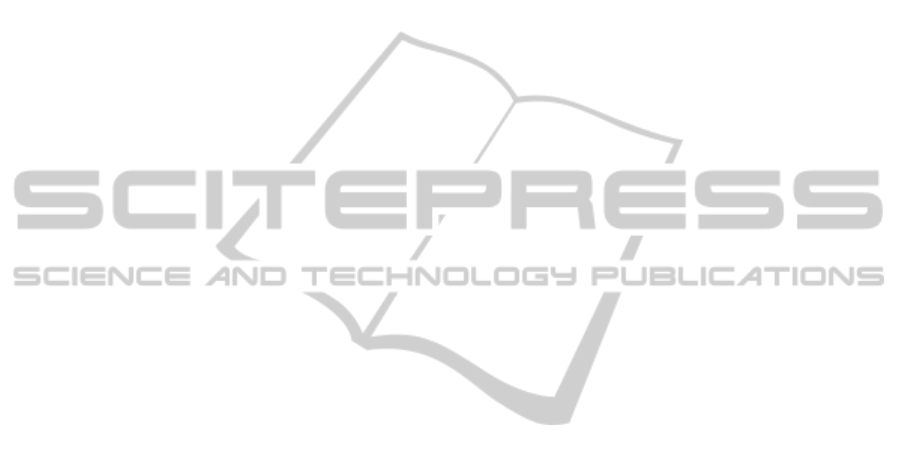
4.3 Agent Properties
In table 1 we summarize these agents’ main inputs,
their main internal processes, their actions, and some
desired properties. We characterize each type of
agents in terms of what are its inputs, its main inter-
nal processes, the actions they can perform, and some
agent related properties.
4.4 Agent Interaction
Cooperation among CST agents happens through
their direct interaction with the CCT agent, that coor-
dinates the tasks and receives the results. Interaction
amongst other agents (CCT, AST, DNL, NRL) has the
role to trigger in each of these agents the goal to per-
form the task under its responsibility. In other words,
all agents have their own specific expertise, and they
have independent and not conflicting goals. So, in
this model there isn’t the conflicting goals problem or
the need for negotiation among agents. On the other
hand, there is plenty of cooperation for the achieve-
ment of a common goal.
CST and AST agents learn and evolve to reduce
the false positive problem, common to systems based
only on a set of rules and patterns of behavior (Gao
et al., 2006; Le Khac and Kechadi, 2010).
5 CONCLUSIONS
The main goal of this contribution is to present a novel
approach to money laundering detection and preven-
tion. This is an ongoing research that has the ultimate
goal of contributing to AML process improvement in
a concrete organization.
It is consensual that the task is hard and far from
being solved, which establishes the relevance of this
work.
As mentioned in section 3, other authors have ex-
plored agent-based approaches to this problem. Nev-
ertheless, our work is distinguished from those, for
start, in the architecture we defined, namely on the
explicit integration of learning components, and in the
inclusion of product specific agents.
There are two main paths in this project. One re-
lates to the definition and implementation of the mul-
tiagent system that will be the basis of the new deci-
sion making process. The other relates to the learning
of new rules and parameters that will serve as valu-
able resources for the agents defined. This second part
relies heavily on real data concerning transactions in
financial institutions.
There is a lot of work still to be done in both paths.
We present the most relevant for now in the following
section.
5.1 Future Work
Regarding agent models, they still need to be refined.
Regarding CST agents, the first product to be included
is the “current accounts”, which is currently being
done. Then we will proceed to other products.
In another trend, we are also working on behav-
ior modeling (recall that suspicious behavior cannot
be found by looking at isolated operations). We are
building behavior patterns that consider large time
spans. This will allow the decision process of the
CCT agent.
Regarding NRL agents, apart from the identifica-
tion of product specific properties/rules, we’re trying
to find cross-product relations, that is, finding, for
each client, in what way are suspicious transactions
regarding a product related to transaction patterns in
the other products. The ultimate goal is to obtain new
parameters to be used by CTS agents.
REFERENCES
Demazeau, Y. (1995). From interactions to collective be-
haviour in agent-based systems. In Proceedings of
the 1st. European Conference on Cognitive Science.
Saint-Malo, pages 117–132.
Gao, S. and Xu, D. (2010). Real-time exception manage-
ment decision model (RTEMDM): Applications in in-
telligent agent-assisted decision support in logistics
and anti-money laundering domains. In System Sci-
ences (HICSS), 2010 43rd Hawaii International Con-
ference on, pages 1–10.
Gao, S., Xu, D., Wang, H., and Wang, Y. (2006). Intelligent
anti-money laundering system. In Service Operations
and Logistics, and Informatics, 2006. SOLI ’06. IEEE
International Conference on, pages 851–856.
Kingdon, J. (2004). AI fights money laundering. Intelligent
Systems, IEEE, 19(3):87–89.
Le Khac, N. A. and Kechadi, M.-T. (2010). Application
of data mining for anti-money laundering detection:
A case study. In Proceedings of the 2010 IEEE In-
ternational Conference on Data Mining Workshops,
ICDMW ’10, pages 577–584, Washington, DC, USA.
IEEE Computer Society.
Liu, X. and Zhang, P. (2010). A scan statistics based sus-
picious transactions detection model for anti-money
laundering (aml) in financial institutions. In Multime-
dia Communications (Mediacom), 2010 International
Conference on, pages 210–213.
Tang, J. and Yin, J. (2005). Developing an intelligent
data discriminating system of anti-money laundering
based on svm. In Machine Learning and Cybernetics,
ICAART2015-InternationalConferenceonAgentsandArtificialIntelligence
234