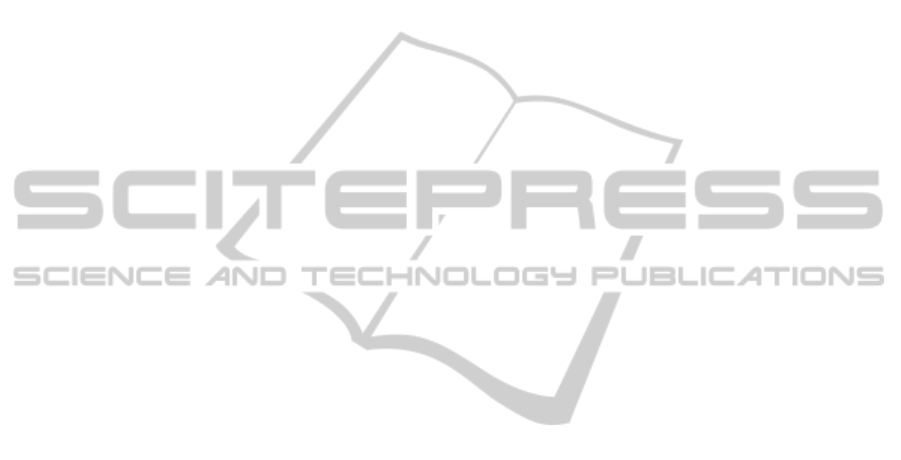
of a dataset to induce emotions and dispositions via
specific and carefully selected stimuli and in the sec-
ond task to focus on the ability of the system to detect
this emotion/disposition and to regulate the interac-
tion back to a positive state. This closed-loop exper-
imental design would allow for the classification to
detect the emotional state and afterwards to be able to
influence the ongoing interaction with the means of
positive feedback, help or supporting stimuli. How-
ever, a self rating should be included (see above) to
have a manipulation check.
5 CONCLUSION
In this position paper, the common design process
for affective data collections was reviewed and ideas
were developed to categorize the necessary steps from
choice of subjects over design of stimuli to the an-
notation of the material. In this work, we propose
to shift the majority of work in the creative process
from corpus design and recording towards annota-
tion to achieve even more natural corpora. This goal
is achieved by leveraging the intrinsic motivation of
subjects in performing specific tasks that occur natu-
rally or by design based on the subject group and then
use human raters to annotate the engaging parts of the
recording using hybrid labeling. Future implementa-
tions of the proposed paradigms should validate the
feasibility of this approach.
ACKNOWLEDGEMENTS
This paper is based on work done within the Tran-
sregional Collaborative Research Centre SFB/TRR
62 Companion-Technology for Cognitive Technical
Systems funded by the German Research Founda-
tion (DFG). Markus K
¨
achele is supported by a schol-
arship of the Landesgraduiertenf
¨
orderung Baden-
W
¨
urttemberg at Ulm University.
REFERENCES
Burkhardt, F., Paeschke, A., Rolfes, M., Sendlmeier, W. F.,
and Weiss, B. (2005). A database of german emotional
speech. In INTERSPEECH’05, pages 1517–1520.
Codispoti, M., Surcinelli, P., and Baldaro, B. (2008).
Watching emotional movies: Affective reactions and
gender differences. International Journal of Psy-
chophysiology, 69(2):90–95.
Daly, I., Malik, A., Hwang, F., Roesch, E., Weaver, J.,
Kirke, A., Williams, D., Miranda, E., and Nasuto,
S. J. (2014). Neural correlates of emotional responses
to music: An EEG study. Neuroscience Letters,
573(0):52–57.
Ekman, P., Sorenson, E. R., and Friesen, W. V. (1969). Pan-
cultural elements in facial displays of emotion. Sci-
ence, 164(3875):86–88.
Glodek, M., Reuter, S., Schels, M., Dietmayer, K., and
Schwenker, F. (2013). Kalman filter based classifier
fusion for affective state recognition. In MCS, volume
7872 of LNCS, pages 85–94.
Hamann, S. (2012). Mapping discrete and dimensional
emotions onto the brain: controversies and consensus.
Trends in Cognitive Sciences, 16(9):458–466.
Healey, J. A. (2000). Wearable and automotive systems for
affect recognition from physiology. PhD thesis, MIT.
Hewig, J., Hagemann, D., Seifert, J., Gollwitzer, M., Nau-
mann, E., and Bartussek, D. (2005). A revised film
set for the induction of basic emotions. Cognition &
Emotion, 19(7):1095–1109.
K
¨
achele, M., Glodek, M., Zharkov, D., Meudt, S., and
Schwenker, F. (2014). Fusion of audio-visual features
using hierarchical classifier systems for the recogni-
tion of affective states and the state of depression. In
Proceedings of ICPRAM, pages 671–678. SciTePress.
K
¨
achele, M., Schels, M., and Schwenker, F. (2014). Infer-
ring depression and affect from application dependent
meta knowledge. In Proceedings of the 4th Interna-
tional Workshop on Audio/Visual Emotion Challenge,
pages 41–48. ACM. Best entry for the Affect Recog-
nition Sub-Challenge.
Kanade, T., Cohn, J., and Tian, Y. (2000). Comprehensive
database for facial expression analysis. In Automatic
Face and Gesture Recognition, 2000., pages 46–53.
Kreibig, S. D., Wilhelm, F. H., Roth, W. T., and Gross, J. J.
(2007). Cardiovascular, electrodermal, and respiratory
response patterns to fear and sadness inducing films.
Psychophysiology, 44(5):787–806.
Labouvie-Vief, G., Lumley, M. A., Jain, E., and Heinze, H.
(2003). Age and gender differences in cardiac reac-
tivity and subjective emotion responses to emotional
autobiographical memories. Emotion, 3(2):115–126.
Lang, P. J., Bradley, M. M., and Cuthbert, B. N. (2005).
International affective picture system (IAPS): Affec-
tive ratings of pictures and instruction manual. Tech-
nical Report A-6, University of Florida, Gainesville,
FL, Gainesville, FL.
Lindquist, K. a., Siegel, E. H., Quigley, K. S., and Bar-
rett, L. F. (2013). The hundred-year emotion war:
are emotions natural kinds or psychological construc-
tions? comment on Lench, Flores, and Bench (2011).
Psychological bulletin, 139(1):255–63.
Nater, U. M., Abbruzzese, E., Krebs, M., and Ehlert, U.
(2006). Sex differences in emotional and psychophys-
iological responses to musical stimuli. Int J Psy-
chophysiol, 62(2):300–308.
Palm, G. and Glodek, M. (2013). Towards emotion recog-
nition in human computer interaction. In Neural Nets
and Surroundings, volume 19 of Smart Innovation,
Systems and Technologies, pages 323–336.
R
¨
osner, D., Frommer, J., Friesen, R., Haase, M., Lange, J.,
and Otto, M. (2012). LAST MINUTE: a multimodal
ICPRAM2015-InternationalConferenceonPatternRecognitionApplicationsandMethods
372