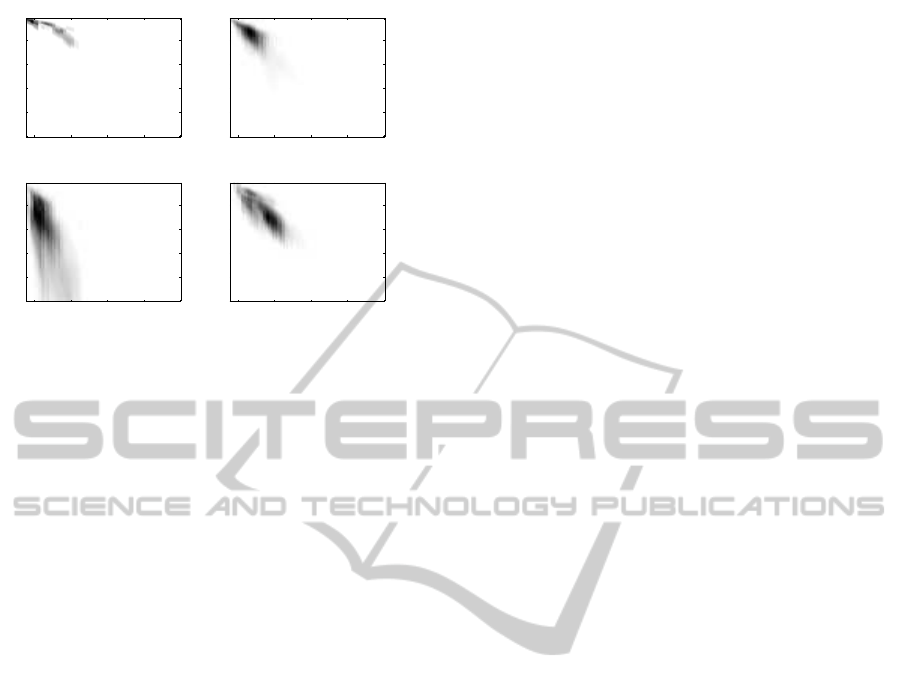
REST
EXCURSION [mm]
100 200 300 400 500
1
2
3
4
5
SHIVERING
100 200 300 400 500
1
2
3
4
5
STRESS
TIME [ms]
EXCURSION [mm]
100 200 300 400 500
1
2
3
4
5
RECOVERY
TIME [ms]
100 200 300 400 500
1
2
3
4
5
Figure 4: Average score values for the data from one subject
(black color - score 1.0).
leads to a data rate of 10 prototype indices per sec-
ond. The training and cross-validation of the HMM
were performed in the same way as in Section 5. The
model had 12 hidden states and the model for tested
for each 4 second episode.
The accumulated results of multiple cross-
validations show that the sensitivity and specificity of
the HMM model in the four-class classification prob-
lem are 75% and 75%, respectively, and the largest
errors are again in the correct classification of the re-
covery phase.
7 DISCUSSION
The topic of the paper is automatic classification of
physiological tremor, shivering, and tremors caused
by physical stress. Three different experiments were
reported. First, it was demonstrated that the ac-
celerometer data can be modeled as a low-order time-
varying autoregressive process and that there are dif-
ferences between the data types in the prediction gain
values. Next, the experiment with a naive Bayes clas-
sifier showed that the different data types can be clas-
sified based on long-term statistics. Finally, similar
classification performance was obtained by modeling
movements as a Markov process of small prototypic
movements.
All modeling approaches seem motivated and are
effective but the error rates were relatively high in all
classification experiments, in particular, in the recov-
ery and resting data.
REFERENCES
Ackmann, J. J., Sances, A., Larson, S. J., and Baker, J. B.
(1977). Quantitative Evaluation of Long-Term Parkin-
son Tremor. Biomedical Engineering, IEEE Transac-
tions on, BME-24(1):49–56.
Akamatsu, N., Hannaford, B., and Stark, L. (1988). An
intrinsic mechanism for the oscillatory contraction
of muscle. In Engineering in Medicine and Biol-
ogy Society, 1988. Proceedings of the Annual Inter-
national Conference of the IEEE, pages 1730–1731
vol.4. IEEE.
Becker, B. C., Tummala, H., and Riviere, C. N. (2008). Au-
toregressive modeling of physiological tremor under
microsurgical conditions. In Engineering in Medicine
and Biology Society, 2008. EMBS 2008. 30th Annual
International Conference of the IEEE, pages 1948–
1951. IEEE.
Ebenbichler, G. R., Kollmitzer, J., Erim, Z., L
¨
oscher,
W. N., Kerschan, K., Posch, M., Nowotny, T., Kranzl,
A., W
¨
ober, C., and Bochdansky, T. (2000). Load-
dependence of fatigue related changes in tremor
around 10 Hz. Clinical neurophysiology, 111(1):106–
111.
Flash, T. and Hogan, N. (1985). The coordination of arm
movements: an experimentally confirmed mathemati-
cal model. The Journal of Neuroscience, 5(7):1688–
1703.
Gantert, C., Honerkamp, J., and Timmer, J. (1992). Ana-
lyzing the dynamics of hand tremor time series. Bio-
logical Cybernetics, 66:479–484.
Grenier, Y. (1983). Time-dependent ARMA modeling of
nonstationary signals. Acoustics, Speech and Signal
Processing, IEEE Transactions on, 31(4):899–911.
Jakubowski, J., Kwiatos, K., Chwaleba, A., and Osowski, S.
(2002). Higher order statistics and neural network for
tremor recognition. IEEE transactions on bio-medical
engineering, 49(2):152–159.
Kucukelbir, A., Kushki, A., and Plataniotis, K. N. (2009). A
new stochastic estimator for tremor frequency track-
ing. In Acoustics, Speech and Signal Processing,
2009. ICASSP 2009. IEEE International Conference
on, pages 421–424. IEEE.
Morrison, S. F. and Nakamura, K. (2011). Central neu-
ral pathways for thermoregulation. Frontiers in bio-
science : a journal and virtual library, 16:74–104.
Murphy, K. P. (2012). Machine Learning: A Probabilis-
tic Perspective (Adaptive Computation and Machine
Learning series). The MIT Press.
Palmes, P., Ang, W. T., Widjaja, F., Tan, L. C. S., and Au,
W. L. (2010). Pattern Mining of Multichannel sEMG
for Tremor Classification. Biomedical Engineering,
IEEE Transactions on, 57(12):2795–2805.
Sung, M., DeVaul, R., Jimenez, S., Gips, J., and Pentland,
A. S. (2004). Shiver Motion and Core Body Temper-
ature Classification for Wearable Soldier Health Mon-
itoring Systems. In Proceedings of the Eighth Inter-
national Symposium on Wearable Computers, ISWC
’04, pages 192–193, Washington, DC, USA. IEEE
Computer Society.
BIOSIGNALS2015-InternationalConferenceonBio-inspiredSystemsandSignalProcessing
316